PART II: OBTAINING, ASSESSING AND SUMMARISING EVIDENCE
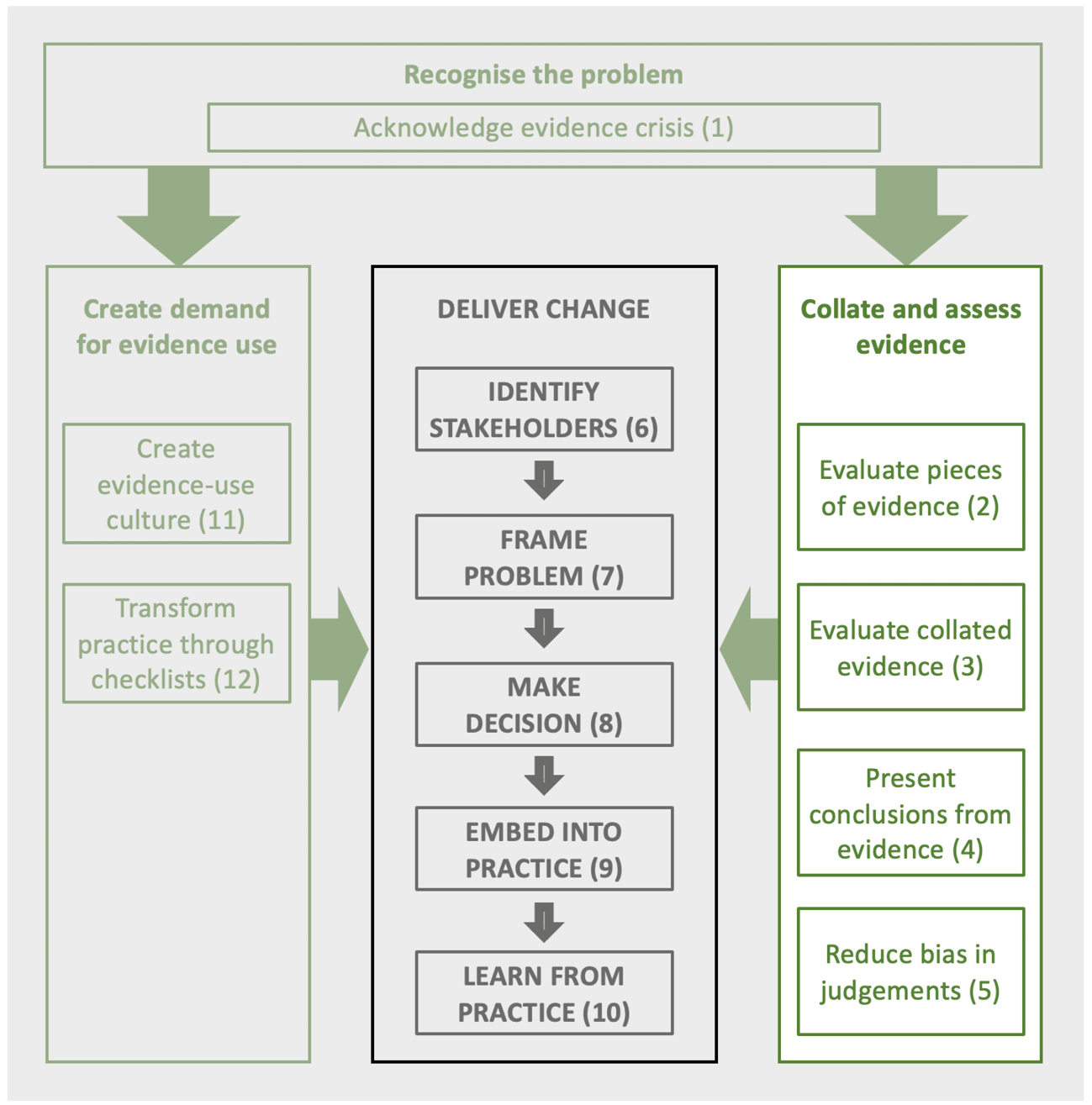
2. Gathering and Assessing Pieces of Evidence
© 2022 Chapter Authors, CC BY-NC 4.0 https://doi.org/10.11647/OBP.0321.02
Finding and assessing evidence is core to making effective decisions. The three key elements of assessing any evidence are the rigour of the information, the trust in the reliability and objectivity of the source, and the relevance to the question under consideration. Evidence may originate from a range of sources including experiments, case studies, online information, expert knowledge (including local knowledge and Indigenous ways of knowing), or citizen science. This chapter considers how these different types of evidence can be assessed.
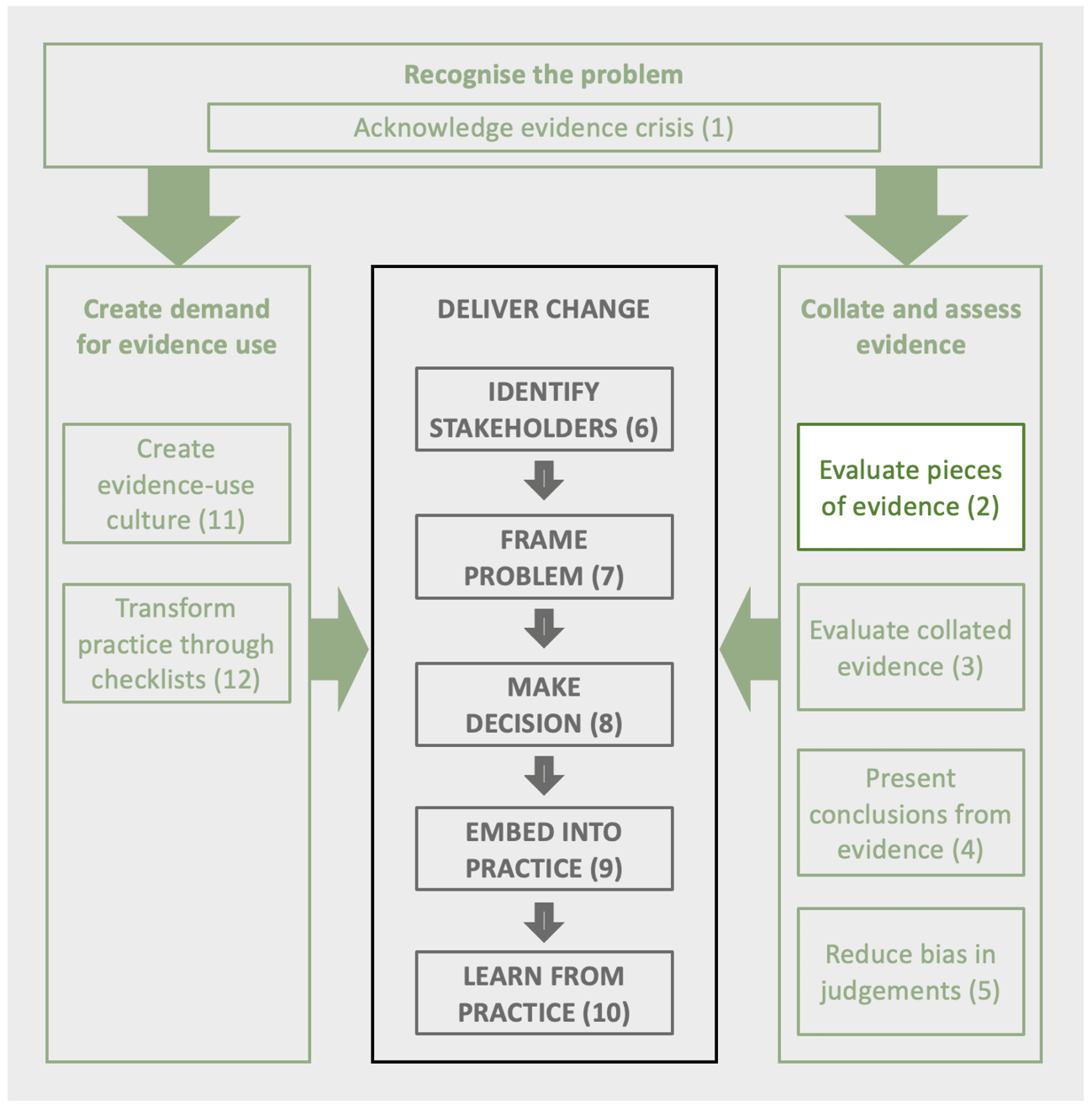
1 Conservation Science Group, Department of Zoology, University of Cambridge, The David Attenborough Building, Pembroke Street, Cambridge, UK
