10. How Conservation Practice Can Generate Evidence
© 2022 Chapter Authors, CC BY-NC 4.0 https://doi.org/10.11647/OBP.0321.10
Conservation practice provides a considerable opportunity to generate new evidence to inform future decision-making. Substantial resources are currently invested in data collection and monitoring, yet too often these are ineffectively designed, meaning the data gathered contributes little to building an evidence base. However, thinking in advance about how actions are implemented, data are collected, and results are shared can greatly increase the usefulness of the results. Controls, comparisons, replication, randomisation, and preregistration can all improve the value of the data collected.
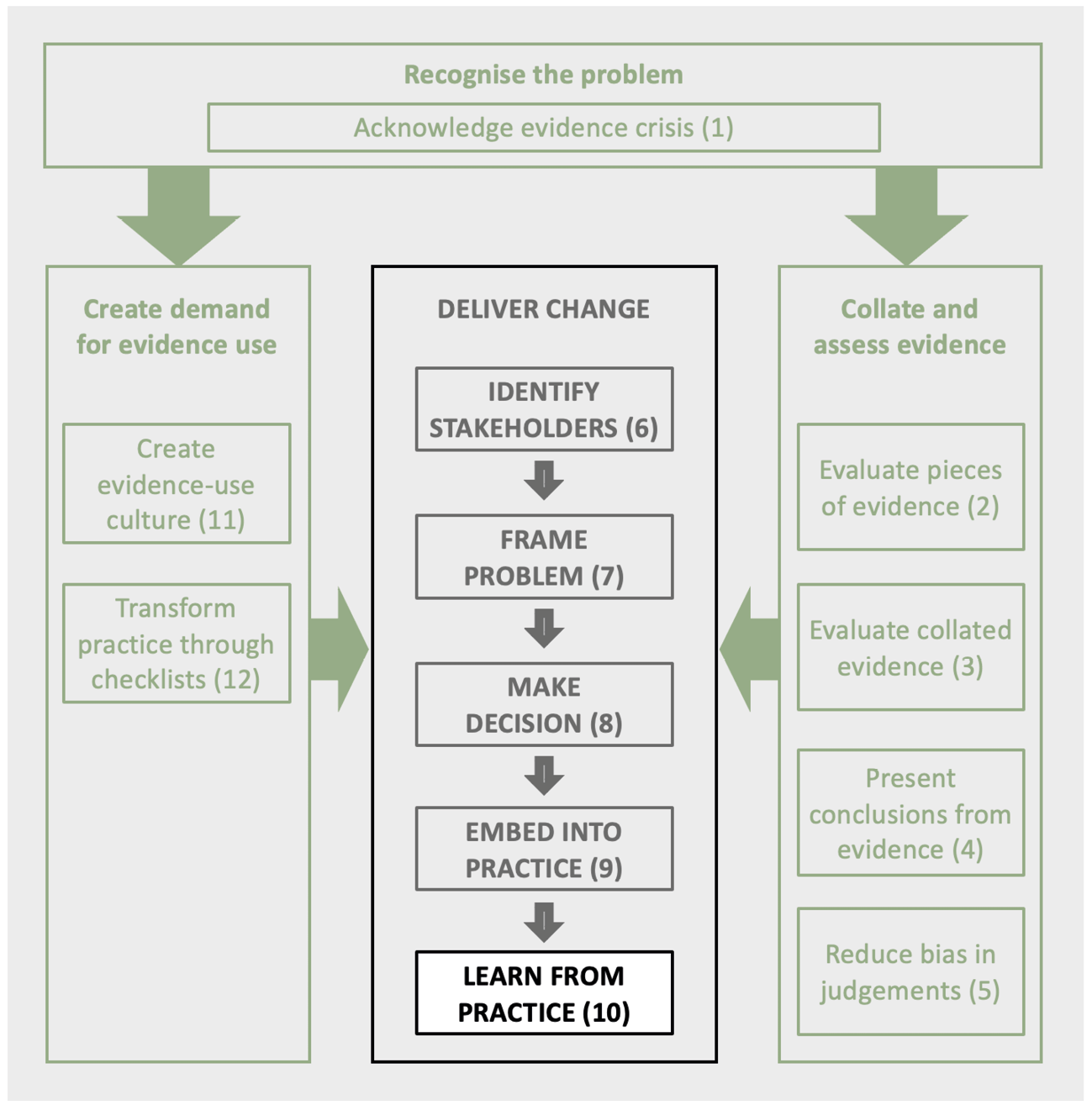
1 Endangered Landscapes Programme, Cambridge Conservation Initiative, The David Attenborough Building, Cambridge, UK
2 Department of Biological Sciences, University of Toronto-Scarborough, 1265 Military Trail, Toronto, ON, M1C 1A4, Canada
3 Digital Geography Lab, Department of Geosciences and Geography, Faculty of Sciences, University of Helsinki, Helsinki, Finland
4 The Center for Behavioral & Experimental Agri-Environmental Research, Johns Hopkins University, Baltimore, Maryland, USA
5 Fauna & Flora International, The David Attenborough Building, Pembroke Street, Cambridge, UK
6 RSPB Centre for Conservation Science, RSPB Scotland, North Scotland Office, Etive House, Beechwood Park, Inverness, UK
7 Cairngorms Connect, Achantoul, Aviemore, UK
8 Conservation Science Group, Department of Zoology, University of Cambridge, The David Attenborough Building, Pembroke Street, Cambridge, UK
10.1 Ensuring Data Collection is Useful
Conservationists need better evidence about the effectiveness of their actions. There are substantial gaps in the evidence (Christie et al., 2020) and many straightforward questions are unanswered (Sutherland et al., 2022). Filling such gaps would greatly enhance the effectiveness of conservation practice.
Such gaps persist despite the fact that conservation practitioners routinely collect large amounts of data that describe the interventions they undertake and the subsequent ecological and socio-economic conditions. These monitoring efforts generally aim to measure the progress of conservation projects but, despite considerable effort and resources dedicated to data collection, conservation projects are rarely designed to demonstrate the link between action and effect (Legg and Nagy, 2006). Embedding experimental designs in conservation projects is the most effective means of demonstrating a link between an action and its effect, yet is seldom used. On the occasions when projects do link action and effect, the results may not be disseminated sufficiently to ensure they contribute to the wider evidence base.
The beauty of planned experimental designs is that conservation projects can focus their attention on collecting data from a specified set of sites for a focused set of informative indicators. This allows them to move away from long lists of indicators collected across large areas that may have used in the past, with little idea of how the data collected could be applied. With a little forward planning of intervention design, data collection and dissemination, conservation projects could yield much more useful information. The resulting improved evidence base may lead to result in better-informed, and hence more effective, conservation decision-making.
This chapter has three main sections. Firstly, we discuss which different approaches to data collection, including measuring outputs, measuring outcomes, testing actions, or evaluating impact, are best applied to selected circumstances. Secondly, we describe the principles of experimental design. Finally, we describe how the evidence generated from well-designed experiments can be effectively shared with the wider community.
There are two main messages that we wish to convey. Firstly, embedding experimental tests into conservation practice is often less challenging than it may initially appear and should become routine. Secondly, the details of the experimental design really matter — better designed experiments are much more likely to produce useful and accurate results.
10.1.1 Standardising methods and outcomes
One major challenge in assessing the effectiveness of conservation actions is a lack of standardisation in how projects are implemented and evaluated. For instance, Cadier et al. (2020) found that 238 different indicators had been used to measure coastal wetland restoration across just 133 projects. The complexity of natural systems means that a range of methodological approaches and indicators is warranted, but such an extreme lack of consistency renders it difficult to synthesise, compare, and draw inferences across different projects.
