6. Assessing the Transferability of Economic Evaluations: A Decision Framework
© Chapter’s authors, CC BY 4.0 https://doi.org/10.11647/OBP.0195.06
6.1 Introduction
As the field of economic evaluation has grown, questions have arisen about how ‘transferable’ or ‘generalizable’ studies are across settings. Part of the answer may lie in improving standards for economic evaluation. Various organizations and groups have proposed standard practices (‘reference case analyses’) to ensure transparency, high quality and comparability across cost-effectiveness analyses.1 Over the past decade, many high-income countries have also developed their own standards and guidelines.2 On the other hand, despite increasing use of economic evaluations for priority-setting and reimbursement decisions,3 local guidelines have only recently emerged among low- and middle-income countries (LMICs).4 To fill the gap, the international Decision Support Initiative (iDSI), with the support of the Bill and Melinda Gates Foundation, has provided a ‘reference case’ for economic evaluations to reflect best practices and guidelines that could apply to different contexts, particularly in LMICs.5
Still, questions about transferability remain. In recent years, the number of available CEAs employing disability-adjusted life-years (DALYs) has grown rapidly (Fig. 6.1). These analyses typically reflect the context and health systems of a particular country or region. Ideally, local authorities in other areas, seeking to understand their own locally relevant Best or Wasted Buys and lacking an economic evaluation applied to their own jurisdictions, would conduct a new study to generate localized evidence. However, such authorities, particular in LMICs, often lack expertise and resources for producing such evidence.6 As shown in Figure 6.2,7 many LMICs have few or no economic studies available, thus highlighting the opportunity for decision-makers in these jurisdictions to apply economic evaluations conducted elsewhere.
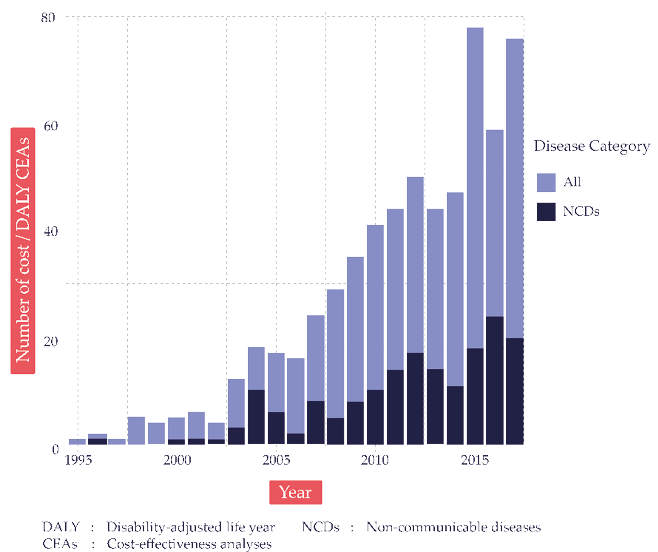
Fig. 6.1 The growth of cost-per-DALY-averted studies. Source: Author’s analysis of Tufts Medical Center Global Health Cost-Effectiveness Analysis (CEA) Registry (www.ghcearegistry.org).
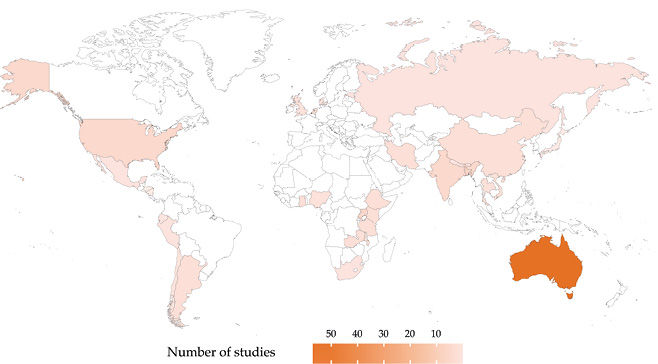
Fig. 6.2 Geographic distributions of available cost-per-DALY-averted studies for non-communicable diseases. Source: Author’s analysis of Tufts Medical Center Global Health CEA Registry (www.ghcearegistry.org).
Note: DALY = disability-adjusted life-year.
When applying economic evidence generated elsewhere to local settings, researchers and decision-makers need to consider potentially important differences in factors such as population characteristics, epidemiology, relative prices, religion and culture, and health systems. For example, the economic value of implementing national breast cancer screening may vary substantially by the regional or local population risks of developing breast cancer; the feasibility of workplace health and safety measures will depend upon the work patterns that prevail and the risks to which workers are exposed; the costs and benefits associated with investing or disinvesting in fertility services or clinics for pregnancy terminations are likely to vary greatly according to predominant national religious affiliations.
For this chapter, we define ‘transferability’ as the extent to which particular study findings can be applied to another setting or context. Results from highly transferable studies could thus be used in various decision-making contexts without further adjustment.
Despite the importance of transferability in global health priority-setting, several major guidelines and reports, including the Disease Control Priorities Third Edition, do not explicitly address a process for evaluating transferability or list the factors to consider for local relevance.8 This chapter provides a decision-making framework and a checklist for the field, to help local decision-makers and practitioners who wish to apply existing economic evaluation results to their own settings.
The chapter starts by reviewing the existing literature on the transferability of economic evaluations (Section 6.2). We then summarize critical factors for consideration and provide a decision-making framework to help determine whether local decision-makers should accept the external evidence without further adjustment, modify it to reflect local data, or reject it altogether (Section 6.3). Section 6.4 provides a worked example to provide a step-by-step illustration of how to perform the transferability assessment using our framework. We also discuss the use of an ‘Impact Inventory’ to aid decision-makers who wish to conduct for themselves original economic evaluations and identify Best and Wasted Buys in local settings (Section 6.5). The final section (Section 6.6) provides conclusions and future steps.
6.2 Review of the Literature
A growing literature discusses issues surrounding the transferability of economic evaluations. Previous studies identified factors such as epidemiology, demography, relative prices, capacities of health systems, political and cultural conditions, affordability and others. These studies also suggested that the transferability of results to other settings was sometimes feasible, though a lack of transparency in the original research often made a judgment impossible. Several case studies have also been conducted to assess transferability empirically, for example, in physical activities among children, breast cancer treatment and smoking cessation.9 Despite the substantial growth of studies on this topic, systematic literature reviews and national guidelines have highlighted variations in approaches regarding the transferability of data from one setting to another.10 Here, we briefly summarize the contributions of major papers identified through Google Scholar, PubMed and cited references. Online Appendix 6A provides the search strategy in detail.