2 School of Biological Sciences, University of Queensland, Brisbane, 4072 Queensland, Australia
3 Centre for Biodiversity and Conservation Science, The University of Queensland, Brisbane, 4072 Queensland, Australia
4 British Trust for Ornithology, The Nunnery, Thetford, Norfolk, UK
5 Department of Geography, University of Florida, Gainesville, FL 32611, USA
6 Biosecurity Research Initiative at St Catharine’s College (BioRISC), St Catharine’s College, Cambridge, UK
7 Downing College, University of Cambridge, Regent Street, Cambridge, UK
8 School of Biosciences, University of Sheffield, Sheffield, UK
9 Ocean Discovery League, PO Box 182, Saunderstown, RI 02874, USA
10 Department of Civil Engineering, University of Asia Pacific, Dhaka 1205, Bangladesh
11 Ritsumeikan Asia Pacific University, Beppu, Oita, Japan
12 Nagoya University Graduate School of Environmental Studies, Nagoya, Aichi, Japan
2.1 What Counts as Evidence?
This book is about making more cost-effective decisions by underpinning them with evidence, defined as ‘relevant information used to assess one or more assumptions related to a question of interest’ (modified from Salafsky et al., 2019). This apparently straightforward approach easily becomes bewildering. For a decision about fisheries, the key evidence may relate to the markets, the communities, economics, legislation, fishing technologies, fish biology, and fishery models. This evidence is likely to be a mix of specific evidence applying to that community, such as the values of stakeholders or changes in fish catches, and generic evidence that applies widely, such as the size at which species start reproducing or the effectiveness of devices for reducing bycatch. The challenge is to make sense of this diverse evidence and marshal it so decisions can be made. This chapter is about assessing single pieces of evidence. The next chapter is about assessing collated evidence, such as a meta-analysis, followed by a third chapter describing the processes for converting evidence into conclusions that can then be the basis for decision making.
This process of embedding evidence into decision making is referred to by various, often interchangeable, terms. Evidence-based (e.g. Sackett et al., 2000) is a term coined in 1991 (Thomas and Eaves, 2015) that has become standard. It is generally used for the practice that aims to incorporate the best available information to guide decision making, often, but not exclusively, with an emphasis on scientific information. Evidence-informed (e.g. Nutley and Davies, 2000; Adams and Sandbrook, 2013) is similar but used to emphasise the importance of diverse types of evidence and contextual factors in decision-making, and used especially when evidence may not be central (Miles and Loughlin, 2011). Evidence-led (e.g. Sherman, 2003) is also overlapping but most often used by those stating an objective to be an evidence-led organisation to describe their aim to make evidence use central to practice.
Evidence can be embedded wherever a claim or assumption is made. For example, a project may make claims about the species present in the project site, the change in abundance of some key species, the spiritual significance of certain species, the threatening processes present, and the effectiveness of specific actions.
This chapter aims to provide a framework for considering how any piece of evidence can be evaluated and then explore what determines the reliability of different sources of evidence.
2.1.1 A taxonomy of the elements of evidence
There is a range of evidence types that serve different purposes. Table 2.1 provides a taxonomy of the key elements of different types of evidence, while Table 2.2 gives some examples of how this classification can be used.
Table 2.1 Some common distinguishing features that can be used to classify different types of evidence.
Table 2.2 Some examples of evidence and their suggested classification based on Table 2.1.
This framework provides the structure for the rest of the chapter, which, after describing a framework for assessing the weight of evidence, considers the different features of evidence, and how this framework can be applied.
2.2 A Framework for Assessing the Weight of Evidence
One of the earliest reports of framing evidence in terms of its weight comes from Greek mythology with Themis, the Greek goddess of justice, depicted carrying a pair of scales to represent the evidence for different sides of an argument. Assessing the weight of evidence is essential if the information from observations, studies or reviews are to be used to inform decisions as different pieces of evidence can vary in their strength, reliability and relevance (Gough, 2007). Such assessment is necessary even when considering formal methods, such as a meta-analysis, as it is important to evaluate the reliability of the meta-analysis, with its associated biases, and its relevance to the issue under consideration.
An approach to weighing the evidence is to consider each piece of evidence as a cuboid, as shown in Figure 2.1. The three axes of the cuboid are:
- Information reliability (I): how much the information contained within a piece of evidence can be trusted, such as the rigour of the experimental design, or whether the statement is supported by information, such as photographs.
- Source reliability (S): how much trust can be placed in the source of the evidence, such as whether it is considered authoritative, honest, competent, and does not suffer from a conflict of interest, or bias.
- Relevance (R): how closely the context in which the evidence was derived applies to the assumption being considered, such as whether it relates to a similar problem, action and situation.
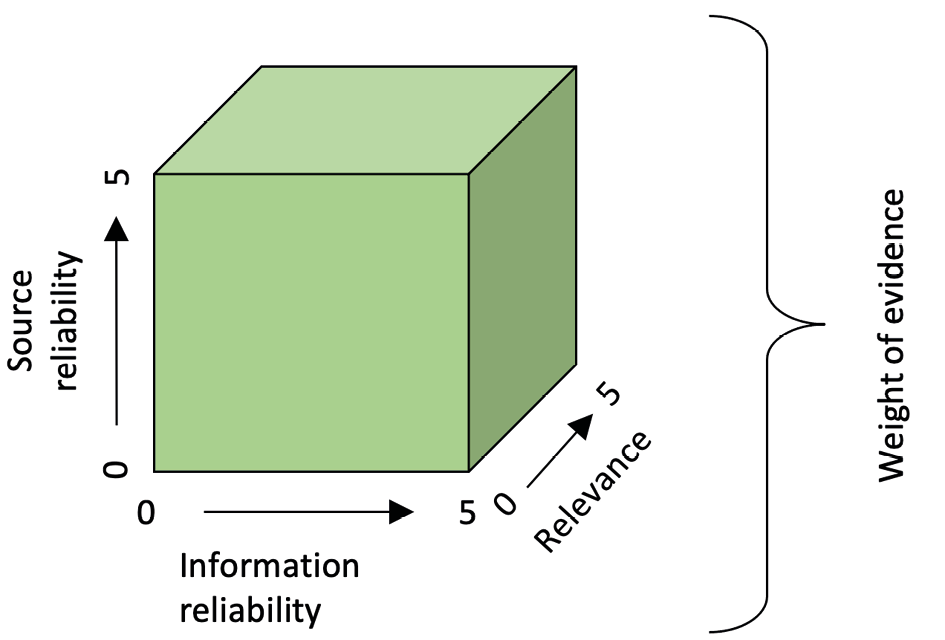
Figure 2.1 Assessing the weight of evidence according to information reliability, source reliability, and relevance (ISR). (Source: Christie et al., 2022, CC-BY-4.0)
Collectively these axes can be used to assess the weight of evidence with each piece of evidence given an information reliability, source reliability, and relevance (ISR) score (Christie et al., 2022). Table 2.3 gives a suggested set of criteria for assigning scores to assess the weight of evidence. If the evidence piece is completely irrelevant, or if there are considerable concerns in either the information reliability or source reliability, then the result is a score of zero and thus no total ISR weight. Conversely, evidence that has high relevance and reliability to a decision-making context would carry considerable weight. A score of two is given in each category for evidence about which little is known. For example, the claim ‘otters have been seen nearby’ provides little information to a nature reserve manager on what was seen, how reliable the observer is, or whether the observation was on the reserve or not.