One possible solution is that expert working groups develop and agree on standards for measuring and reporting conservation outcomes. For example, Sutherland et al. (2010) developed a set of minimum standards for documenting and monitoring bird translocation and reintroduction projects. These aimed to facilitate the collection of comparable data that could be more effectively combined to detect patterns in the causes of successes and failures.
Another approach to ensuring consistency in reporting across projects is demonstrated by the Mangrove Restoration Tracker Tool (Leal and Spalding, 2022). This tool has been co-designed by mangrove scientists, NGO staff and restoration practitioners from around the world, and provides a framework to collect data on all aspects of a mangrove restoration project, from design, through implementation to monitoring. Each project that uses the tool will record a comprehensive and consistent, yet easily collected, set of ecological and social metrics, alongside baseline information describing the site and actions undertaken. As this tool accumulates comparable data from a wide range of projects, it will permit rapid synthesis, making it easier to identify the most successful and cost-effective approaches and allowing a better assessment of progress toward national and global restoration targets.
Standardised methods also allow harmonised experiments to be carried out (Ferraro and Agrawal, 2021). Here, multiple teams carry out parallel experiments, using agreed standards and data collection to examine the generalisability of results and determine whether results are condition-dependent.
In the absence of an agreed method for monitoring a conservation intervention, a sensible rule is to adopt an already widely used technique where possible. The aim is to avoid seemingly trivial changes in methodology and data collection that render direct comparisons between studies impossible.
10.1.2 Adaptive management and learning
An advantage of embedding experimental designs into conservation projects is the potential to improve future management. Adaptive management entails information describing the progress and effectiveness of project actions being fed back to inform future management decisions (Figure 10.1, Walters and Holling, 1990). Furthermore, generating evidence within a project provides project managers with confidence about its relevance.
Evidence will have much greater benefits if it is also shared with the wider conservation community in addition to being used in adaptive management (Figure 10.1, Section 10.6). If the majority of conservation projects committed to some routine testing and documentation, then the massively enlarged evidence base could revolutionise effectiveness, fully justifying the costs required by each organisation (Rey Benayas et al., 2009).
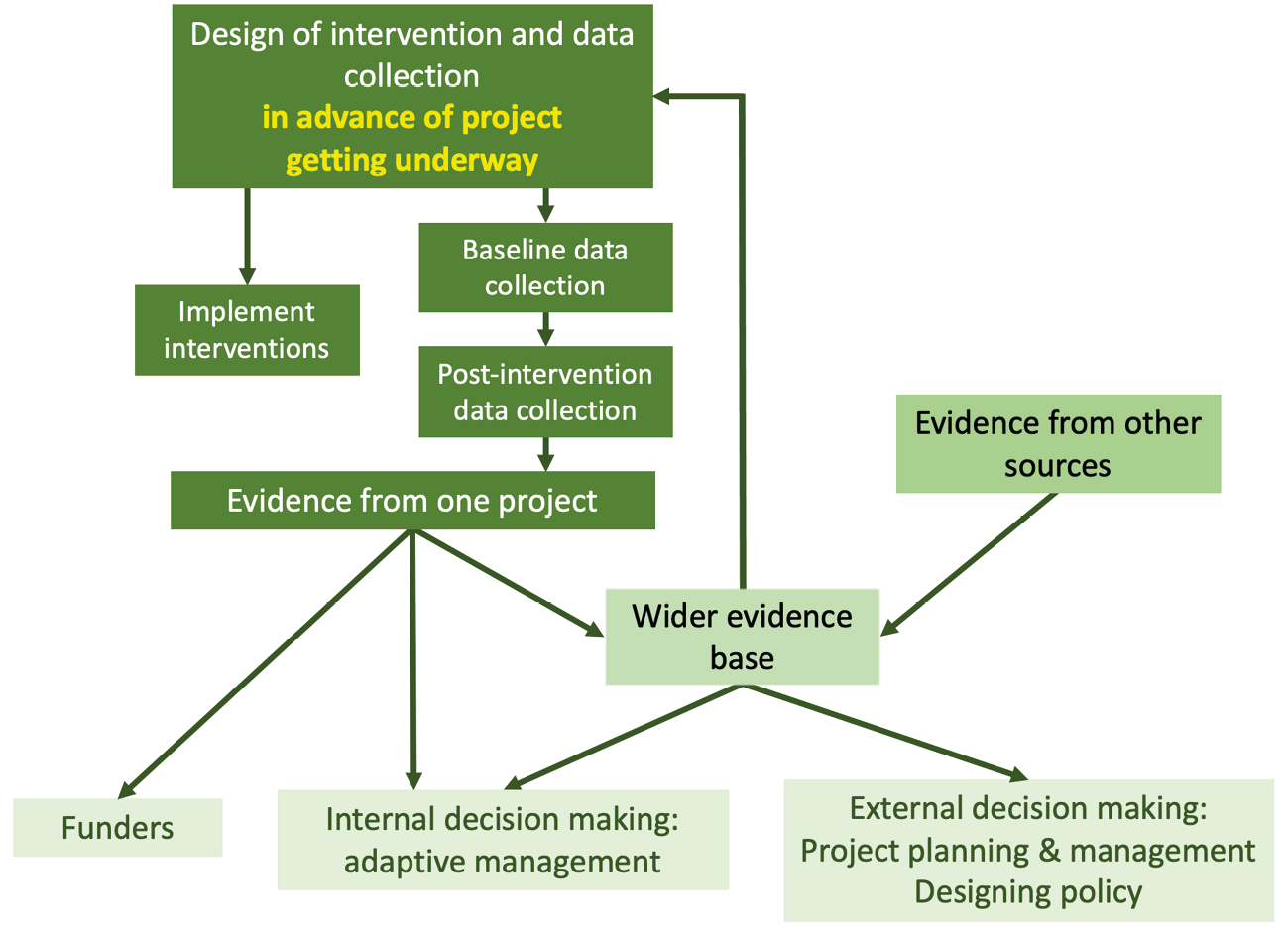
Figure 10.1 The flow of evidence, from appropriately designed data collection to a wider evidence base to inform internal and external decision making. (Source: authors)
10.2 Collecting Data Along the Causal Chain
Project planning often involves developing a theory of change (Figure 10.2) showing how actions are expected to lead to the desired change in outcomes and the target. Data can be collected at each stage along this causal chain (Table 10.1): this section considers the relative merits of each stage.
There is often a demand, from funders or others, to demonstrate the ultimate impact of a project — the changes in the target as a consequence of the actions undertaken (right-hand box of Figure 10.2). However, in moving through the causal change from left to right, the link to each action becomes weaker due to the effects of other actions and known and unknown external factors. Therefore, unless a project is deliberately designed to measure or control for these effects, practitioners cannot separate the effect of their actions from that of other external, uncontrolled factors that also affect the project outcomes or the ultimate target variable. To isolate the contribution of the conservation actions, practitioners need a way to estimate the counterfactual value of the target that would be seen in identical conditions except for the absence of the conservation actions. The effect of the project actions can then be attributed to the difference between the project site and the counterfactual. Estimating counterfactual outcomes requires data from control or comparison sites where no project actions take place. Because of the challenges of finding such identical conditions in the natural ecosystems where conservation takes place, collecting data at multiple sites, both with and without the project action, will greatly increase our confidence that any observed difference is due to the actions implemented.