In 1992, Drummond and co-authors first highlighted important considerations for extrapolating economic evaluation results using a case study of a multi-country evaluation of the prophylactic use of misoprostol vs. no prophylaxis for patients with abdominal pain.11 The paper suggested that a standard methodology used for all studies and the application of local data may facilitate the extrapolation process. In 1997, O’Brien summarized concerns about the transferability of cost-effectiveness data, underscoring six significant issues: demographics and the epidemiology of diseases; local clinical practice and conventions; incentives and regulations for providers; relative prices; patient preferences; and the opportunity costs of resources.12
Since the publication of these papers, several other investigators have suggested overarching frameworks, further explored key factors, and have begun to provide an empirical basis for understanding transferability. For example, Sculpher et al. systematically reviewed factors underlying variability in economic evaluations and recommended strategies for improving the generalizability of results.13 Welte et al. developed a transferability decision chart that included ‘knock-out’ criteria and offered a transferability factor checklist as well as methods for improving transferability.14 Boulenger et al. provided the European Network of Health Economics Evaluation Databases (EURONHEED) transferability information checklist.15 Manca and Willan proposed algorithms to choose an appropriate methodology to address between-country differences.16 Goeree et al. identified seventy-seven factors affecting transferability, which they grouped into five categories: the patient; the disease; the provider; the healthcare system; and methodological conventions.17 In an attempt to improve the evaluation process, researchers have developed transferability indices to quantify the degree of transferability of economic evaluations.18 An International Society for Pharmacoeconomics and Outcomes Research (ISPOR) Task Force Report reviewed national guidelines on transferability and made several recommendations for improvement.19 A regional-specific assessment of transferability was conducted for middle-income countries,20 Eastern Europe21 and Latin America.22
Despite previous work to identify key factors and suggest frameworks for assessing transferability, existing tools may not be suited for local authorities due to the technical and complex nature of the assessment. Building upon past literature, we sought to develop a new decision framework and checklist for assessing the transferability of economic evidence to local settings (Section 6.3). Decision-makers and program managers often require rapid answers to complex questions. Our step-by-step guideline is a practical tool to compensate for the scarcity of locally-relevant economic evidence in many LMICs and to help assess the transferability of external evidence.
6.3 A Decision Framework for Identifying Locally-Relevant Best and Wasted Buys
6.3.1 Background
In Chapter 3, the authors describe a decision pyramid (the Systematic thinking for Evidence-based and Efficient Decision-making [SEED] Tool), which suggests exploring the transferability of economic evidence (Consideration 3 of the SEED Tool) as part of a process for identifying Best and Wasted Buys. In this chapter, we provide a decision framework and assessment checklist to assess transferability objectively and transparently in a practical manner.
Before applying the framework and checklist, we recommend that evaluators, like NCD program managers, proceed only after identifying the following types of information: economic evaluations relevant for disease areas of interest (e.g., Best Buy interventions identified in Chapter 4); and local guidelines on economic evaluation to be used as a point of reference during assessment (if unavailable, we recommend international guidelines, such as the iDSI reference case).23 Because assessments can be complex, we suggest convening a technical review panel that involves, if possible, a variety of stakeholders, such as epidemiologists, clinicians, disease program managers and analysts (e.g., decision scientists or modeling experts). A variety of expertise in the review panel can provide diverse perspectives on how best to determine the transferability of the evidence to the local context.
6.3.2 A Decision Framework and a Transferability Assessment Checklist
The process starts with an initial assessment to determine whether the existing study warrants further evaluation (Step 1), followed by a data transferability assessment (Step 2). Using the flowchart (Fig. 6.3), four options regarding transferability exist: 1) applying the external evidence without further adjustment; 2) modifying the economic evidence based on local data; 3) using the evidence with caution when the economic evidence is not necessarily highly transferable, but still deemed informative to the decision problem; and 4) rejecting the evidence altogether. Table 6.1 provides a transferability checklist tool. The case study (Section 6.4 in this chapter) illustrates how to apply our framework and conduct the transferability assessment by using the example of Best Buy interventions for diabetes prevention and management for Kenya.
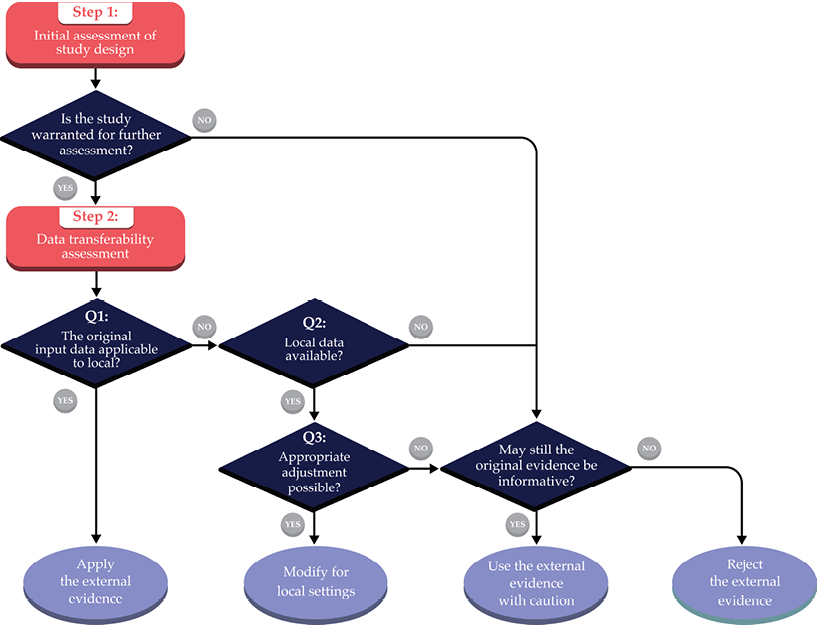
Fig. 6.3 Decision chart for assessing transferability and evidence review.
Table 6.1 Transferability assessment checklist.