Table 2.3 Criteria for classifying evidence weight scores, as shown in Figure 2.1.
Relevance (R) |
|||||
5 |
Considerable trust |
5 |
Extremely relevant |
5 |
|
4 |
Moderate trust |
4 |
Very relevant |
4 |
|
3 |
Some trust |
3 |
Relevant |
3 |
|
No knowledge of approach |
2 |
2 |
Somewhat relevant |
2 |
|
Some concerns over approach |
1 |
1 |
Not very relevant |
1 |
|
Considerable concerns over approach |
0 |
0 |
0 |
Evidence is frequently quoted but without information on the original source; these should be treated carefully. In some cases the original source of a piece of physically documented evidence may not only be unknown but, when looked for, cannot be located and even may not exist. These are sometimes referred to as ‘zombie studies’ or ‘zombie data’.
These ISR scores can be used for any piece of evidence, whether individual pieces (as described in this chapter) or collated evidence such as a meta-analysis (described in Chapter 3). The aim of the rest of this section is to consider the general principles for assessing the information reliability, source reliability, and relevance for different types of evidence.
2.2.1 Information reliability
Information reliability refers to how much confidence we have in the information contained within a piece of evidence, rather than its source. This could depend on the quality of the research design (see Section 2.6.1) or the support for a field observation (for example a statement, description, sketch, photo or DNA sample).
Questions to consider when assessing the reliability of the information provided by a piece of evidence could include:
- What is the basis for the claim?
- Are the methods used appropriate for the claim being made?
- Is the approach used likely to lead to bias?
- Is the material presented in its entirety or selectively presented?
- Is there supporting information, such as photographs or first-hand accounts?
- Are the conclusions appropriate given the information available?
Measurement error, sometimes called observational error, describes the difference between a true value and the measured value in the piece of evidence. For many types of evidence, this will be an important component of information reliability as this error can influence the precision and accuracy of evidence (see Freckleton et al., 2006).
Measurement error can be from multiple sources. For example, if we are counting the numbers of birds in a flock on an estuary, such counts are rarely exact and are estimates of the real number. Alternatively, we can exactly count the numbers of plants in a quadrat: however, any quadrat is a sample from a much larger population and there will consequently be error in our estimate. Similarly, many methods for censusing populations are indirect (e.g., scat counts, frass measurement, acoustic records or camera-trapping), so there is error translating these numbers into estimates of the size of the actual population.
The effect of measurement error will depend on whether it is random or systematic. Random errors affect the precision of an estimate (i.e. the variance from measurement to measurement of the same object), whereas systematic errors affect the accuracy of an estimate (i.e. how close the measured value is to the true value).
2.2.2 Source reliability
Source reliability refers to the reliability of the person, organisation, publication, website or social media providing information, including whether they can be considered authoritative or likely to be untrustworthy.
These scores are individual assessments and so different individuals will use different criteria. For example, for projects related to Pacific salmon conservation, stakeholders considered work reliable if the researchers had been seen to be involved in fieldwork, whereas government decision makers considered research reliable if it had been formally reviewed (Young et al., 2016).
Questions to consider when assessing the reliability of the source of evidence could include:
- Does the source have an interest in the evidence being used?
- Are the sources explicit about their positions, funding or agendas?
- What is the source of funding and could it influence outcomes?
- Is there evidence (real or perceived) of agendas or ulterior motives?
- What is the track record of the source in delivering reliable information?
- Is the source an expert in their field?
- Does the source have the appropriate experience for making this claim?
- If published, does it seem to be in a reliable unbiased publication?
- If published, was it peer reviewed?
2.2.3 Relevance to local conditions
Assessing relevance requires asking if a piece of evidence can be expected to apply to the issue being considered. As such it involves considering extrinsic factors, such as the similarities in location, climate, habitat, socio-cultural, and economic contexts. It also includes intrinsic factors about the problem or action being proposed, such as the type of action, specifics of implementation, or type of threat being considered.
Assessing the relevance of evidence is critical. When German forester practitioners were provided with some evidence-based guidance on forest management, the majority had concerns about the lack of specificity, were sceptical that the guidance would work across the board, wanted to know the location, forest type or soil type of the forest, and objected to a ‘cookbook’ approach (Gutzat and Dormann, 2020).
Salafsky and Margoluis (2022) make the important distinction between specific and generic evidence. For example if looking at the evidence for a species’ ecology, information on the typical diet may apply widely but the actual fruit trees visited are location dependent. Similarly, a species may be threatened by overexploitation at a global scale, but in the local area, that specific threat may be unimportant. Figure 2.2 shows the interlinking relationships between these two types of information when considering the evidence of effectiveness for conservation actions. General conclusions combine specific studies as described in various approaches in Chapter 3.
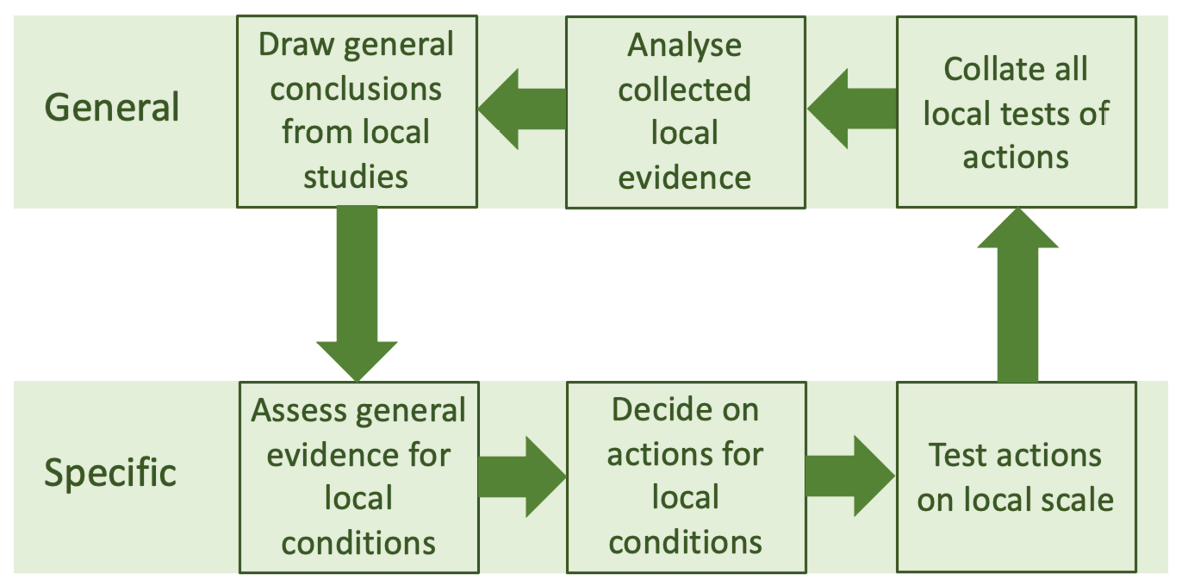
Figure 2.2 The links between general and specific information for conservation actions. Decision makers and practitioners have to interpret the general information for their specific conditions. They may generate specific evidence from their practice. The combined local studies generate general conclusions. (Source: authors)
Some questions to consider when assessing the relevance of a piece of evidence:
- Is the difference in the location and resulting differences in climate, community, etc., likely to affect the relevance?