_FINAL.jpg)
Figure 10.2 Options for collecting data along the causal chain. A conservation project consists of a series of actions. Each action (organise workshops, fund creation of islands for nesting birds) is implemented at a particular scale, context and efficiency, which determines the outputs (fishers attend workshops, islands created). These outputs may then have a direct outcome (bycatch of seabirds reduced, birds nest on islands), which may be additive or interactive (dotted line). The outcomes can result in changes in the ultimate target (seabird populations). Each stage may be influenced by known (climate, another project) and unknown (changes in fish abundance, an undocumented predator) external factors. The relative importance of external factors will usually increase along the chain. The figure also shows a range of processes: evidence-based practice (adopting measures shown to be effective), project delivery (ensuring actions are delivered), assessing the outcomes of actions (testing effectiveness) and testing the theory of change. (Source: authors)
Table 10.1 The different stages of a project life cycle (see Figure 10.1) at which data can be collected, with examples of the type of data at each stage. Not all projects will collect data at all stages. Each stage is described in the relevant section in the text below.
In a review of monitoring in conservation, Mascia et al. (2014) identified that conservation practice tends to be good at ambient monitoring (measuring status and change in ambient social and ecological conditions, independent of any conservation intervention), measures for assessing how management is progressing (inputs, actions, and outputs) and measures for assessing performance (assessing progress toward desired levels of specific actions, outputs, and outcomes). However, it is much weaker at determining the intended and unintended causal impacts of conservation interventions or synthesising findings to improve practice. They concluded that this is a serious gap as ensuring that such learning is generated, and builds on existing knowledge, is key to delivering improved conservation outcomes (as shown in Figure 10.1).
In practice, a monitoring and evaluation programme for a project is likely to comprise a number of the approaches described in Table 10.1. For example, the landscape restoration projects funded by the Endangered Landscapes Programme, based in the Cambridge Conservation Initiative, collect information to monitor their outputs (generally used internally by organisations to assess project progress), and their outcomes (to report to funders and others). Each project also has to include a documented test of an action (to improve the project and society’s understanding of what does and does not work).
10.2.1 Monitoring project progress — outputs
A routine element of project management is to keep a record of the delivery of project outputs — the activities or deliverables produced by the project. For example, a project that aimed to reduce mangrove loss by raising awareness and changing the behaviour of shrimp farmers may include the output ‘Hold a series of workshops for shrimp farmers’. The output could then be measured via the number of workshops held and the number of farmers that participated. This is useful information that can be used internally, or reported to funders, to ensure that the project is on track with its planned programme of work.
However in conservation, outputs and outcomes may not be directly linked (the workshops may be well attended but not result in behaviour change); unless this link is proven, outputs cannot be used to reliably measure the success of an action or project. This has been a major criticism of many recent large-scale tree-planting initiatives, carried out as part of efforts to mitigate climate change, where success has often been measured by the number of trees planted, rather than by any long-term ecological or carbon sequestration benefit (Lee et al., 2019). In many cases the survival rate of trees is very low, meaning simple monitoring of outputs is not an accurate assessment of success in the medium or long term.
However, in cases where the action is already known to be effective (i.e. the link between output and outcome is proven), measuring outputs may be sufficient to assess project success. Thus in health, a clinic delivering vaccines that are known to be effective at improving health outcomes may report success as the number of people vaccinated, rather than looking at subsequent disease rates in the community. This is the ‘project delivery’ box in Figure 10.2. An ecological equivalent might be to provide barn owl nest boxes, which are well known to be effective (Johnson 1994, Bank et al. 2019), to farms with suitable habitat along with instructions for their positioning, but not ask for any monitoring.
10.2.2 Monitoring project results — outcomes
In most circumstances, monitoring outcomes rather than outputs will provide a much better measure of a project’s success (Figure 10.2). Outcomes tend to refer to the medium-term effects of actions taken, and often relate to the objectives of a project. Examples could include the number of trees that are alive after 5 years (rather than the number of trees planted), the rate of adoption of the new fishing technique (rather than the number of fishers attending workshops), or the number of tourists visiting a national park (rather than the creation of a new visitor centre).
The challenge when monitoring outcomes is to know how much of any observed change can be attributed to a project’s actions. For actions known to be effective, where the casual chain is simple and understood, and where there are few other potentially confounding factors, it may be reasonable to attribute the change in the outcome to the actions undertaken. For example, creating some small islands and counting the number of birds nesting on them is likely to be a reasonable approach to assessing effectiveness. But in many cases, with more complex causal chains and the potential for considerable impacts from external factors, it is difficult to confidently attribute any observed changes in an outcome to the project’s actions.
10.2.3 Testing effectiveness of actions
An excellent option for improving our understanding of the consequences of conservation actions is to identify opportunities to experimentally test single actions as part of conservation practice (Section 10.3). Although such tests are rarely currently included in conservation projects, there is an appetite to routinely include an element of testing in funding proposals for conservation projects amongst funders and practitioners alike. When asked what proportion of conservation grants should be allocated to testing intervention effectiveness, there was considerable overlap in their responses, with practitioners tending to prefer slightly larger percentages (median 3–6%) than funders (median 1–3%) (Tinsley-Marshall et al., 2022).
10.2.4 Various definitions of ‘impact’
Project managers may be asked to demonstrate the difference that a project or programme of work has made to its ultimate target(s) (Figure 10.2). Funders in particular are often interested in such measures of overall impact, to show that the interventions that they have supported have brought about change. However, across different parts of the conservation community, there is variation in the use and meaning of the terms impact and impact evaluation. In much of the conservation community, impact describes the changes in the ultimate target that take place between the beginning and end of a project, without necessarily rigorously demonstrating attribution. We refer here to this general meaning of impact as descriptive impact (Table 10.1). In contrast, in the impact evaluation community impact has a different and more precise meaning, where it refers to the change in target status during a project in comparison to a control or counterfactual where no action is taken (White, 2010). Here, we refer to this experimental form of impact as causal impact. As an example, the descriptive impact of a project could describe the changes observed in its target at the end compared to the start (e.g. the change in fish populations and local livelihoods at the end of a reef restoration programme), while the causal impact would require a comparison of these changes with a counterfactual (e.g. the difference in the changes in fish populations and livelihoods at the project site compared to another similar site where no actions were taken, 10.3.5).
Prior to applying any specific impact evaluation method or study design, it is important to clearly define the scope of the project and the outcome variables of interest. The following five steps outlined by Glewwe and Todd (2022) are useful when starting to design a causal impact evaluation:
- Clarify the project and outcome variables of interest.
- Formulate a theory of change to define and refine the evaluation questions.
- Depict the theory of change in a results chain i.e. inputs => action=> outputs => outcomes => target (impact)
- Formulate specific hypotheses for the impact evaluation.
- Select performance indicators for monitoring and evaluation.
- Design a sampling program and the details of analyses.
10.2.5 Monitoring final target of actions: descriptive impact
Descriptive impact is the change seen in the final target when a project finishes, without any attribution of the change to the actions undertaken. This approach is very commonly used across conservation projects for a number of reasons: a package of actions is often implemented together, it is challenging and resource intensive to find and monitor a comparable control site, the mechanisms linking actions and outcomes are poorly understood, and a culture of experimental testing is not widespread among conservation organisations or funders. Although simple monitoring of changes in the targets of a project is widely used and potentially useful, it is important to recognise the limitations and to ensure that appropriate caveats are attached.
As with the discussion of outcomes (section 10.2.2), the adequacy of using descriptive impact to assess the success of a project depends on the strength of the causal change and the likely impact of external factors. Consider a project removing a barrier in a river to allow the movement of migratory fish. If there were no such fish present upstream when the barrier existed, but after removal both fish and spawn are recorded, it is probably unnecessary to monitor another river, where no such barrier removal took place, in order to attribute the improved status of the fish population to the project. In this case, simply monitoring a before-and-after change along the length of the river is sufficient to demonstrate project impact. In another project, deer numbers are reduced to allow tree regeneration, and after two years the change in the number of tree seedlings is monitored. Without comparing these results to an area without reduced deer numbers, it is difficult to assess the success of the project: any regeneration may actually be due to the unusually suitable weather or a collapse in the rabbit population. Descriptive impacts often comprise a significant component of the results reported in project case studies (10.6.1).