Step 1: Initial assessment of study design |
|||||
Criteria |
Evaluation questions for each criterion |
Decision Question: Considering your evaluation for each criterion, is the original study warranted for the further assessment? |
|||
Q1: Is the listed study characteristic aligned with local decision-making context? (If No, go to Q2) |
Q2: Is the original study still informative to the decision problem? |
||||
Study perspective |
|
|
A. No, reject the external evidence B. No, but the external evidence can be used with caution C. Yes, proceed to data transferability assessment (Step 2) |
||
Intervention and its comparator(s) |
|
||||
Time horizon |
|
|
|||
Discounting |
|
|
|||
Study quality |
|
|
|||
Step 2: Data transferability assessment |
|||||
Major considerations |
Evaluation questions for each data input |
Decision Question: Considering your evaluation for each criterion, is the original evidence transferable to your local setting? |
|||
Q1: Are the original input data applied to the local setting? (If No, go to Q2) |
Q2: Is local data on the specific input available? (If Yes, go to Q3 If No, go to Q4) |
Q3: Is appropriate adjustment for local data input possible? (If No, go to Q4) |
Q4: Is the data input used in the original study still informative to the local context? |
||
Baseline risk |
|
|
A. No, reject the external evidence B. No, but the external evidence can be used with caution C. Yes, but only after appropriate adjustments for local data input D. Yes, apply the external evidence as it is |
||
Treatment effects |
|
|
|||
Unit costs/prices |
|
|
|||
Resource utilization |
|
|
|||
Health-state preference weight |
|
|
Step 1: Initial Assessment of Study Design
A foundational starting point is to examine whether the study under consideration is a suitable candidate for the transferability assessment. Previous literature also describes this process as the minimal methodology standard or the set of ‘knock-out’ criteria.24 The initial assessment consists of five components relevant to study design: A) perspective; B) intervention and its comparator(s); C) time horizon; D) discounting; and E) study quality. If any of these components do not meet the minimum criteria — which are subject to the evaluator’s judgment — the study conclusion cannot be applied to local settings. However, when the original study results are judged as potentially useful (e.g., through sensitivity analyses reporting how Incremental Cost-Effectiveness Ratios [ICERs] vary by different perspectives), the evaluator may either apply the original findings with caution or proceed further to the data transferability assessment. We discuss each of the five components for minimum study standards in detail:
A. Study Perspective
Practice guidelines for economic evaluation emphasize the importance of the analytic perspective (or viewpoint) because it determines which costs and benefits to include in the analysis.25 Analytic perspectives may reflect a specific payer (e.g., Ministry of Health or local government), the healthcare sector as a whole, or the broader society. Depending on the choice of perspective, an intervention may be more cost-effective (i.e., have a lower ICER) or less cost-effective. For example, pharmacotherapy for patients with alcohol use disorder is more cost-effective from a societal perspective than a healthcare sector perspective because of improved outcomes that go beyond the healthcare sector, such as improved productivity or reduced alcohol-related motor-vehicle accidents.26 We recommend that evaluators assess whether the study perspective aligns with their own decision-making preferences in their local setting.
B. Intervention and its Comparator(s)
Economic evaluation should reflect the specific decision problem that each individual decision-making group faces (e.g., interventions in routine use in the local setting). As a summary measure, the ICER represents the relative value between an intervention, which might already be available or considered for introduction in the local setting, and a comparator, which could be the standard of care, a comparable intervention, or the absence of an intervention. If the intervention or comparator(s) in the original study are not available or are not relevant in the local settings, results may not be easily transferable. Inadequate description of the intervention and comparator(s) in the original study may also limit transferability.
C. Time Horizon
The time horizon used in CEAs can substantially affect the estimated value of an intervention.27 Standard guidelines recommend using a time horizon long enough to capture all relevant costs and health benefits.28 When assessing interventions targeted for NCDs, such as cardiovascular diseases, cancer and diabetes, a lifetime horizon is recommended. Lifetime horizons can capture all of the important differences in consequences over time. For example, evaluators who wish to understand the economic evidence on cardiovascular disease prevention may want to exclude studies conducted from a short-term horizon.
D. Discounting
Practice guidelines recommend discounting all future costs and health outcomes so that ICERs represent the ‘present value’ of the intervention, adjusting for the differential timing of costs and benefits.29 In other words, discounting makes near-term consequences (e.g., immediate costs and health benefits) more valuable than long-term consequences (e.g., costs and health benefits occurring in distant future). This is because of the opportunity costs to spend money now and to experience immediate benefits instead of those in the future. The use of higher discounting rates (i.e., strongly devaluing distant costs and benefits) tends to underestimate the value of preventive interventions.
A discount rate reflects society’s (or a specific decision-maker’s) time preference (i.e., how much they are willing to trade off consumption today vs. tomorrow). Thus, guidelines sometimes suggest using the real rates of government bonds as a proxy. Despite the common use of 3% or 3.5% for discounting both costs and health outcomes (per guideline recommendations, such as iDSI reference case, designed to promote comparability across studies),30 local evaluators may wish to select a time preference suitable for their country or context, or there may be standard rules set for all public-sector investment decisions.
E. Study Quality
When considering transferability, evaluators may understandably wish to exclude economic evaluations of low quality. The question is how to determine quality. Despite various guidelines and checklists on conducting and reporting CEAs,31 challenges remain because these instruments are not designed to guide decision-makers on how to differentiate high- and low-quality studies. The quality of the study can be assessed based on adherence to the economic evaluation guidelines (the iDSI reference case or the Second Panel’s recommendations) or via a formal quality assessment tool.32 One source for such information is the Tufts Medical Center Global Health CEA Registry (www.ghcearegistry.org), which includes detail on the degree to which published cost-per-DALY-averted studies adhere to the iDSI reference case.33
Step 2: Data Transferability Assessment
After an initial screening, evaluators can determine, depending on data availability, whether the original evidence can be directly applied to their local setting. Despite a long list of items to be considered for data transferability, we focus on five major factors most often referred to in the literature: baseline risk, treatment effects, unit costs/prices, resource utilization and health-state preference weight. We will also briefly describe the other possible items for consideration.34
During the data assessment for each of the five factors, the evaluator will determine whether or not to progress to the next stage by doing a separate analysis in three key aspects. These aspects are: 1) the need for further adjustment; 2) the availability of local data; and 3) the possibility of adjustment based on information from the original study (e.g., in sensitivity analysis) or access to the original model (or authors) for further modification. In certain instances, evaluators may determine that the original study is still informative to the local context even when the local data are not available or appropriate adjustment is not possible.
A. Baseline Risk (Disease Profile)
Variation in underlying population risk factors across countries is linked to different inherent baseline risk characteristics, such as differences in disease incidence, prevalence and background mortality. Differences in baseline risk may influence both an intervention’s effects and its costs in terms of actual resource utilization. For example, implementing a nation-wide screening program for type 2 diabetes may generate more favorable ICERs for countries with a higher prevalence of undiagnosed type 2 diabetes.35 Thus, the evaluator must determine whether the baseline risk in the original study is relevant to the local context.
B. Treatment Effects (Clinical Information)
Treatment effects (i.e., measured as an intervention’s relative efficacy) are generally considered more transferable than other data inputs as the estimate is less likely to depend upon the practices and competencies of local professionals in LMICs and the incentive embodied in the local health system.36 An estimate of the absolute treatment effect from a multinational, randomized controlled trial would presumably have high transferability. An estimate of the relative treatment effect may also be used from country-specific studies after an appropriate adjustment in local baseline risk.