- Do the species or ecological community differ and, if so, is this important?
- Are any sociocultural, economic, governance or regulatory variations likely to be important?
- Are there any differences in the season that could affect the relevance of the evidence?
- How much is the observed variation between the context and where the evidence was created likely to matter for the result observed? If an action is being considered, how similar is it to the action proposed?
2.2.4 Improbability of claims
Laplace’s Principle states that ‘the weight of evidence for an extraordinary claim must be proportioned to its strangeness’ (Gillispie, 1999). This was reframed and popularised by Carl Sagan who, whilst discussing extra-terrestrial life in his TV series Cosmos, proclaimed, ‘Extraordinary claims require extraordinary evidence’. Thus, a claim to have seen a common species will be readily accepted, whilst the equivalent claim to have seen a dodo would lead to calls for the observer to provide photos, videos, DNA, details of recreational drug use, etc. This improbability can be considered in terms of Bayesian statistics in which the prior belief is the likelihood of a claim or estimate, which can be updated based on additional evidence to give a posterior belief.
Improbability is an expression of the weight of evidence required to believe the claim. In many cases this is small. For example, being told that someone has reported that a tree has fallen blocking a road (i.e. an unsupported statement by a stranger) is likely as the event is unambiguous and it is hard to imagine why someone would report it inaccurately. Improbability also includes the likelihood of an alternative explanation, for example, that a rare species reported on a nature reserve is confused with a common similar species.
The factors influencing improbability include:
- How likely is it?
- Does it fall outside current knowledge?
- Does it sound plausible?
- Is there an alternative, more obvious (and parsimonious), explanation?
- Are there reasons why improbable claims might be made?
- Is this part of a pattern of claims, such as from those holding an agenda?
2.2.5 Presenting information reliability, source reliability, relevance (ISR) scores
Each piece of evidence can then be presented followed by the ISR score as assessed by the user (see Box 2.1).
2.3 Weighing the Evidence
We take all the different weighted pieces of evidence for a particular claim or assumption and balance these to arrive at a decision. Figure 2.3 shows how the evidence for an assumption can be visualised (Salafsky et al., 2019; Christie et al., 2022). The combined pieces of evidence (represented by green blocks) can fall on either side of the scales to refute (left-hand side) or support (right-hand side) and must outweigh the improbability of the assumption (the red block). This represents a situation where an assumption can either be supported or refuted in a binary manner and can be used to indicate the weight of evidence required for us to have confidence that an assumption holds.
This conceptual model helps to explain why assessing different types of evidence is so critical, which is the basis for the remainder of the chapter. If pieces of evidence are deemed highly reliable, and relevant, then there may be a strong evidence base backing up a claim or assumption. However, if there is limited evidence, or if the existing evidence has poor reliability and relevance to the context, then the evidence base may not tip the scales of the balance, and we cannot be confident in the assumption being made. Chapter 4 expands on this model to consider cases where the strength of support for the claim matters (for example there may be strong evidence for the effect of an action, or only evidence of a weak effect).
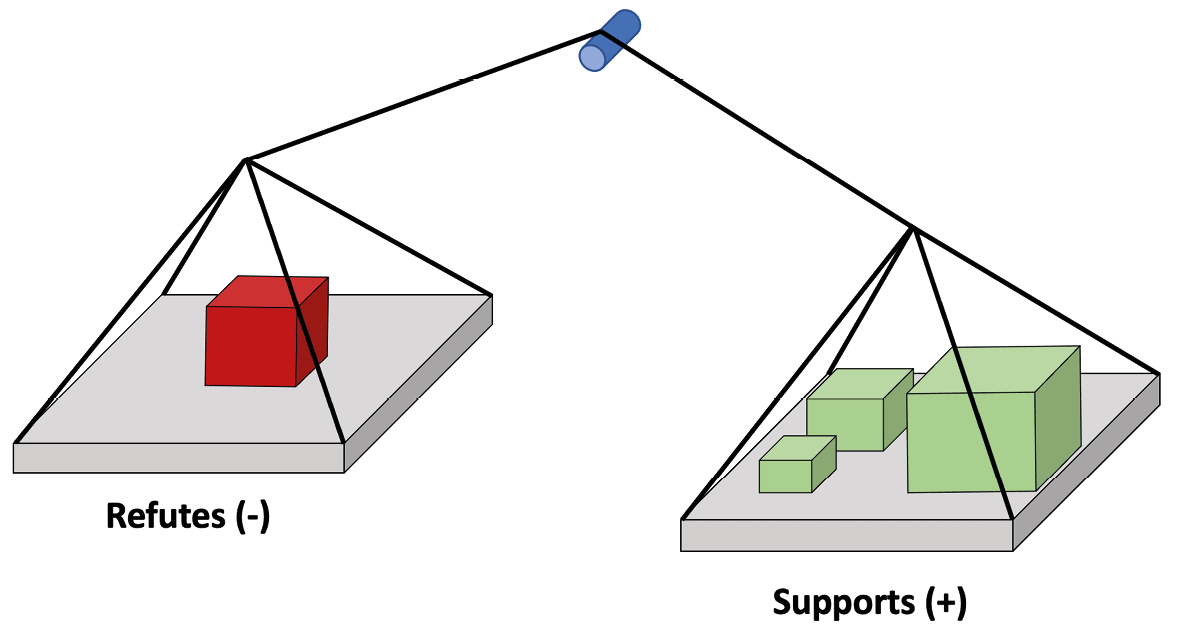
Figure 2.3 A means of visualising the evidence behind an assumption. The pieces of evidence are placed on either side of the scale, with evidence supporting the assumption falling on the right-hand side (green cuboids), and evidence refuting the assumption and the improbability (red cuboid) falling on the left-hand side. The volume of each cuboid represents its weight. The greater the tilt the higher the confidence in accepting (or rejecting) an assumption. (Source: adapted from Christie et al., 2022, following an idea of Salafsky et al., 2019, CC-BY-4.0)
2.4 Subjects of Evidence
2.4.1 Patterns or changes in status
A conservation project will often define features of interest (e.g. the focal conservation targets). These can include species, habitats, broader ecosystems or even cultural features. Thus, when designing a project an important subject of evidence is the patterns of these features and changes in that status. For example, what species are present at a site? What is the population size, and how has it changed over time? Similar questions could be asked for habitats: what is the extent and quality of habitat at the project site? How has the quality of habitat changed over time? Further details are provided in Chapter 7.
2.4.2 Patterns or changes in threats and pressures
Understanding the patterns and changes in the direct threats to biodiversity, and the indirect threats (i.e. underlying drivers) is an important subject for which evidence is required when designing a conservation project. For example, what are the key threats in a project area? What are the processes driving these threats? And how are these threats likely to change in future? Direct threats may include, but not be limited to, habitat loss and degradation, overexploitation, pollution, invasive species, or climate change. Further details are provided in Chapter 7.
2.4.3 Responses to actions
Another important subject of evidence in the design of conservation projects are the responses of features to actions that are implemented. This could be the effect of actions on biodiversity directly, the effect of actions on direct threatening processes, the effects on indirect threats (i.e. the underlying drivers of decline) or the effects of actions on other environmental and social variables (e.g. impacts on carbon storage, water flows, community health). Further details are provided in Chapter 7.
2.4.4 Financial costs and benefits
Costs are an important element of decisions, making evidence of costs an important topic. Conservationists lack the funds to implement all required interventions (Duetz et al., 2020) with Barbier (2022) calculating that nature protection may be underfunded by about $880 billion annually. This means conservationists need to prioritise actions based on their financial costs — whether that be deciding where to focus effort geographically, deciding which species and habitats to concentrate on, or which specific management action to implement. Financial costs and benefits are therefore other important types of evidence in decision making.