10.2.6 Monitoring final target of actions: causal impact
To confidently assess the difference a conservation project has made, and hence quantify the causal impact, in most cases comparative data describing the target state either before or without the intervention (or both) are needed, in addition to an assessment of the final state of the target of the project. Section 10.3 describes how measures of causal impact can be achieved.
10.2.7 Ambient monitoring
Ambient monitoring involves collecting data describing ecological or socio-economic states but which is not directly aiming to understand the effects of particular actions or projects. For example, it may provide surveillance of the spread of an invasive species, the shift in a coastline or the national or regional trends in a species or ecosystem. This can provide a wider understanding, identify issues and indicate whether overall conditions are improving or deteriorating.
10.3 Incorporating Tests into Conservation Practice
Implementing agencies, funders and policy makers want to know the effectiveness of actions. However, as previously described, simply monitoring changes in the target variable rarely discriminates whether any changes seen were due to the actions undertaken, rather than other factors. Is the provision of predator-proof nest boxes responsible for an increase in a bird population, or was it a particularly good year for insects? Is a reduction in snaring related to a project’s support for alternative livelihoods or because young people are leaving the villages for the cities? Furthermore, the actions may have had beneficial effects on the target population, but these could be masked by a wider overall decline caused by other factors.
In order to attribute observed changes to an action, tests in which the changes in the target variable are compared in the presence and absence of the action are needed. This helps eliminate rival explanations, such as external factors, for the patterns observed in the monitoring data. The most robust tests, such as randomised controlled trials, include replication of treatments, comparisons or controls, and random allocation of treatments and controls. Studies that contain only one or two of these components of experimental designs are less robust, but still more informative than those that contain none. Quasi-experimental designs may be appropriate for interventions in which the treatment is already determined, such as an assessment of the effectiveness of marine reserves in which their location has already been decided (Section 10.4.3).
10.3.1 Why include a test?
Experimental tests are usually the best way of generating rigorous evidence for the effectiveness of particular actions (Ockendon et al., 2021). Routinely including tests in conservation projects will both increase the number of actions tested and the range of contexts in which they are tested. An increased number of studies on any particular action increases confidence in the results and enables consideration of heterogeneity and the variation in outcome between studies, meaning the evidence will be relevant for a wider range of users.
Of course the results of tests can also be used immediately by conservation organisations and projects to enable adaptive management (Section 10.1.3) and improve practice. This requires a deliberate process to embed learning and evidence generation into project implementation (Wardropper et al., 2022).
10.3.2 When to test actions?
A key skill is to be able to recognise where and when trials can be included most easily and effectively in conservation practice. The best opportunities occur when it is relatively straightforward to integrate a test into ongoing work (for example the same action is being carried out on multiple independent occasions); the necessary skills and capacities are available; and the results from the test are likely to be of interest to the organisation or others (Figure 10.3). Collaboration and codesign between stakeholders, the practitioner and academic partners can be an effective way to identify and capitalise on these opportunities (Kurle et al., 2022).
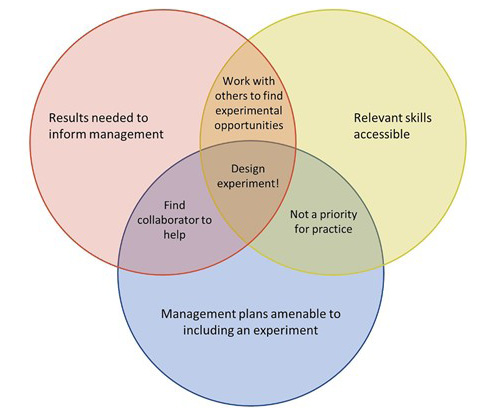
Figure 10.3 How a combination of skills, the need for results, and the existence of opportunities determines whether an experiment can usefully be included in conservation management. The optimal conditions for carrying out an experiment in practice arise when all three overlap. (Source: Ockendon et al., 2021, CC-BY-4.0)
Below we briefly consider the key principles of experimental and test design in the context of conservation practice.
10.4 Design of Experiments and Tests
There are five key elements (Crawley et al., 2015) that can improve the design of experiments and tests: (1) Randomisation; (2) Controls or comparisons; (3) Data sampled before and after an intervention or impact has occurred; (4) Temporal and spatial replication; (5) Preregistration. These elements can be combined in different ways, to give the six most commonly used test designs: Randomised controlled trials (RTC), Randomised before-after control-intervention (R-BACI), Before-after control-intervention (BACI), Before-after; Control-intervention; and After designs. These different designs vary considerably in their likelihood of producing an accurate answer, as tabulated in Table 2.5 (Christie, 2019, 2020b). Thus it is important to note that the components included in the design of a study make a significant difference to the likelihood of it giving useful results.
10.4.1 Randomised controlled trials
Randomised controlled trials (RCTs) and Randomised Before-After Control-Impact (R-BACI) experiments are the most rigorous study design for reliably and accurately estimating the magnitude and direction of an effect (Table 2.5, Christie, 2019, 2020b); therefore, where they are practically feasible, they should be the first choice for testing conservation actions. Although RCTs have a reputation for being complicated and difficult to implement in conservation, in practice they are carried out routinely, particularly when testing simple actions that can easily be replicated. This is reflected by the presence of over 1400 RCTs of conservation in the Conservation Evidence database — 16.7% of the total number (Christie et al. 2020b). For example, a simple replicated controlled trial demonstrated that the addition of an artificial, moulded ‘form’ into nest boxes for swifts Apus apus increased occupancy rates compared to boxes without such a ‘form’. This study, which monitored multiple swift nest boxes across four sites, demonstrated how replication, stratification and controls can be applied to generate evidence to inform future design using a straightforward and easy-to-implement trial design (Newell, 2019). There are innumerable opportunities to design such simple yet robust experiments, as shown by a group of conservation researchers and practitioners identifying a hundred possible experiments that could test the effectiveness of actions using an RTC and would also produce useful results for practice (Sutherland et al., 2022).
Carrying out RCTs at the level of conservation programmes or projects, as used in formal impact evaluation, may be more complicated in conservation, although the approach has been widely adopted in the medical and international development sectors (Ferraro and Pattanayak, 2006; White, 2010). As with any aspect of project design, the decision of whether to use an RTC will depend on the value of the data produced versus the time and resources required to set up, implement and monitor the trial, within any practical constraints that exist. Time and financial resources are frequently cited as limiting factors in the more widespread adoption of randomised controlled trials as a project evaluation approach in conservation (Curzon and Kontoleon, 2016).
10.4.2 Randomisation
If the controls and replicated plots are spatially clumped, with treatments in one area and controls in another, it is possible that confounding factors may bias the results. For example, if a study testing the effect of nest box height on breeding success was designed such that the boxes placed at 2m height were all on one side of the site, those at 3m in the middle section, and those at 4m at the other end of the site, then any gradient across the site in an underlying environmental factor that influences nesting success could override any effect of nestbox height. In such cases, controls and replication are insufficient; experimental units also need to be interspersed in space so that spatial confounding factors are random with respect to the treatments.
The most commonly used method to allocate interventions to plots or experimental units is to randomly assign them as controls or treatments. The simplest way to achieve this is to number each unit and use a random number generator (there are many online options) to assign each unit to treatment or control. This method works well if the study area is homogeneous and has a relatively large number of replicates. However, if the study includes a small number of larger plots, then randomisation can result in suboptimal plot distributions, if, for example, the three replicate control plots all happen to be assigned to one side of the study area. This is a particularly important issue if there is a known underlying gradient at the site. In these cases, blocking can be used prior to randomisation (either by creating contiguous experimental blocks, each containing a number of plots or sites or by matching plots into blocks by some other criterion, e.g., slope in peatlands, which links to hydrology and peat depth) to reduce the potential differences in environmental conditions between treatment and control sites.