C. Unit Costs/Prices
Adjusting for unit costs or prices relevant to the local context will typically be required for data transferability. Because of its importance,37 economic evaluations often conduct sensitivity analyses on the prices of the intervention/comparator(s) as well as the prices for other services. Assuming that all other data inputs are relevant to the local setting, if the original study provides results from sensitivity analyses for a range of intervention prices, evaluators could extract the ICERs relevant to their local settings without re-analyzing the data. For example, when the price of a drug is $100 in the local setting, instead of $500 in the original study, an ICER from a sensitivity analysis (e.g., $1000/quality-adjusted life-years [QALY] gained at the drug price of $100) can be used as the locally relevant evidence, rather than the original evidence (e.g., $5000/QALY at the drug price of $500).
D. Resource Utilization
Similar to the case for unit costs, the application of locally-relevant resource use data (e.g., on hospital days, physician office visits, or medications) may be required for the estimation of overall costs associated with the intervention and comparator(s). Many international guidelines consider resource use data from external locations as inappropriate sources and strongly encourage the use of locally-relevant resource data.38
E. Health-State Preference Weight
Health-state preference weights, used as inputs into calculations of QALYs, represent the relative desirability for being in different health states. Guidelines generally recommend using generic preference measures (e.g., EQ-5D, SF-6D, or HUI) that assign a specific value to each health state, including zero for dead and one for perfect health.39 Because of social and cultural factors, individuals in different countries may assign different values to similar health states.40 Previous studies have demonstrated that the valuations of health states can be different for US and UK residents and, as a result, cost-effectiveness ratios were doubled when adjusted to US-specific weights.41
For health-related quality of life measures used to calculate QALYs, thirty-two country-specific preference weights for EQ-5D (valuation sets) are currently available and the number continues to grow.42 Disability weights, which are used to calculate DALYs, have been estimated from international survey participants. Although they may not reflect the preference for health states among specific target populations, disability weights may be more readily transferable across different countries.43
Once the data transferability assessment is completed, a final decision is required on whether local decision-makers should: 1) apply the external evidence without further adjustment, 2) modify the evidence based on local data, 3) use the evidence with caution because it is not highly transferable, but still deemed informative, or 4) reject the evidence altogether. In addition to the five major factors listed above, previous literature has described additional factors that may be relevant for assessing transferability.44 The list includes variation in local clinical practice, healthcare infrastructure, cultural background, implementation costs and the valuation of productivity and other non-health benefits. When appropriate, evaluators may include additional factors for their data transferability assessments.
6.4 Worked Example: Assessing Transferability of Best Buy Interventions for Diabetes Prevention and Management in Kenya
6.4.1 Background and Rationale
To provide a step-by-step illustration of how to perform a transferability assessment using our framework and checklist, we offer a worked example from Kenya. We evaluated the transferability of seven studies for diabetes prevention and management,45 which included fourteen interventions deemed Best Buys based on the WHO definition (i.e., cost-saving or ICER ≤ $100 international dollars (I$)/DALY averted). These interventions mostly include screening or interventions targeting behavioral changes (Table 6.2).
This worked example should be viewed as a stylized application, in order to provide an illustrative case study, rather than a definitive analysis for Kenya. Thus, throughout the example, we assume the role of a program manager for a hypothetical national diabetes prevention and control program in Kenya. The primary responsibility of the manager is to determine whether the identified Best Buy interventions for diabetes prevention and management can be transferrable to Kenya and recommended as part of the country’s essential health benefits package.
We selected Kenya as the target country for two reasons: 1) Kenya’s recent move to Universal Health Coverage (UHC);46 and 2) the rising burden of diabetes in the country.47 Kenya’s efforts to reform its health and finance system to achieve UHC have been the subject of media coverage.48 However, barriers remain to achieving UHC in Kenya, specifically for NCD coverage, as infectious disease remains the focus of the government’s funding and coverage expansions.49 The burden of NCDs in Kenya has been rapidly increasing, accounting for 13,200 DALYs [36% of the country’s overall disease burden in 2017, up from 25% in 1990). The prevalence of diabetes in Kenya was 2.4% in 2015,50 and its burden is growing, accounting for 1.7% of total DALYs in 2017, up from 0.83% in 1990.51
6.4.2 Evaluator’s Guideline on Economic Evaluation
To our knowledge, Kenya does not have local guidelines for conducting economic evaluations. For this stylized example, we selected the iDSI reference case as our hypothetical economic evaluation guideline for Kenya for the purpose of the transferability assessment.52 Again, we note that the assumptions should be considered as illustrative and may not reflect actual context or preferences in Kenya.
For the initial assessment of study design (Step 1), our baseline decision making criteria were the following: 1) Study perspective: a societal perspective preferred, but healthcare payer (or government) perspective is acceptable; 2) Intervention and its comparator(s): the intervention under consideration should be available in the local setting; 3) Time horizon: a lifetime horizon is strongly preferred, but results from a shorter time horizon may also be considered with caveats; 4) Discounting: a 3% annual discount rate for both costs and health outcomes is preferred but results using different discounting rates may also be considered with a caveat; and 5) Study quality: poor study quality, which can be assessed based on adherence to the iDSI reference case guidelines,53 is a reason for excluding a study from further assessment.
For the data transferability assessment (Step 2), considering our hypothetical role of a program manager for a national diabetes prevention and control program in Kenya, we assume that local data on baseline risk (i.e., disease profiles), unit costs/prices and resource utilization are readily available. Data on treatment effects or other relevant clinical information (e.g., diabetes risk prediction) are assumed to be transferable to Kenya in the absence of locally-relevant clinical data. Finally, we assume that use of disability weights, or health-related quality of life weights measured from local participants and valued using a local valuation set, is preferred, but measures or valuation sets from elsewhere can be used with a caveat. A summary of the hypothetical economic evaluation guideline for Kenya, on which our assessment is based, is available in the Online Appendix 6B.
Table 6.2 Assessing transferability of Best Buy interventions for diabetes prevention and management.