In this section we focus on financial and economic costs to deliver an action, but note that non-financial costs are also an important consideration in decision making; these include other environmental costs (e.g. changes in carbon storage, water quality, soil retention) and social costs (e.g. loss of access, cultural impacts).
Financial/accounting costs
Despite their importance, open and transparent information on costs is often surprisingly sparse (Iacona et al., 2018; White et al., 2022a). A study of the peer-reviewed literature of conservation actions identified that only 8.8% of studies reported the costs of the actions they were testing. Even when costs were reported there was little detail as to what types of costs were incorporated and rarely any breakdown of the constituent costs (White et al., 2022a). This detail is essential for determining the relevance of available cost estimates to a particular problem. For example, knowing that planting 1 ha of wildflower meadow in Bulgaria costs 5,000 Lev in 2021 is difficult to apply without knowing what equipment and consumables were paid for, if labour costs were included, if consultant fees were paid, or whether there were any overheads.
Table 2.4 lists the types of costs and benefits that should be considered when thinking about the costs of proposed actions. Costs can be accessed from databases of costs, project reports or budgets (although these are often not freely available) or from online catalogues of prices and cost data.
Table 2.4 Types of financial costs and benefits of conservation interventions. (Source: White et al., 2022b)
Financial benefits
Sometimes there may be financial benefits associated with a project that warrant consideration (see Table 2.4). Some conservation outcomes are easily valued, for example, there may be ecotourism revenue or the sale of sustainably harvested timber products. However, many benefits of conservation actions may be difficult to value financially (e.g. the value of ecosystem services, which are traditionally not accounted for, or seen as an externality to the economic system). It is worth considering these hidden, or unaccounted-for, benefits when assessing different conservation actions.
Economic costs and benefits
However, financial costs do not always represent the true cost of an action, and in some situations, it may be useful to estimate wider economic costs and benefits of actions. Economic costs are distinguished from accounting costs in that they represent the costs of choosing to implement a given action compared to the most likely alternative scenario, including implicit costs and benefits (e.g. opportunity costs, avoided costs), which are not seen directly on a balance sheet. For example, although the cost of setting up a protected area may include employing rangers, fencing and constructing buildings, the true cost to local communities may also include the lost agricultural income that can no longer be obtained due to the protected area placing limits on activities. Similarly, a road construction project may be installing a wildlife bridge over the road to mitigate impacts to mammal species; the cost of the bridge may be substantial, but if the bridge had not been built then the project may have been substantially delayed, or the constructor fined, due to impacts on those mammals, meaning these costs could be avoided with the construction of the bridge. Calculating economic cost would include consideration of these avoided and opportunity costs, which provide important evidence of the financial implications of a given intervention.
Distribution of costs and benefits
It is important to note that the costs and benefits of a given intervention will not be distributed evenly amongst all relevant stakeholder groups. A conservation project may be paid for by a non-governmental organisation, with financial benefits received by local government agencies, and substantial opportunity costs only felt by local communities. It is important therefore to consider who will win and lose as a result of an intervention to make sure the overall costs and benefits of the action are equitable and fair.
2.4.5 Values and norms
Decision making in conservation often requires understanding the values placed upon different scenarios, outcomes or behaviours by those stakeholders who may be influenced by the decisions. For example, the proposed reintroduction of white-tailed sea eagles to parts of the UK faced substantial opposition from farming groups, whilst gaining broad support from conservation charities. Having buy-in and involvement from local stakeholders is a vital component of ensuring conservation projects are successful, and not doing so can be a common cause of project failure (Dickson et al., 2022). Therefore, gaining evidence on the values held by different stakeholder groups, and the acceptability of different actions, is vital for designing effective and equitable conservation projects.
Just as costs and effectiveness are important for designing effective conservation actions, it is also important that decision makers consider the values placed on different outcomes, behaviours or actions by all relevant stakeholders to ensure that proposed actions are acceptable, equitable, and likely to be effective in local contexts (e.g. Gavin et al., 2018; Gregory, 2002; Whyte et al., 2016).
Values can be motivated by the want for enjoyment (e.g. a comfortable life, happiness), security (e.g. survival, health), achievement, self-direction (e.g. to experience for yourself and learn), social power (e.g. status), conformity, or for the welfare of others (Schwartz and Bilsky, 1987). Stakeholders in conservation may have values placed upon different components of the environment, desirable outcomes from a conservation project, how species and habitats should be used, cultural or religious values placed on different areas of habitats, or values on who should be implementing conservation projects. Values can either be concepts or beliefs of what is desirable.
Where it is thought that something is true, beliefs are often held by different individuals or groups. For example, a local community may highly value a particular area of habitat due to its religious significance. Understanding such beliefs, and integrating them into decision-making, is important for designing conservation projects that are likely to be successful in local contexts. These beliefs may be embedded into stories.
Where values are held widely by many individuals or groups, these can be termed social norms, which describe the shared standards of acceptable behaviour or outcomes by groups or widely by society. These can also be termed ‘collectivist’ values (Schwartz and Bilsky, 1987).
Whilst some information on values may be documented and widely available, often obtaining this information will require close consultation with different local stakeholders and communities (see Chapter 6). Such approaches can also be used to obtain evidence on status, threats, effects, and costs that may be known by different stakeholder groups.
2.5 Sources of Evidence
In the section above, we described the range of subjects of evidence that may be required when designing a conservation project. But this information can come from a range of different sources and localities. For example, evidence can be contained within peer-reviewed literature, NGO reports, online databases, or embodied within people’s knowledge and experience. Evidence can come from primary sources, secondary or tertiary sources, or where the original source of the information may not be known. Lastly, evidence can be available from sources in multiple languages.
2.5.1 Primary, secondary or unknown sources
Evidence can come from a range of localities (see below), but there are broad levels of traceability to the sources. Evidence can be from a primary source, where the evidence is available from its original locality. For example, the original research paper in which the data was published, or an original datapoint recorded by the individual who made the observation. Evidence can also be from secondary sources, which refer back to the original source of the evidence. For example, this could be in a review of many studies, in databases that reference the original source, or from experts and individuals when talking about specific studies or observations made by others. Lastly, there may be some instances where the source of evidence is unknown. This may be the case for example where unreferenced claims are made within published literature, or when experts know something based on experience but cannot pinpoint a source of the information. Unknown sources make it difficult to determine the reliability of a piece of evidence.
2.5.2 Databases
Databases are a major source of data on species and habitat status, threats, management actions, the effectiveness of actions, and the costs of actions. Stephenson and Stengel (2020) give a list of 145 conservation databases. This will typically need to be supplemented with national and local sources of documented evidence along with experience and local knowledge.
2.5.3 Peer-reviewed publications
A major source of relevant evidence to conservationists is in peer-reviewed literature, where there are a wealth of publications detailing information on species status, threats, effects of actions, as well as stakeholder values. Publications are peer-reviewed, meaning that (in theory) they are vetted by experts in the field before publication, to ensure the quality of the study and its results. Studies will be published in a range of different journals which vary in their scope and aims (e.g. what types of studies they will publish). This process helps to improve the quality of the published evidence base and is an important part of the scientific process.
Published studies vary in their quality. The main means of assessing study quality should be through evaluating the study i.e. how it was conducted and the methodology used (see Section 2.6.1 for discussion of this issue for experiments). Very good, rigorous studies can be published in little known journals and awful ones accepted in prestigious ones, but the quality of a journal is often used to give some broad indication as to the quality of the research. The following issues should however be considered when thinking about journal quality:
- Level of peer review — Does the journal have a rigorous peer-review process? For example, the rigour of the peer-review process at some journals may be poor, meaning there is less vetting of research before publication.