10.4.3 Quasi-experimental designs or natural experiments
Sometimes, experimental tests with randomised allocations of treatments and controls are not feasible in conservation for ethical, logistical or political reasons. Alternatively, in some circumstances, for example, where an intervention is being carried out numerous times albeit not under the control of the data analyst (e.g. agri-environment prescriptions) statistical approaches can be used to estimate the impact of an interventions (Schleicher et al. 2020). Identifying opportunities to take advantage of such ‘natural experiments’ can greatly improve understanding of the effectiveness of national or regional policy decisions. In such cases, methodological advances in statistical approaches have allowed causal inferences to be drawn from non-experimental data (Ferraro and Pattanayak, 2006). These quasi-experimental designs allow us to maximise the potential learning from large observational datasets by using statistical approaches to overcome the potential biases that are likely where randomization is not possible (Schleicher et al., 2020). Such quasi-experimental statistical methods, including matching, instrumental variables, or difference in difference, have been widely used in other disciplines, particularly economics. In combination with remotely sensed data, they have huge potential for building the evidence base in conservation.
These methods attempt to identify treatment and control groups that are similar in their observed characteristics. An example could be a comparison of rates of forest loss inside a protected area with rates in areas that are not protected but are otherwise similar in terms of remoteness and accessibility (e.g. Eklund et al., 2016). In this example, distance from urban centres is a likely so-called confounding variable, as sites far from human settlements tend to be more likely to be assigned for protection but are also less prone to suffer from deforestation as they are harder to reach. Using quasi-experimental designs makes it possible to control for the effect of such confounding variables in order to obtain a true estimate of the impact of the treatment in question (here site protection).
Matching is one of the more commonly used impact evaluation methods in conservation because it lends itself so well to evaluating an intervention post hoc. With matching methods, it is possible to reduce differences between treatment and control units in terms of confounding variables, thus aiming to isolate the intervention effect. The idea is simply to pair each treatment unit with an observably similar control unit and then interpret any difference in their outcomes as the effect/impact of the project intervention. A major benefit of matching is that it has relatively few data requirements, but on the other hand it does assume that there are no unobserved confounders (Schleicher et al., 2020). In conservation, another possible difficulty is if control units that are similar enough to the treatment units simply no longer exist. For example, if forest only remains at high altitudes and these areas of forest are all protected, then there are no control units of unprotected forest at high altitudes available for the comparison. The best designs often combine approaches (Ferraro and Miranda, 2017).
It is important to acknowledge that not all conservation projects or programmes are amenable to experimental or quasi-experimental study designs and for many projects it will not be possible to collect data in a way that can reliably distinguish a treatment effect from the most plausible hidden biases (Ferraro, 2009). However, an understanding of counterfactual thinking and confounding factors is beneficial for any conservation project. As a practical step, most conservation projects, when preparing theories of change, could include a consideration of the possible confounding factors that might also affect the outcome of interest. Informed guesses or judgements can then be used to adapt project implementation to minimise or account for these biases. Key here is that project implementation could be adapted in response to both evidence generated from the project in question and evidence from analyses elsewhere with better internal and external validity (Ferraro, 2009).
10.4.4 Controls and comparisons
Some form of comparison is vital for assessing the magnitude of the impact of an intervention, although monitoring areas where no action has taken place has traditionally not been a priority for conservation. Comparing a species, habitat or community before and after an action has been carried out can provide some information about the change that has taken place, but results are vulnerable to other external factors and sources of bias that change through time, such as weather conditions, disease or climate change. The inclusion of an untreated control, with such a before-and-after comparison (a BACI design), significantly improves the value of the results (Christie et al., 2019).
Practical challenges are likely to have contributed to the rarity of the inclusion of controls or comparisons in conservation projects. These include that the immediate benefits of comparative treatments are not obvious to landholders or funders or that control areas are not covered by the same funding as areas of conservation activity. Related to this, management decisions at these control sites made over the course of the study may sometimes be made independently of the data collection needs of the experimental design. However, in many cases these challenges can be overcome once the value of including a comparison site is realised and communicated.
Although an untreated control is often the default comparator, it may be more informative to compare the results of a new intervention with ongoing or traditional management practice to assess their relative effectiveness and see if the new approach works better (Smith et al., 2014). For example, if a site manager is wondering whether changing the timing of herbicide application improves control of an invasive plant, then it would be much more useful to compare the results with an area where herbicide is applied at the conventional time, rather than an untreated area where no herbicide is applied at all.
There is no guarantee that another site selected as a control will not differ from the treatment site in some key aspects (such as soil moisture, land-use history or elevation), limiting its ability to serve as a robust comparison. To check this, vegetation, soil and environmental sampling should be conducted at the outset to determine if sites differ in any important aspects. Where feasible, subdividing each management site and retain areas within each that are untreated (blocking) will help minimise these biases. Replication (10.3.3) of treatment and control sites is also important in helping to demonstrate a causal link between interventions and changes in the target. For example, a study looking at the effectiveness of creating deadwood in forests in the Scottish highlands is testing three possible approaches (winching, cutting and ring-barking, plus an untreated control) on trees at five blocks of replicates in different areas of the forest. If the three treatments result in the same changes in the invertebrate community across each of these blocks, this will provide good evidence that it is the treatments, rather than another factor, that are causing these changes.
10.4.5 Replication
Replicating, the independent repeating of the action and control, increases the precision of the estimated effect and so will increase the confidence that observed results are due to the action taken rather than any other factor in the wider environment. Replication can take place either in space (e.g. multiple plots, community groups, protected areas) and/or through time (e.g. repeating treatments on multiple dates or across several years, Stewart-Oaten et al., 1986).
Formally, the number of replicates needed to detect a certain effect size with a specified probability can (if sufficient appropriate information is available) be calculated using a power analysis (Crawley, 2015). However, within the constraints of conservation, a general rule is ‘the more replicates the better’. More practically, a minimum of three replicates is required to estimate mean and variance. Larger numbers of replicates are more powerful and also allow for unforeseen and uncontrolled circumstances, such as part of the study site being flooded.
However, for many large-scale conservation interventions, such as reintroductions of animals or alterations to whole ecosystems (e.g. wetland creation or dam removal), there may be substantial or even insurmountable challenges to replication. In these cases, where the options for monitoring the effect of an action is to carry out an unreplicated trial or not to do it at all, there may well be benefits to carrying out unreplicated actions (e.g. Davies and Gray, 2015; Ockendon et al., 2021), especially if before- and after-treatment sampling is possible. If an unreplicated trial is undertaken, it is especially important that the necessary caveats are included in any interpretation of the results, in particular in relation to ascribing causality between the action and any changes seen in the target. Developing an understanding of the mechanism by which actions cause any observed changes in the target can aid the drawing of inferences from unreplicated studies.
Another justification for carrying out tests with very small numbers of replicates (or even none) is that, if multiple studies of the same or similar actions exist, these can be combined in meta-analyses to investigate the generality of the results (Gurevitch et al., 2001). For example, an analysis of the effects of eradicating invasive mammals on seabirds across 61 oceanic islands (where each individual study had n = 1) found that most seabird populations had increased, with a mean annual recovery rate of 1.12 (Brooke et al., 2018). Here, the results of an intervention that would be very difficult for a single project to replicate were combined across a large number of individual studies to produce an estimate of the average impact of the action.