Study |
Year |
Intervention |
Comparator |
Study countries |
Recommendations for transferability of external evidence to Kenya settings (Consensus statement) |
Goldman |
2006 |
Diabetes control (stated as: ‘prevention,’ and ‘better treatment’) |
None |
United States |
The study finding is not transferable due to the failure to meet the minimum criteria for the study design evaluation. (e.g., no specific intervention was evaluated, no discounting rate was stated and low-quality score) |
Colagiuri |
2008 |
Screening for undiagnosed diabetes or pre-diabetes |
None |
Australia |
Due to differences in key data inputs and the inability to adjust the original findings, the study finding is not directly transferable. However, the external evidence may be used with caution when reflecting local-level data (e.g., utilization rates). |
Bertram |
2010 |
Multiple interventions, 6 total:(1) pre-diabetes screening and diet and exercise; (2) pre-diabetes screening and exercise; (3) pre-diabetes screening and diet; (4) pre-diabetes screening and Acarbose; (5) pre-diabetes screening and Metformin; (6) pre-diabetes screening and Orlistat |
Standard/usual care |
Australia |
Due to differences in key input data (e.g., Australian-specific disability weights were used), the study finding is not directly transferable. However, the external evidence may be used to (A) inform future research on the original cost-effectiveness study, or (B) pilot on a small scale to test for feasibility. |
Lohse |
2011 |
Gestational diabetes mellitus screening and lifestyle change |
None |
India; Israel |
Given limited data on gestational diabetes and antenatal resource utilization, the study finding is not directly transferable. However, the study findings of cost-savings or favourable cost-effectiveness, which are applicable to a wide range of input values in India and Israel, may be used to inform resource allocation decisions in Kenya with caution. (India has a similar GDP per capita as Kenya) |
Salomon |
2012 |
Multiple interventions, 4 total: (1) lipid control; (2) blood pressure control; (3) conventional glycemic control; and (4) intensive glycemic control |
None |
Mexico |
Reviewers both agreed that this is a high-quality study with great detail provided for key data inputs. With acknowledging that the study is based on the Mexican setting, the study findings could be used in Kenya settings after making reasonable substitutions based on local data, such as local prices. |
Marseille |
2013 |
Gestational diabetes mellitus screening using oral glucose tolerance test |
None |
India; Israel |
Same recommendations as we made for Lohse et al., 2011. This study is an update of the existing study and reported similar results. |
Basu |
2016 |
Benefit-based tailored treatment (BTT)(which is based on a composite risk score of cardiovascular diseases or microvascular disease) |
treat-to-target (TTT) strategy, which is based on achieving target levels of specific biomarkers (e.g., cholesterol level) |
China; Ghana; India; Mexico; South Africa |
Considering similarities between one of the study countries, Ghana and Kenya in terms of disease prevalence and economic profile, the external evidence is highly transferable and can be applied to inform resource allocation decisions in Kenya settings. |
6.4.3 Transferability Assessment Process
We conducted a transferability assessment as follows. Three evaluators on our research team with experience in cost-effectiveness analysis (a senior investigator and two junior researchers) formed our ‘evaluation committee’ to simulate the kind of transferability assessment that might occur in Kenya. The evaluation consisted of first reviewing: 1) the decision chart for assessing transferability (Fig. 6.3); 2) the transferability assessment checklist (Table 6.1); and 3) the hypothetical economic evaluation guideline for Kenya (Online Appendix 6B). After an initial training session, each of the three committee members independently conducted a transferability assessment for one of the seven articles54 and then convened to review and discuss questions or challenges that arose during the assessment.
Once the two junior evaluators completed the basic training, they were ‘commissioned’ to evaluate the transferability of the remaining six articles independently. Next, they convened a consensus meeting to discuss whether the original study warranted further data assessment and, if so, whether the original evidence could be transferable to Kenya. Although each of the evaluators was encouraged to assess specific questions pertaining to the individual study characteristics and data inputs listed in Table 6.1, the final decision corresponded to one of four options: 1) apply the external evidence without further adjustment; 2) modify the evidence based on local data; 3) use the evidence with caution because the economic evidence was not necessarily highly transferable; and 4) reject the evidence altogether. During the consensus meeting, each of the members shared their individual decision and comments and the group discussed conflicting opinions to reach a consensus. Finally, the group made consensus recommendations for the transferability of the external evidence.
6.4.4 Transferability Assessment Results
Among seven studies evaluated, only one was deemed directly transferable to Kenya. In that case, the country of the original study, Ghana, was deemed sufficiently similar to Kenya in terms of disease prevalence and its economic profile.55 The study found that a benefit-based tailored treatment, a strategy to reduce the composite risk of developing CVD in the next ten years or a microvascular disease risk over a lifetime for patients with type 2 diabetes, was a cost-saving strategy (i.e., lower costs with greater health benefits), compared to a treat-to-target strategy, which aimed to achieve target levels of specific biomarkers.
Our committee also decided that another study was not transferable due to its failure to meet the minimum criteria for the study design evaluation.56 For example, although the original study reported that effective control of hypertension could avoid 75 million DALYs and reduce healthcare spending by $890 billion, the study did not examine any specific intervention to achieve effective hypertension control. The study’s low-quality score (it neither stated its discount rate nor listed a specific intervention to be targeted) contributed to the committee’s decision to reject the use of this evidence for Kenya.
For most of the other cases, study findings were deemed not directly transferable due to differences in key data inputs and an inability to adjust the original findings. However, the committee believed that the external evidence may still provide useful insight for how resources for diabetes prevention and management might best be allocated for Kenya, though caveats and caution were in order.
The initial assessment of the study design (Step 1) reached consensus with no disagreement. However, evaluation committee members were often unsure about the data transferability assessment (Step 2). Some of the assessment questions required knowledge about the availability of local data inputs and the accessibility of the models, which was not readily grasped by the committee members. During the consensus meeting, the evaluation committee resolved conflicts and ambiguity based on our guideline of transferability assessment designed for Kenya (the Online Appendix 6B). Table 6.2 provides our committee’s consensus recommendations for the seven studies. The Online Appendix 6C provides the individual transferability assessment forms completed by the two evaluators for all of the studies.
Our worked example revealed a few challenges in assessing transferability. First, the lack of transparency in the reporting of existing economic evaluations, particularly on data inputs (e.g., unit costs/prices), often constrained the ability to determine transferability. The use of the online appendix to provide analytic approaches, and to model assumptions and data inputs in detail, would be valuable. More comprehensive deterministic and probabilistic sensitivity and scenario analyses in published economic evaluations may also help to improve transferability of the external evidence.
Another issue was the inaccessibility of the original models needed to generate results with locally-relevant data inputs. In practice, evaluators may reach out to study author(s) to obtain the original model. Access to an original model allows evaluators to revise the analysis, reflecting local data and adapting the model structure or assumptions to be more context-specific. The open-source model would thus be valuable in LMIC settings.57
Finally, when assessing interventions and their comparator(s), the feasibility and scalability for Kenya frequently arose as a concern. For example, although many interventions involving diet and exercise counseling are found to be cost-saving or very cost-effective for managing diabetes,58 it was challenging to assess the availability, feasibility and scalability of such interventions in Kenya without input from a local expert. In actual practice, a diverse set of experts in the evaluation committee, such as epidemiologists, clinicians, disease-program managers and analysts, may help to alleviate some of these concerns.