- Impact measures — Much is made of journal quality and especially evaluating them by measures such as impact factors (the frequency with which papers are quoted by others) although these measures have serious flaws (Kokko and Sutherland, 1999). There are also means of manipulating impact factors.
- Predatory journals — There is also the issue of predatory journals that have the appearance of others but publish with minimal assessment (as illustrated by the acceptance and publication of joke papers submitted to ridicule them). There is a list of probable predatory journals (Beall’s list, https://beallslist.net).
- Ethics — The publishing system has many ethical barriers that can disadvantage people accessing the evidence base and researchers wanting to publish. A common model is that journals charge authors to publish open-access (e.g. article processing charges, open-access fees), or charge readers fees to access articles. This creates barriers to the access of evidence and limits who can publish. A recent study found that only 5% of conservation journals met all of the Fair Open Access Principles (Veríssimo et al., 2020); principles developed to help move publishing towards sustainable, ethical practice.
- Publication delays — There can be large delays in the publishing of studies in the peer-reviewed literature due to delays with submission, and the publication process. For example, Christie et al. (2021) show that the average delay in publications providing evidence for the effectiveness of actions between the end of data collection and final publication in the literature was 3.2 years. This delay in the evidence base prevents timely access to information.
As a result of some of the issues above, there have been calls to move away from some aspects of the system. For example, Stern and O’Shea (2019) call for moves towards a publish first, curate second model of publishing where authors publish work online, peer-review happens transparently, and journals then choose which papers they wish to include. Peer Community In is an initiative that offers free, transparent peer reviews of preprints.
Databases exist to help access the scientific literature. For example, Scopus, Web of Science and Google Scholar are commonly used databases to help find relevant research literature articles based on topic or keyword searches.
2.5.4 Grey literature
Many studies of conservation actions are published in reports, conference proceedings, theses, etc. (also known as grey literature, defined as not controlled by commercial publishers), rather than as papers in academic journals. The main criticisms of grey literature are that it usually has not been peer reviewed, sometimes is not available online and even if online can sometimes be difficult to locate and search. Another is that some grey literature has a clear agenda and may be less neutral. However, there is nothing inherently unreliable about grey literature, and academic journals cannot be assumed to publish high-quality research just because they have a peer review process. There will be many high-quality pieces of evidence published in the grey literature.
Increasingly, preprint servers are hosting papers that have not yet been peer reviewed or are in the process of peer review. Although there are many models, the most common involves the preprint server simply being a repository. However, there are a growing number of preprint servers that enable readers to make comments on the documents that parallel what would happen in traditional peer review. As with any source of material, the reader must beware of variable quality (see Hoy, 2020) with potential for evidence misuse leading to trust crises, as has been observed with some preprints during the COVID-19 pandemic (Fleerachers et al., 2022). Nonetheless, such servers provide rapid access to information that may be particularly salient to conservation given that there is inherent urgency when, say, trying to recover an imperilled species (Cooke et al., 2016).
Databases also exist to help access the grey literature. Applied Ecology Resources provide a database of grey literature that can help support and improve biodiversity management (https://www.britishecologicalsociety.org/applied-ecology-resources/). The Conservation Evidence website (https://www.conservationevidence.com/) has a catalogue of open-access online reports that have been searched by them and their collaborators, now including 25 report series and identifying 278 reports that tested actions.
2.5.5 Global evidence in multiple languages
Much scientific evidence is published in languages other than English, although it is often ignored at the international level (Lynch et al., 2021). Recent research shows that up to 36% of the conservation literature is published in non-English languages (Amano et al., 2016a). Further, the number of non-English-language conservation articles published annually has been increasing over the past 39 years, at a rate similar to English-language articles (Chowdhury et al., 2022).
Ignoring scientific evidence provided in non-English-language literature could cause severe biases and gaps in our understanding of global biodiversity and its conservation. For example, using the same selection criteria as those used by Conservation Evidence (Sutherland et al., 2019), the translatE project screened 419,679 papers in 16 languages and identified 1,234 relevant papers that describe tests of conservation actions, especially in areas and for species with little or even no relevant English-language evidence (Amano et al., 2021a). Incorporating non-English-language evidence can expand the geographical coverage of English-language evidence by 12% to 25%, especially in biodiverse regions (e.g. Latin America), and taxonomic coverage by 5% to 32%, although non-English-language papers tend to adopt less robust study designs (Amano et al., 2021a). Konno et al. (2020) showed that incorporating Japanese-language studies into English-language meta-analyses caused considerable changes in the magnitude, and even direction, of overall mean effect sizes. These findings indicate that incorporating scientific literature published in non-English languages is important for synthesising global evidence in an unbiased way and deriving robust conclusions.
Finding evidence, for example in the form of literature or data, typically involves the following four stages:
- Developing search strategies
- Conducting searches
- Screening evidence based on eligibility criteria
- Extracting relevant information
Finding evidence in non-English languages could be challenging as it would require sufficient skills in the relevant languages at all stages, for example, for developing search strings (Stage 1), using language-specific search systems (Stage 2), reading full texts for screening and assessing validity (Stage 3), and extracting specific information from eligible sources (Stage 4).
One obvious solution to securing relevant language skills at all stages is to develop collaboration with native speakers of the languages, who should also be familiar with the ecology and conservation of local species and ecosystems. As conservation science has increasingly been globalised, it is now relatively easy to find experts on a specific topic who are native speakers of different languages. For example, the translatE project worked with 62 collaborators who, collectively, are native speakers of 17 languages, for screening non-English-language papers (Amano et al., 2021a). Such collaborators should be involved in as many stages of finding evidence as possible and given appropriate credit (e.g. in the form of co-authorship). In healthcare, Cochrane Task Exchange (https://taskexchange.cochrane.org/) provides an online platform where you could post requests for help with aspects of a literature review, such as screening, translation or data extraction.
The quality of machine translation (e.g. Google Translate and DeepL) has been improving, aiding some of the stages in finding evidence (e.g. for reading full texts [Stages 3 and 4], Zulfiqar et al., 2018; Steigerwald et al., 2022). However, even a few critical errors, for instance, when translating search strings (Stage 1) and extracting information (Stage 4) could have major consequences. Therefore, we still need robust tests to assess the validity of machine translation at each of the stages in finding relevant non-English-language evidence. For now, we should still try to find collaborators with relevant language skills and use machine translation with caution, i.e. only when a native speaker of the language is available for double-checking the translation output.
When developing search strategies (Stage 1), identifying appropriate sources of non-English-language evidence (e.g. bibliographic databases) is key, as few international sources index non-English-language evidence (Chowdhury et al., 2022). For example, non-English-language literature is searchable on Google Scholar (https://scholar.google.com) using non-English-language keywords. Searches on Google Scholar should be restricted only to pages written in the relevant language (from Settings), apart from languages where this search option is not available (Amano et al., 2016a), as otherwise Google Scholar’s algorithm is known to make non-English-language literature almost invisible (Rovira et al., 2021). Another effective approach is to use language-specific literature search systems, such as SciELO (https://scielo.org) for Spanish and Portuguese, J-STAGE (https://www.jstage.jst.go.jp) for Japanese, KoreaScience (https://www.koreascience.or.kr) for Korean, and CNKI (https://cnki.net) for simplified Chinese. Again, it is important to involve native speakers of relevant languages who are familiar with language-specific sources of evidence.