10.4.6 Preregistration and project declarations
Conservation actions take place within highly complex and dynamic settings (Catalano et al., 2019). Therefore, the failure of conservation actions should be accepted as unsurprising. However, publication bias, whereby organisations and researchers only communicate positive outcomes and where journals preferentially publish positive results, is a pervasive problem in conservation (Wood 2020), with successful projects reported at four times the rate of unsuccessful ones (Catalano et al., 2019). From an individual or organisational standpoint, this may be driven by the need to maintain reputation, particularly when reporting to funders or applying for more funding to work on similar conservation actions. However, a bias towards reporting positive outcomes will inflate the apparent effectiveness of an action and may lead to other individuals or organisations applying it, in the mistaken belief that the action is generally effective. The failure to report negative outcomes means we lose a valuable opportunity to learn from past mistakes or to understand factors affecting success, and will likely result in wasted effort using less effective actions (Wood, 2020).
There is therefore a need to develop a model whereby the reporting of well-designed actions is the norm, and rewarded even when results are neutral or negative (Burivalova et al., 2019). One proposed solution is ‘pre-registration’, whereby the project design, data collection and analysis are defined and published prior to collecting data to measure the outcomes; this is the norm for medical trials (Nosek et al., 2019). One can always deviate from the pre-registered plan, but one should report that deviation. One can also do exploratory analyses that were not anticipated, but again, such analyses ought to be reported as such. The idea is to make it clearer what kind of evidence confirms theories of change and what kind of evidence is more speculative or indicative of a theory of change because the evidence was only discovered after an ex post exploration of the data.
Given the time and effort required for detailed, refereed pre-registration, such as is seen for medical trials, this is unlikely to become widespread in conservation. This is likely to be a particular issue for small organisations with limited resources, where there are a large number of small projects that apply a similar approach, or where actions are time sensitive and delays caused by pre-registering would have negative impacts. A less onerous alternative pathway is for simple preregistration reporting the intent to do a study and including the hypothesis (adding X will increase Y) and the primary and secondary outcome variables. The website AsPredicted shows how the main aspects of a trial can be covered by answering a series of questions: Have any data been collected for this study already? What’s the main question being asked or the hypothesis being tested in this study? Describe the key dependent variable(s) specifying how they will be measured. Specify exactly which analyses you will conduct to examine the main question/hypothesis. How many and which conditions will be used? Describe exactly how outliers will be defined and handled, and your precise rule(s) for excluding observations. How many observations will be collected or what will determine the sample size? AsPredicted then creates a time-stamped URL that can be shared with referees and linked back to when the final results are written up. Such an approach could easily be adopted in conservation.
Another related option is project declaration, whereby collaborations are established to create repositories in which conservation projects on certain topics can be efficiently recorded pre-intervention. Providing project data to such repositories should be free, simple and quick, and should describe whether the outcomes will be monitored. By publicly sharing their plans before implementation, projects can be encouraged (for example by funders) to follow this up by adding their findings as they become available; this should help reduce the bias associated with only recording successful actions.
10.5 Value of Information: When Do We Know Enough?
Decisions often need to be made during the planning of conservation projects and ongoing management about the balance of resources and effort that are allocated to monitoring and data collection versus action on the ground (McDonald-Madden et al., 2010). Given the scale of the biodiversity crisis and the limited funding available to tackle it, it can often feel that any diversion of limited resources and funds from practical conservation action is hard to justify. However, as we have discussed in this chapter, there are many reasons why generating new knowledge is an important component of any nature conservation project that is likely to improve the ultimate outcome.
The value of information describes the benefit a decision maker would gain for additional information prior to making a decision. This is important in deciding when you should delay a decision until more information about its likely effectiveness is known and when you should act on the information you already have. For example, a project is working on the recovery of a highly endangered bird species where chick survival is low; one proposed option is to take a number of chicks into captivity to attempt a captive rearing programme before releasing them back into the wild. However, there is uncertainty around the likely success of both the rearing programme and the release of sub-adults back into the dwindling population. How important is it to reduce these uncertainties? Is it better to collect more data to understand the likely effectiveness versus the risk of delaying, during which the population is likely to decline still further (Bolam et al., 2019)? In general, collecting further information is most worthwhile when uncertainty is high, further data collection is likely to substantially reduce the uncertainty, and the gain in effectiveness is important (Canessa et al., 2015).
10.6 Writing Up and Sharing Results
10.6.1 Generating case studies
Case studies are often written by conservation practitioners at the end of a project or programme of work, to document their experiences and share lessons learned. They generally contain descriptions of the site and context, and the actions and monitoring activities carried out, followed by the results observed and lessons learned, in a narrative form. Case studies often contain substantial practical details, but rarely include any experimental component to the design. Examples of case studies could range from descriptions of how a community engagement programme has been implemented to reduce unsustainable harvesting, to describing the process of restoring a grassland site.
Case studies are often written and shared between practitioners and may be particularly useful for those working in a similar context. Drawing together a large number of case studies can also begin to allow generalities and patterns to be drawn out (e.g. a database of dam removals in Europe https://damremoval.eu/case-studies; Panorama provides an extensive database of case studies in conservation https://panorama.solutions/en). However, challenges that are frequently associated with drawing of generalities from case studies include a failure to report final outcomes, particularly for projects or components that are deemed unsuccessful, that information may be scattered across various media and formats making synthesis extremely time consuming, and variability in the consistency and scope of the data recorded (Gatt et al., 2022). Overall, case studies tend to be weak at providing evidence for the effectiveness of actions, as they lack controls or replication, and often include multiple actions carried out simultaneously or adapted over time. This means that effects cannot be clearly disentangled, and it is likely to be difficult to extrapolate results to other contexts. Case studies describing the story of a project are usually more readable than scientific papers, easier to relate to and can provide inspiration for others. They provide a useful means of describing general approaches and how problems were overcome and may also include tips for practical application. They are useful in providing the bigger picture used alongside other methods that are more effective at assessing effectiveness.
The creation of common reporting frameworks could improve consistency, reduce reporting bias and ease the tracking of the progress of multiple projects toward national and global targets (Eger et al. 2022).
10.6.2 Publishing peer-reviewed articles
Publication in a peer-reviewed journal is another option for disseminating the results of a test of a conservation action, with the benefits of verification and authentication.
Publication in this form has the additional advantage that the journal curates the report of the research, securing it for the future and guaranteeing its availability. Some journals actively seek papers written by practitioners in formats more appropriate to them (e.g. Conservation Evidence Journal, Oryx, Conservation Science and Practice, and Ecological Solutions and Evidence).
Communicating ideas and findings in a way that is both informative and interesting may require some practice. Some journals provide help with writing (e.g. Fisher, 2019, The British Ecological Society Short Guide to Scientific Writing). An additional resource is AuthorAID, a global network that provides support, mentoring, resources and training for researchers.
Box 10.1 summarises the details to include in a published article to facilitate the extraction of the results for evidence collation (e.g. Conservation Evidence, systematic review, meta-analysis).
One consideration is whether you (or your funder) would like to make the article open access, ensuring it is available for all to read online, free of charge. There is variation between journals in this regard: Conservation Evidence Journal is open access and there are no publication charges. Most other open access journals levy an article-processing charge, but in practice this may be waived or reduced, in particular for residents of countries on the Research4life eligibility list.
Here is a recommended pipeline for the writing, submission and sharing of results in a peer-reviewed journal:
- Speak to colleagues and/or search online to identify journals that could potentially be suitable for the publication of your findings. Pertinent considerations include whether a journal supports the publication of case studies, results of interventions and of negative or non-significant results. Is the journal open access (if this is your preference) and does it have an article processing charge? If an article processing charge is levied and you do not have access to funds for this purpose, are waivers/discounts of the charge available? If yes, does the process of applying for a waiver/discount appear straightforward?