6.5 Using the Impact Inventory
In previous sections, we sought to provide a framework for decision-makers and practitioners to assess the transferability of economic evaluation to local settings. When possible, analysts should conduct original economic evaluations to identify relevant Best and Wasted Buys in local settings. For these cases, we recommend using an ‘Impact Inventory’, a structured table listing an intervention’s health and non-health consequences, developed by the Second Panel on Cost-Effectiveness in Health and Medicine.59 The Impact Inventory is intended to ensure that all the consequences of interventions, including those falling outside the formal healthcare sector, are considered regularly and comprehensively (Online Appendix 6D).
Because of the substantial impact of NCDs on non-healthcare sectors, it is essential to consider the potential consequences of interventions for NCDs as much as possible. Ideally, analyses will consider factors, such as health effects on caregivers among Alzheimers patients or the impact of some interventions (e.g., alcohol-use-disorder treatment) on the criminal justice system. Even if decision-makers disagree over how to value those consequences and do not incorporate them into formal assessments, they should be aware of the potential implications outside the originally intended outcomes in determining an intervention’s value. The Impact Inventory provides an approach that allows these components to be considered along with local needs and priorities.
6.6 Conclusion and Next Steps
Identifying locally-relevant Best or Wasted Buys often requires adapting economic evaluations conducted in one country to local settings elsewhere. The process is challenging and requires careful examinations of data inputs, local data availability and other contextual factors relevant to specific settings. The framework and checklist provided in this chapter are intended to be used to assess transferability objectively and transparently in a practical manner. We recognize that others could expand the checklist to include other factors that may be relevant in particular circumstances and we would encourage this tool development. We hope that these tools serve as a useful guide to identifying locally-relevant Best or Wasted Buys.
Improving transparency and reporting in original studies would help an evaluator’s ability to assess the transferability of available evidence. Future areas for improving transferability across countries may include multi-national economic evaluations, international cost catalogues (https://ghcosting.org/) and an open-source platform to share decision-analytic models to which local data can be applied. Additionally, future research may examine whether each element of the checklist is equally important for assessing transferability and in what situations it is worthwhile to conduct a thorough transferability assessment considering the resources required for the task.
1 Tessa-Tan-Torres Edejer et al., ‘Making Choices in Health: WHO Guide to Cost-Effectiveness Analysis’, 2003, (Geneva: World Health Organization), https://www.who.int/choice/publications/p_2003_generalised_cea.pdf; Gillian D. Sanders et al., ‘Recommendations for Conduct, Methodological Practices, and Reporting of Cost-Effectiveness Analyses: Second Panel on Cost-Effectiveness in Health and Medicine’, JAMA, 316.10 (2016), 1093–103, https://doi.org/10.1001/jama.2016.12195; Thomas Wilkinson et al., ‘The International Decision Support Initiative Reference Case for Economic Evaluation: An Aid to Thought’, Value Health, 19.8 (2016), 921–28, https://doi.org/10.1016/j.jval.2016.04.015
2 Randa Eldessouki and Marilyn Dix Smith, ‘Health Care System Information Sharing: A Step toward Better Health Globally’, Value in Health Regional Issues, 1.1 (2012), 118–20, https://doi.org/10.1016/j.vhri.2012.03.022; Yot Teerawattananon, ‘Thai Health Technology Assessment Guideline Development’, Journal of the Medical Association of Thailand, 91.6 (2011), 11; Pharmaceutical Benefits Advisory Committee, Guidelines for Preparing Submissions to the Pharmaceutical Benefits Advisory Committee (PBAC) Version 5.0 (Canberra: Department of Health; 2016); Michael D. Rawlins and Anthony J. Culyer, ‘National Institute for Clinical Excellence and Its Value Judgments’, BMJ: British Medical Journal, 329.7459 (2004), 224–27, https://doi.org/10.1136/bmj.329.7459.224; Health Intervention and Technology Assessment Program (HITAP), Guide to Health Economic Analysis and Research (GEAR) Online Resource: Guidelines Comparison, 2019, http://www.gear4health.com/gear/health-economic-evaluation-guidelines
3 Catherine Pitt et al., ‘Foreword: Health Economic Evaluations in Low- and Middle-Income Countries: Methodological Issues and Challenges for Priority Setting’, Health Econ, 25 Suppl 1 (2016), 1–5, https://doi.org/10.1002/hec.3319
4 Health Intervention and Technology Assessment Program (HITAP); Benjarin Santatiwongchai et al., ‘Methodological Variation in Economic Evaluations Conducted in Low-and Middle-Income Countries: Information for Reference Case Development’, PLoS One, 10.5 (2015), e0123853, https://doi.org/10.1371/journal.pone.0123853
5 Wilkinson et al.
6 Michael F. Drummond et al., ‘Issues in the Cross-National Assessment of Health Technology’, International Journal of Technology Assessment in Health Care, 8.4 (1992), 670–82; M. Drummond, F. Augustovski et al., ‘Challenges Faced in Transferring Economic Evaluations to Middle Income Countries’, International Journal of Technology Assessment in Health Care, 31.6 (2015), 442–48, https://doi.org/10.1017/s0266462315000604
7 Center for the Evaluation of Value and Risk in Health (CEVR) Tufts Medical Center, Global Health CEA Registry, 2018, http://healtheconomics.tuftsmedicalcenter.org/ghcearegistry/
8 Wilkinson et al.; S. Horton, ‘Cost-Effectiveness Analysis in Disease Control Priorities’, in Disease Control Priorities, ed. by D. Jamison et al. (Washington, DC: World Bank, 2017), 3rd edn., IX, pp. 145–56.