It is also recommended to seek input from native speakers of the languages when developing appropriate search strings in non-English languages (Stage 1). It is often difficult to find the most appropriate non-English translations for scientific terms (Amano et al., 2021b). For example, ‘biodiversity’ in German can be any of ‘Biodiversität’, ‘biologische Vielfalt’ and ‘Artenvielfalt’. In such a case, using all translations for the search helps make it as comprehensive as possible. When searching with species names, including common names in the relevant language in a search string can be effective; bird species names in multiple languages, for example, are available in the IOC World Bird List (Gill et al., 2022) and Avibase (2022).
The translatE project provides useful tools and databases that aid multilingual evidence searches. This includes a list of 466 peer-reviewed journals in ecology and conservation in 19 languages (translatE Project, 2020), a list of language-specific literature search systems for 13 languages (available in Table 1 in Chowdhury et al., 2022), a list of 1,234 non-English-language studies testing the effectiveness of conservation actions (available as S2 Data in Amano et al., 2021a), and more general tips for overcoming language barriers in science (Amano et al., 2021b) including resources and opportunities for non-native-English speakers (Amano, 2022).
2.5.6 Practitioner knowledge and expertise
Another important source of evidence is the knowledge held by practitioners and other stakeholders, which may not necessarily be documented in the peer-reviewed or non-peer-reviewed literature. The type of individual, or group of individuals, who could hold useful evidence will vary substantially depending on the context but could include: local communities and landowners, business owners, local and regional government, NGOs, Indigenous groups, scientists/researchers or policy makers. For example, it could be the knowledge of species and habitats held by local conservationists or landowners, or it could be the knowledge held by members of a scientific advisory board for NGOs or government agencies.
Often there is an overlap between this source of evidence, and other sources, as knowledge held by individuals can sometimes be traced back to other sources. For example, a conservationist may offer some evidence of the presence of a given species on a site. This knowledge may be from a primary observation and not documented, or it may have been learnt by the conservationist from a secondary source in the documented literature.
This source of evidence is particularly useful for local information in a conservation context where practitioners may have a detailed understanding of project sites and the status of species and habitats, threats present within them, and the suitability of sites for particular interventions. This is also an important source for gathering evidence on stakeholder values that can be used to help ensure actions taken are equitable and acceptable to different stakeholder groups.
The same groups, or individuals, who act as a source of evidence in this context, can also help judge the evidence to make better decisions. Chapter 5 expands upon this.
2.5.7 Indigenous and local knowledge
One particular source of expert knowledge and expertise is that held within Indigenous and local knowledge systems. Indigenous and local knowledge systems are dynamic bodies of integrated, holistic, social, cultural and ecological knowledge, practices and beliefs pertaining to the relationships of people and other living beings with one another and with their environments (IPBES, 2017). As described in Chapter 6, there is a wide range of reasons for using this knowledge and ethical ways of using it with protocols developed locally.
The ways of knowing for Indigenous and local knowledge systems include sense perception, reason, emotion, faith, imagination, intuition, memory and language (Berkes, 2017). Berkes discusses four layers of Indigenous and local knowledge: empirical (e.g. knowledge over animals, plants, soils and landscape); resource management (e.g. ecological, medicinal, scientific, and technical knowledge and practice); institutions of knowledge (e.g. the process of social memory, creativity and learning); and overarching cosmologies (e.g. underpinning knowledge-holders’ understanding of the world). Under Salafsky et al.’s (2019) list of seven types of ‘evidence’ to be used in conservation, Indigenous and local knowledge is especially strong in providing ‘direct and circumstantial evidence’ (through long-term observation); ‘specific evidence’ (local information about a specific hypothesis in a particular situation); and ‘observational and experimental evidence’ (experience from long-term trial and error). Tengö et al. (2017) point out that ‘Indigenous and local knowledge systems, and the holders of such knowledge, carry insights that are complementary to science, in terms of scope and content, and also in ways of knowing and governing social-ecological systems during turbulent times and articulating alternative ways forward’.
The collation, use and sharing of evidence may involve a range of ethical issues. One infamous example is the collection of 70,000 rubber seeds from Brazil by Henry Wickham in 1876 to take to Kew Gardens for germination, which became the source of rubber plantations in South-East Asia that replaced the South American market (Musgrave and Musgrave, 2010). Another example is ‘teff’, a gluten-free cereal that is high in protein, iron and fibre, which has been cultivated in Ethiopia for more than 2,000 years. In 2003, a dozen varieties of teff seeds were sent to a Dutch agronomist through a partnership with the Ethiopian Institute of Biodiversity Conservation for research and development. Four years later, the European Patent Office granted a patent for teff flour and related products (including the Ethiopian national staple pancake, ingela) to his Dutch company. The Ethiopian government and the Convention on Biological Diversity (CBD) tried to employ the Access and Benefit Sharing (ABS) mechanism to compensate for the loss to Ethiopians, but it was unsuccessful (Andersen and Winge, 2012).
Ensuring participatory approaches are used is key to working with indigenous and local knowledge; Verschuuren et al. (2021) set out the guidance on the approach to recognising and working with Indigenous and local knowledge on biodiversity and ecosystem services. Yet some, such as Krug et al. (2020) and Reyes-Garcia et al. (2022), suggest IPBES should improve its own processes to appropriately engage Indigenous peoples and local communities and increase linguistic diversity in its ecosystem assessments (Lynch et al., 2021). Local initiatives such as Kūlana Noi’i in Hawai’i (https://seagrant.soest.hawaii.edu/wp-content/uploads/2021/09/Kulana-Noii-2.0_LowRes.pdf) can offer guidelines along local language and value, even though engagement will look different with different communities, as discussed in Chapter 6.
Indigenous knowledge is often embedded in Indigenous languages, many of which are threatened and going extinct (Nettle and Romaine, 2000; Maffi, 2005; Amano et al., 2014). Where appropriate, respecting knowledge sovereignty and, where mutually beneficial, there is a need for storing and incorporating Indigenous and local knowledge. There are three main approaches for accessing and using knowledge: ‘knowledge co-assessment’, ‘knowledge co-production’, and ‘knowledge co-evolution’.
Knowledge co-assessment is the process in which those involved in the decision reflect and assess the different knowledge prior to making a decision (Sutherland et al., 2014). This is the most cost-effective solution and, therefore, the one that is carried out at scale.
Knowledge co-production with local communities in which research priorities are identified by the community and the research planned collectively. There are a number of good examples — the systematic review of Zurba et al. (2022) identified 102 studies. This makes sense after an inclusive process of co-assessment to check whether the knowledge already exists and if there is the capacity for the considerable work involved.
Knowledge co-evolution includes the objectives of capacity building, empowerment, and self-determination alongside knowledge co-production (Chapman and Schott, 2020).
A new role for the scientists and environmental conservationists would be to restructure and systemise a diverse knowledge system through the eyes of stakeholders, and to work with the stakeholders to utilise it to solve local environmental issues (Satō et al., 2018). In the context of local and Indigenous knowledge, the information providers are also users of this knowledge. This context-based knowledge holds multiple perspectives that are necessary to solve local issues, through the actions in which diverse stakeholders influence each other, and is constantly evolving. The sharing of this tacit knowledge mainly occurs through social interactions, informal networking, observation and listening. Therefore, an outsider’s ability to fully understand Indigenous and local knowledge may be compromised without strong trusting relationships built over time. The transfer of this vast and diverse local and Indigenous knowledge is often intergenerational. They are passed from the elders or first people to the youth through oral storytelling, talking circles or place-based education (Ross, 2016). A couple of recent projects/studies are also holding workshops, using digital storytelling and producing a local seasonal calendar to share or transfer the Indigenous and local knowledge to youth groups (Hausknechta et al., 2021; McNamara and Westoby, 2016).