- To ensure that you have selected a trusted journal, use the tools and resources at thinkchecksubmit.org.
- Use an article template for your writing. If the journal you have chosen does not provide a template, repurpose a template from one that does.
- Study the content of a few articles in your chosen journal for format and style, and for inspiration. All trusted journals provide guidelines/instructions for authors that detail their preferred format and style.
- Use a bibliography manager, such as Zotero or Mendeley, to manage citations and references.
- Include the details covered in Box 10.1 to enable results to be used in a collation of evidence (e.g. meta-analysis).
- Ensure that all figures convey their message or purpose clearly and unambiguously (see Fisher 2019, for guidance) and the data can be extracted easily. Provide detailed, self-explanatory captions for all figures and tables.
- Obtain a free ORCID iD and include it with address and affiliation details. Using this persistent unique identifier will ensures recognition for all publications.
- Full datasets can be shared in the form of supplementary material to the article, or in a data repository. Follow the 10 simple rules of Contaxis et al. (2022) to ensure your research data are discoverable.
- Ensure any co-authors have read and approved the manuscript (or better, involve them in the writing).
- Aim to optimise your text for search engines (see Fisher, 2019, for guidance), as this will improve the discoverability of your article following publication.
- Following peer review, but before acceptance, the journal is likely to call for a revision of your article: provide the revision promptly and supply a list of the changes you have made.
- Finally, publication is not the end of the story, but rather the beginning: promote the findings using additional means such as a blog, Twitter or other social media feeds, and a press release (your institution or the journal’s publisher will normally be able to help with this). A public Google Scholar profile will help in the discoverability of your article.
Not all data collected by conservation projects and organisations will be written up and published as a peer-reviewed article. Peer-review publication can be a lengthy and time-consuming process that conservationists may not have the time or enthusiasm to undertake. Instead, the priority might be to use results to produce a report that communicates project outcomes to stakeholders or other practitioners, or to inform adaptive management. These reports and case studies can still be published on organisational websites or in a practitioner-focused information clearing house like Applied Ecology Resources (https://www.britishecologicalsociety.org/applied-ecology-resources/about-aer/), a repository of conservation reports, articles, case studies, fact sheets. Applied Ecology Resources contains thousands of documents and is fully searchable, thus ensuring that reports are sharable, discoverable and permanent.
Whatever the format, data collected describing the outcomes and impact of conservation actions is likely to have immense value. Evidence describing many conservation actions across a wide range of contexts and stages in the causal chain (Figure 10.2) is still far too scarce (Christie et al., 2020a), and the value of this information will grow as more evidence is accumulated, allowing patterns of success and failure to be understood. This evidence can result in better-informed decision making and prioritisation across conservation practice and policy.
References
Bank, L., Haraszthy, L. Horváth, A et al. 2019 Nesting success and productivity of the common barn-owl: results from a nest box installation and long-term breeding monitoring program in outhern Hungary, ornis Hungarica 27, 1–31, doi:10.2478/orhu-2019-0001
Bolam, F.C., Grainger, M.J., Mengersen, K.L., et al. 2019. Using the value of information to improve conservation decision making. Biological Reviews 94: 629–647, https://doi.org/10.1111/brv.12471.
Brooke, M. D. L., Bonnaud, E., Dilley, B. J., et al. 2018. Seabird population changes following mammal eradications on islands. Animal Conservation 21: 3–12, https://doi.org/10.1111/acv.12344.
Burivalova, Z., Miteva, D., Salafsky, N., et al. 2019. Evidence types and trends in tropical forest conservation literature. Trends in Ecology & Evolution 34, 669–679, https://doi.org/10.1016/j.tree.2019.03.002.
Cadier, C., Bayraktarov, E., Piccolo, R. et al. 2020. Indicators of coastal wetlands restoration success: A systematic review. Frontiers in Marine Science 7: 600220, https://doi.org/10.3389/fmars.2020.600220.
Canessa, S., Guillera‐Arroita, G., Lahoz‐Monfort, J.J. et al. 2015. When do we need more data? A primer on calculating the value of information for applied ecologists. Methods in Ecology and Evolution 6: 1219–1228, https://doi.org/10.1111/2041-210X.12423.
Catalano A.S., Lyons-White J., Mills, M.M. et al. 2019. Learning from published project failures in conservation. Biological Conservation 238: 108223, https://doi.org/10.1016/j.biocon.2019.108223.
Christie, A. P., Amano, T., Martin, P. A., et al. 2019. Simple study designs in ecology produce inaccurate estimates of biodiversity responses. Journal of Applied Ecology 56: 2742–2754, https://doi.org/10.1111/1365-2664.13499.
Christie, A.P., Amano, T., Martin, P.A., et al. 2020a. Poor availability of context-specific evidence hampers decision-making in conservation. Biological Conservation 248: 108666, https://doi.org/10.1016/j.biocon.2020.108666.
Christie, A.P., Abecasis, D., Adjeroud, M., et al. 2020b. Quantifying and addressing the prevalence and bias of study designs in the environmental and social sciences. Nature Communications 11: 1–11. https://doi.org/10.1038/s41467-020-20142-y
Coetzee, B.W. and Gaston, K. J. 2021. An appeal for more rigorous use of counterfactual thinking in biological conservation. Conservation Science and Practice 3: e409, https://conbio.onlinelibrary.wiley.com/doi/pdf/10.1111/csp2.409.
Contaxis N, Clark J, Dellureficio A, et al. 2022. Ten simple rules for improving research data discovery. PLoS Computational Biology 18: e1009768. https://doi.org/10.1371/journal.pcbi.1009768
Crawley, M. J. 2015. Statistics: An introduction using R (2nd ed.) (Chicester: John Wiley & Sons).
Curzon, H.F. and Kontoleon, A., 2016. From ignorance to evidence? The use of programme evaluation in conservation: Evidence from a Delphi survey of conservation experts. Journal of Environmental Management 180: 466–475, https://doi.org/10.1016/j.jenvman.2016.05.062.
Davies, G. M. and Gray, A. 2015. Don’t let spurious accusations of pseudoreplication limit our ability to learn from natural experiments (and other messy kinds of ecological monitoring). Ecology and Evolution 5: 5295–5304, https://doi.org/10.1002/ece3.1782.
Eger A., Earp H., Friedman K., et al. 2022. The need, opportunities, and challenges for creating a standardized framework for marine restoration monitoring and reporting. Biological Conservation 266: 109429, https://doi.org/10.1016/j.biocon.2021.109429.
Eklund, J., Blanchet, F. G., Nyman, J., et al. 2016. Contrasting spatial and temporal trends of protected area effectiveness in mitigating deforestation in Madagascar. Biological Conservation 203: 290–97, https://doi.org/10.1016/j.biocon.2016.09.033.
European Commission (EU). 2015. The Habitats Directive. Brussels, Belgium, EU.
Ferraro, P. J. 2009. Counterfactual thinking and impact evaluation in environmental policy. In: Environmental Program and Policy Evaluation: Addressing Methodological Challenges. New Directions for Evaluation, ed. by M. Birnbaum and P. Mickwitz, 122: 75–84, https://doi.org/10.1002/ev.297.
Ferraro, P. and Agrawal, A. 2021. Synthesizing evidence in sustainability science through harmonized experiments: Community monitoring in common pool resources. Proceedings of the National Academy of Sciences 118: e2106489118. https://doi.org/10.1073/pnas.2106489118.
Ferraro P. J. and Hanauer M. M. 2014. Advances in measuring the environmental and social impacts of environmental programs. Annual Review of Environment and Resources 39: 495–517, https://www.annualreviews.org/doi/pdf/10.1146/annurev-environ-101813-013230.