9 Saskia Knies et al., ‘The Transferability of Economic Evaluations: Testing the Model of Welte’, Value Health, 12.5 (2009), 730–38, https://doi.org/10.1111/j.1524-4733.2009.00525.x; Katharina Korber, ‘Potential Transferability of Economic Evaluations of Programs Encouraging Physical Activity in Children and Adolescents across Different Countries — a Systematic Review of the Literature’, International Journal of Environmental Research and Public Health, 11.10 (2014), 10606–21, https://doi.org/10.3390/ijerph111010606; Brigitte A. B. Essers et al., ‘Transferability of Model-Based Economic Evaluations: The Case of Trastuzumab for the Adjuvant Treatment of HER2-Positive Early Breast Cancer in the Netherlands’, Value Health, 13.4 (2010), 375–80, https://doi.org/10.1111/j.1524-4733.2009.00683.x; Marrit Berg et al., ‘Model-Based Economic Evaluations in Smoking Cessation and Their Transferability to New Contexts: A Systematic Review’, Addiction, 112.6 (2017), 946–67, https://doi.org/10.1111/add.13748
10 Ron Goeree et al., ‘Transferability of Health Technology Assessments and Economic Evaluations: A Systematic Review of Approaches for Assessment and Application’, Clinicoeconomics and Outcomes Research, 3 (2011), 89–104, https://doi.org/10.2147/CEOR.S14404; M. Angel Barbieri et al., ‘What Do International Pharmacoeconomic Guidelines Say about Economic Data Transferability?’, Value Health, 13.8 (2010), 1028–37, https://doi.org/10.1111/j.1524-4733.2010.00771.x
11 M. F. Drummond et al., Issues in the Cross-National Assessment of Health Technology.
12 Bernie J. O’Brien, ‘A Tale of Two (or More) Cities: Geographic Transferability of Pharmacoeconomic Data’, Am J Manag Care, 3 Suppl (1997), S33–9.
13 Mark J. Sculpher et al., ‘Generalisability in Economic Evaluation Studies in Healthcare: A Review and Case Studies’, Health Technology Assessment, 8.49 (2004), https://doi.org/10.3310/hta8490
14 Robert Welte et al., ‘A Decision Chart for Assessing and Improving the Transferability of Economic Evaluation Results between Countries’, Pharmacoeconomics, 22.13 (2004), 857–76, https://doi.org/10.2165/00019053-200422130-00004
15 Stephanie Boulenger et al., ‘Can Economic Evaluations Be Made More Transferable?’, European Journal of Health Economics, 6.4 (2005), 334–46, https://doi.org/10.1007/s10198-005-0322-1; John Nixon et al., ‘Guidelines for Completing the EURONHEED Transferability Information Checklists’, European Journal of Health Economics, 10.2 (2009), 157–65, https://doi.org/10.1007/s10198-008-0115-4
16 Andrea Manca and Andrew R. Willan, ‘‘Lost in Translation’: Accounting for Between-Country Differences in the Analysis of Multinational Cost-Effectiveness Data’, Pharmacoeconomics, 24.11 (2006), 1101–19, https://doi.org/10.2165/00019053-200624110-00007
17 Ron Goeree et al., ‘Transferability of Economic Evaluations: Approaches and Factors to Consider When Using Results from One Geographic Area for Another’, Current Medical Research Opinion, 23.4 (2007), 671–82, https://doi.org/10.1185/030079906x167327
18 Boulenger et al.; Fernando Antonanzas et al., ‘Transferability Indices for Health Economic Evaluations: Methods and Applications’, Health Economics, 18.6 (2009), 629–43, https://doi.org/10.1002/hec.1397
19 Michael J. Drummond et al., ‘Transferability of Economic Evaluations across Jurisdictions: ISPOR Good Research Practices Task Force Report’, Value in Health, 12.4 (2009), 409–18, https://doi.org/10.1111/j.1524-4733.2008.00489.x
20 Michael Drummond et al., ‘Challenges Faced in Transferring Economic Evaluations to Middle Income Countries’, International Journal of Technology Assessment in Health Care, 31.6 (2015), 442–48, https://doi.org/10.1017/s0266462315000604
21 Olena Mandrik et al., ‘Transferability of Economic Evaluations To Central and Eastern European and Former Soviet Countries’, Value in Health, 17.7 (2014), A443-4, https://doi.org/10.1016/j.jval.2014.08.1172
22 G. Stewart et al., ‘A Systematic Review of Economic Evaluations in Latin America: Assessing the Factors That Affect Adaptation and Transferability of Results’, Value in Health, 18.7 (2015), A813, https://doi.org/10.1016/j.jval.2015.09.218
23 Wilkinson et al.
24 Welte et al.; D. K. Heyland et al., ‘Economic Evaluations in the Critical Care Literature: Do They Help Us Improve the Efficiency of Our Unit?’, Critical Care Medicine, 24.9 (1996), 1591–98; Helmut Spath et al., ‘Analysis of the Eligibility of Published Economic Evaluations for Transfer to a Given Health Care System. Methodological Approach and Application to the French Health Care System’, Health Policy, 49.3 (1999), 161–77.
25 Michael F. Drummond et al., Methods for the Economic Evaluation of Health Care Programmes (Oxford: Oxford University Press, 2015); Peter J. Neumann et al., Cost-Effectiveness in Health and Medicine (New York: Oxford University Press, 2017).
26 David D. Kim et al., ‘Worked Example 1: The Costeffectiveness of Treatment for Individuals with Alcohol Use Disorders: A Reference Case Analysis’, in Cost-Effectiveness in Health and Medicine, ed. by Peter J. Neumann et al. (New York: Oxford University Press, 2017), pp. 385–430.
27 David D Kim et al., ‘The Influence of Time Horizon on Results of Cost-Effectiveness Analyses’, Expert Review of Pharmacoeconomics Outcomes Research, 17.6 (2017), 615–23, https://doi.org/10.1080/14737167.2017.1331432.
28 Wilkinson et al.; Michael F Drummond et al., Methods for the economic evaluation of health care programmes; Neumann et al.
29 Michael F. Drummond et al., Methods for the economic evaluation of health care programmes; Neumann et al.
30 Wilkinson et al.
31 Wilkinson et al.; Michael F. Drummond et al., Methods for the Economic Evaluation of Health Care Programmes; Sanders et al.; Don Husereau et al., ‘Consolidated Health Economic Evaluation Reporting Standards (CHEERS) Statement’, BMJ, 346 (2013), f1049, https://doi.org/10.1186/1741-7015-11-80; M. F. Drummond and T. O. Jefferson, ‘Guidelines for Authors and Peer Reviewers of Economic Submissions to the BMJ. The BMJ Economic Evaluation Working Party’, BMJ, 313.7052 (1996), 275–83; Alan Williams, ‘The Cost-Benefit Approach’, Br Med Bull, 30.3 (1974), 252–56; Zoë Philips et al., ‘Good Practice Guidelines for Decision-Analytic Modelling in Health Technology Assessment: A Review and Consolidation of Quality Assessment’, Pharmacoeconomics, 24.4 (2006), 355–71, https://doi.org/10.2165/00019053-200624040-00006
32 Sanders et al.; Wilkinson et al.; Joshua J. Ofman et al., ‘Examining the Value and Quality of Health Economic Analyses: Implications of Utilizing the QHES’, J Manag Care Pharm, 9.1 (2003), 53–61, https://doi.org/10.18553/jmcp.2003.9.1.53
33 Center for the Evaluation of Value and Risk in Health (CEVR) Tufts Medical Center; Joanna Emerson et al., ‘Adherence to the IDSI Reference Case among Published Cost-per-DALY Averted Studies’, PLOS ONE, 14.5 (2019), e0205633, https://doi.org/10.1371/journal.pone.0205633
34 Barbieri et al.; O’Brien; Sculpher et al.; Welte et al.; Boulenger et al.; Goeree et al.
35 Thomas J. Hoerger et al., ‘Screening for Type 2 Diabetes Mellitus: A Cost-Effectiveness Analysis’, Annals of International Medicine, 140.9 (2004), 689–99, https://doi.org/10.7326/0003-4819-140-9-200405040-00008
36 Barbieri et al.
37 Barbieri et al.; Sculpher et al.; Welte et al.
38 Barbieri et al.; Sculpher et al.; Boulenger et al.; Goeree et al.; Michael Drummond et al., ‘Increasing the Generalizability of Economic Evaluations: Recommendations for the Design, Analysis, and Reporting of Studies’, International Journal of Technology Assessment in Health Care, 21.2 (2005), 165–71, https://doi.org/10.1017/s0266462305050221
39 Michael F. Drummond et al., Methods for the economic evaluation of health care programmes; Neumann et al.
40 Francis Guillemin et al., ‘Cross-Cultural Adaptation of Health-Related Quality of Life Measures: Literature Review and Proposed Guidelines’, Journal of Clinical Epidemiology, 46.12 (1993), 1417–32, https://doi.org/10.1016/0895-4356(93)90142-n; Roger T. Anderson et al., ‘A Review of the Progress towards Developing Health-Related Quality-of-Life Instruments for International Clinical Studies and Outcomes Research’, Pharmacoeconomics, 10.4 (1996), 336–55, https://doi.org/10.2165/00019053-199610040-00004
41 Jeffrey A. Johnson et al., ‘Valuations of EQ-5D Health States: Are the United States and United Kingdom Different?’, Medical Care, 43.3 (2005), 221–28, https://doi.org/10.1097/00005650-200503000-00004; Katia Noyes et al., ‘The Implications of Using US-Specific EQ-5D Preference Weights for Cost-Effectiveness Evaluation’, Medical Decision Making, 27.3 (2007), 327–34, https://doi.org/10.1177/0272989X07301822
42 ‘EQ-5D Instruments — EQ-5D’, https://euroqol.org/eq-5d-instruments/
43 Joshua A. Salomon et al., ‘Disability Weights for the Global Burden of Disease 2013 Study’, Lancet Glob Health, 3.11 (2015), e712–23, https://doi.org/10.1016/S2214-109X(15)00069-8
44 Sculpher et al.; Welte et al.
45 Shukri F. Mohamed et al., ‘Prevalence and Factors Associated with Pre-Diabetes and Diabetes Mellitus in Kenya: Results from a National Survey’, BMC Public Health, 18.Suppl 3 (2018), 1215, https://doi.org/10.1186/s12889-018-6053-x; Sanjay Basu et al., ‘Comparative Effectiveness and Cost-Effectiveness of Treat-to-Target versus Benefit-Based Tailored Treatment of Type 2 Diabetes in Low-Income and Middle-Income Countries: A Modelling Analysis’, Lancet Diabetes & Endocrinology, 4.11 (2016), 922–32, https://doi.org/10.1016/s2213-8587(16)30270-4; Elliot Marseille et al., ‘The Cost-Effectiveness of Gestational Diabetes Screening Including Prevention of Type 2 Diabetes: Application of a New Model in India and Israel’, Journal of Maternal-Fetal & Neonatal Medicine, 26.8 (2013), 802–10, https://doi.org/10.3109/14767058.2013.765845; N. Lohse et al., ‘Development of a Model to Assess the Cost-Effectiveness of Gestational Diabetes Mellitus Screening and Lifestyle Change for the Prevention of Type 2 Diabetes Mellitus’, International Journal of Gynaecology & Obstetrics, 115 Suppl (2011), S20–5, https://doi.org/10.1016/s0020-7292(11)60007-6; Melanie Y. Bertram et al., ‘Assessing the Cost-Effectiveness of Drug and Lifestyle Intervention Following Opportunistic Screening for Pre-Diabetes in Primary Care’, Diabetologia, 53.5 (2010), 875–81, https://doi.org/10.1007/s00125-010-1661-8; Stephen Colagiuri and Agnes E. Walker, ‘Using an Economic Model of Diabetes to Evaluate Prevention and Care Strategies in Australia’, Health Affairs (Millwood), 27.1 (2008), 256–68, https://doi.org/10.1377/hlthaff.27.1.256; Dana Goldman et al., ‘The Value of Elderly Disease Prevention’, Forum Health Economics Policy, 9.2 (2006), https://doi.org/10.2202/1558-9544.1004
46 Jemimah W. Mwakisha and O. K. A. Sakuya, Building Health: Kenya’s Move to Universal Health Coverage (WHO Africa, 2018), https://www.afro.who.int/news/building-health-kenyas-move-universal-health-coverage
47 Global Burden of Disease Collaborative Network, Global Burden of Disease Study 2017 (Seattle, United States: Institute for Health Metrics and Evaluation (IHME), 2019), http://ghdx.healthdata.org/gbd-results-tool
48 Jemimah W. Mwakisha and O. K. A. Sakuya; ‘Focus on Infrastructure, Staffing as Kenya Rolls out Universal Healthcare’, Business Daily, 2018, https://www.businessdailyafrica.com/datahub/Kenya-rolls-out-universal-healthcare/3815418-4889486-6tmjej/index.html; Elizabeth Merab, ‘Road to UHC: What It Will Take to Achieve Health for All’, Daily Nation (Nairobi City, Kenya, 2018), https://www.nation.co.ke/health/Road-to-UHC-what-it-will-take--to-achieve-health-for-all/3476990-4655230-jtp203z/index.html
49 Fredrick Nzwili, ‘Kenya To Launch Universal Health Coverage Pilot Of Free Healthcare,’ (Health Policy Watch, 2018), https://www.healthpolicy-watch.org/kenya-to-launch-universal-health-coverage-pilot-of-free-healthcare/
50 Mohamed et al.
51 Global Burden of Disease Collaborative Network.
52 Wilkinson et al.
53 Ibid.
54 Basu et al.
55 Ibid.
56 Goldman et al.
57 Joshua T. Cohen et al., ‘A Call for Open-Source Cost-Effectiveness Analysis’, Annals of Internal Medicine, 167.6 (2017), 432–33, https://doi.org/10.7326/l17-0695
58 Marseille et al.; Lohse et al.; Bertram et al.; Colagiuri and Walker.
59 Neumann et al.