Ferraro, P.J. and Miranda, J.J. 2017. Panel data designs and estimators as substitutes for randomized controlled trials in the evaluation of public programs. Journal of the Association of Environmental and Resource Economists 4: 281–317, https://www.journals.uchicago.edu/doi/10.1086/689868.
Ferraro, P. J., and Pattanayak, S. K. 2006. Money for nothing? A call for empirical evaluation of biodiversity conservation investments. PLoS Biology 4: e105, https://doi.org/10.1371/journal.pbio.0040105.
Fisher, M. 2019. Writing for Conservation. Fauna & Flora International, Cambridge, UK. https://www.oryxthejournal.org/writing-for-conservation-guide.
Fraser E.D., Dougill A.J., Mabee W.E. et al. 2006. Bottom up and top down: Analysis of participatory processes for sustainability indicator identification as a pathway to community empowerment and sustainable environmental management. Journal of Environmental Management 78: 114–127, https://doi.org/10.1016/j.jenvman.2005.04.009.
Gatt Y.M., Andradi-Brown D.A., Ahmadia G.N., et al. 2022. Quantifying the reporting, coverage and consistency of key indicators in mangrove restoration projects. Frontiers in Forests and Global Change 5: 720394, https://doi.org/10.3389/ffgc.2022.720394.
Glewwe, P. and Todd, P. 2022. Impact Evaluation in International Development: Theory, Methods and Practice. Washington, DC: World Bank. https://openknowledge.worldbank.org/handle/10986/37152 License: CC BY 3.0 IGO
Gurevitch, J., P. S. Curtis, and M. H. Jones. 2001. Meta-analysis in ecology. Advances in Ecological Research 32: 199–247, https://doi.org/10.1016/S0065-2504(01)32013-5.
Hughes, F. M. R., Adams W.M., Butchart S.H.M., et al. 2016. The challenges of integrating biodiversity and ecosystem services monitoring and evaluation at a landscape-scale wetland restoration project in the UK. Ecology and Society 21: 10, http://dx.doi.org/10.5751/ES-08616-210310.
Jellesmark, S., Ausden, M., Blackburn, T.M., et al. 2021. A counterfactual approach to measure the impact of wet grassland conservation on UK breeding bird populations. Conservation Biology 35: 1575–1585, https://doi.org/10.1111/cobi.13692.
Johnson P.N. (1994) Selection and use of nest sites by barn owls in Norfolk, England. Journal of Raptor Research, 28, 149–153.
Kurle, C. M., M. W. Cadotte, H. P., Jones, et al. 2022. Co‐designed ecological research for more effective management and conservation. Ecological Solutions and Evidence 3: e12130, https://doi.org/10.1002/2688-8319.12130.
Lahoz-Monfort, J. J., and Magrath, M. J. 2021. A comprehensive overview of technologies for species and habitat monitoring and conservation. BioScience 71: 1038–1062, https://doi.org/10.1093/biosci/biab073
Leal, M. and Spalding, M. D. (Eds.), 2022 The State of the World’s Mangroves 2022. Global Mangrove Alliance.
Legg, C.J. and Nagy, L. 2006. Why most conservation monitoring is, but need not be, a waste of time. Journal of Environmental Management 78: 194–199, https://doi.org/10.1016/j.jenvman.2005.04.016.
Lee, S.Y., Hamilton, S., Barbier, E.B. et al. 2019. Better restoration policies are needed to conserve mangrove ecosystems. Nature Ecology and Evolution 3: 870–872, https://www.nature.com/articles/s41559-019-0861-y/.
Mascia, M. B., Pailler, S., Thieme, M. L. t al. 2014. Commonalities and complementarities among approaches to conservation monitoring and evaluation. Biological Conservation 169: 258–267, https://doi.org/10.1016/j.biocon.2013.11.017.
McDonald-Madden, E., Baxter, P. W., Fuller, R. A. et al. 2010. Monitoring does not always count. Trends in Ecology & Evolution 25, 547–550, https://doi.org/10.1016/j.tree.2010.07.002.
Newell, D., 2019. A test of the use of artificial nest forms in common swift apus apus nest boxes in southern England. Conservation Evidence 16: 24–26, https://www.conservationevidence.com/reference/pdf/6956
Nosek, B.A., Ebersole, C.R., DeHaven, A.C., et al. 2019. National differences in gender–science stereotypes predict national sex differences in science and math achievement. Proceedings of the National Academy of Sciences 115: 2600–2606. https://doi.org/10.1073/pnas.0809921106.
Ockendon, N., Amano, T., Cadotte, M. et al. W.J., 2021. Effectively integrating experiments into conservation practice. Ecological Solutions and Evidence 2: e12069. https://doi.org/10.1002/2688-8319.12069.
Pynegar, E., Gibbons, J., Asquith, N et al. 2021. What role should randomized control trials play in providing the evidence base for conservation? Oryx 55: 235–244. https://doi:10.1017/S0030605319000188
Rey Benayas, J.M., Newton, A. C., Diaz, A. et al. 2009. Enhancement of biodiversity and ecosystem services by ecological restoration: A meta-analysis. Science 325: 1121–1124. https://doi:10.1126/science.1172460
Roy, H.E.; Pocock, M.J.O.; Preston, C.D. et al. 2012. Understanding citizen science and environmental monitoring: final report on behalf of UK Environmental Observation Framework. Wallingford, NERC/Centre for Ecology & Hydrology.
Schleicher, J., Eklund, J., D. Barnes, M. et al. 2020 Statistical matching for conservation science. Conservation Biology 34: 538–549, https://doi.org/10.1111/cobi.13448.
Smith R.K., Dicks L.V., Mitchell R. et al. 2014 Comparative effectiveness research: the missing link in conservation. Conservation Evidence 11: 2–6, https://conservationevidencejournal.com/reference/pdf/5475.
Stewart-Oaten A., Murdoch W.W. and Parker K.R. 1986 Environmental impact assessment:’ Pseudoreplication’ in time? Ecology 67: 929–940, https://doi.org/10.2307/1939815.
Sutherland, W.J., Armstrong, D., Butchart, S.H.M. et al. 2010. Standards for documenting and monitoring bird reintroduction projects. Conservation Letters 3: 229–235, https://doi.org/10.1111/j.1755-263X.2010.00113.x.
Sutherland W.J., Robinson J.M., Aldridge D.C. et al. 2022 Creating testable questions in practical conservation: a process and 100 questions. Conservation Evidence Journal, 19, 1–7. https://doi.org/10.52201/CEJ19XIFF2753.
Tinsley-Marshall, P. Downey, H., Adum, G. et al. 2022. Funding and delivering the routine testing of management interventions to improve conservation effectiveness. Journal for Nature Conservation 67: 126184, https://doi.org/10.1016/j.jnc.2022.126184.
Vickery, J. A., et al. 2004. The role of agri-environment schemes and farm management practices in reversing the decline of farmland birds in England. Biological Conservation 119: 19–39, https://doi.org/10.1016/j.biocon.2003.06.004.
Walters, C. J., and Holling, C. S. 1990. Large-scale management experiments and learning by doing. Ecosphere 71: 2060–2068, https://doi.org/10.2307/1938620.
Wardropper, C.B., Esman, L.A., Harden, S.C. et al. 2022. Applying a ‘fail‐fast’ approach to conservation in US agriculture. Conservation Science and Practice 4: e619, https://doi.org/10.1111/csp2.619.
White, H. 2010. A contribution to current debates in impact evaluation. Evaluation 16: 153–164, https://doi.org/10.1177/1356389010361562.
Wood, K.A. 2020 Negative results provide valuable evidence for conservation. Perspectives in Ecology and Conservation 18: 235–237, https://doi.org/10.1016/j.pecon.2020.10.007.