Ideally, if the targeted evidence is to be used for decisions regarding land that Indigenous Peoples still manage or have tenure rights over (Indigenous land), Indigenous governance should be respected and strengthened (e.g. Artelle et al., 2019). In such cases, Indigenous agency and leadership rule the decision making while external logistical and/or technical support should be offered instead of trying to impose a western governance or decision-making system. This is supported by the fact that Indigenous lands host a consequent proportion of global biodiversity as well as protected areas and can be efficient as protected areas (Garnett et al., 2018; IPBES, 2019). If strictly local governance is not possible or the evidence is to be collected for use elsewhere than on the landscape/seascape where the knowledge is from, then the three above-mentioned approaches to access and use local and Indigenous knowledge can be adopted.
As described in Section 2.2, evidence can be assessed using the criteria of information reliability, source reliability and relevance. This can potentially be applied to any source of information. At the same time, different communities may have different ways of assessing these axes. For example, some languages embed evidence with different words, a process called ‘evidentiality’: languages may thus have specific words for different ways of knowing (Aikhenvald, 2003).
2.5.8 Online material
For information outside some of the sources listed above it is likely that an internet search will be used to obtain further information. This is especially appropriate for straightforward facts (What is the most common frog in the region? What size in the National Park? What does this species feed on?) and where the information required is not critical to the success of the project. Such sources could include Wikipedia articles, blog posts, news articles and organisation websites. Again, the same principles for considering information and source reliability apply to information online as to other sources of evidence. For example, does it seem to be an acceptable source or linked to an authoritative source? Does it seem authoritative? Does it provide sensible material on other issues you know about? Does it seem to have an agenda? Is the topic controversial?
Possible checks include:
- Does the URL have a name related to the material provided?
- Does the mailing address look legitimate in a search engine or street views?
- Is the evidence is provided by respected sources or by commercial interests?
- Is it clear where the material comes from? Does the material link back to a primary or secondary source?
- Does it seem up to date and, if not, does that matter?
- When was the source was last updated (there are a range of ways of checking this)?
- Who was involved in creating this evidence creation and do they seem credibile?
- Which other sites link to that source — found by typing the website name as link: http://www.[WEBSITE].com?
- Is there any criticism of the source?
Although probably not a major issue for most conservation evidence at present there is an increasing quantity of entirely fake material online. In fact, some conservationists have documented and warned of the rise of ‘extinction denial’ where there is denial of the evidence documenting biodiversity loss, often in media coverage (Lees et al., 2020). This may be more common in online sources of evidence, but also pervade other information sources. At a more extreme level, powerful images and videos can be reused claiming to show something different (reverse image search can be used to identify where the image was originally presented) and apparent mass support or criticism of a statement may be created by bots.
2.6 Types of Evidence
2.6.1 Experiments and quasi-experimental studies
Research studies provide data on status, change, correlations, impacts of threats and outcomes of actions. Pimm et al. (2019) state ‘measuring resilience is essential to understand it’; this principle of measurement applies widely. A number of factors indicate the accuracy of research studies. Checklist 2.1 outlines a range of issues to consider when evaluating study quality.
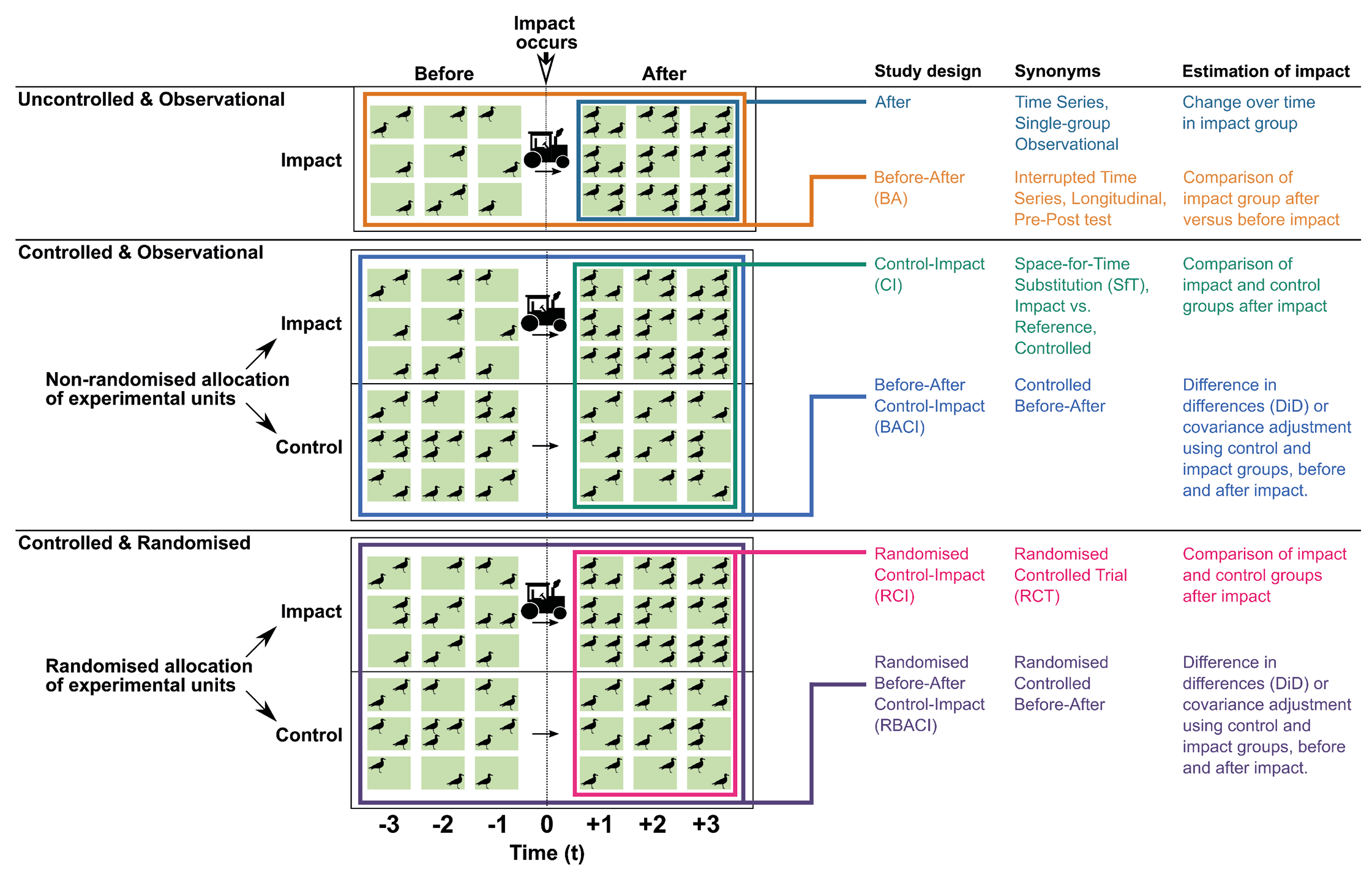
Figure 2.4 A summary of the main six broad types of study designs. Designs with an R preceding their names feature randomisation (RCI and RBACI) whilst all other designs do not. (Source: Christie et al., 2020a, CC-BY-4.0)
Figure 2.4 illustrates six broad types of study designs. There are four key aspects of study design that can improve the reliability and accuracy of results and inferences derived from them: (1) Randomisation; (2) Controls; (3) Data sampled before and after an intervention or impact has occurred; (4) Temporal and spatial replication.
Christie et al. (2019, 2020b) demonstrated that study designs that incorporate randomisation, controls, and before–after sampling (e.g. randomised control–impact [RCI or RCT] and randomised or non-randomised before–after control–impact designs [RBACI and BACI]) are typically more reliable and accurate at estimating the magnitude and direction of an impact or intervention than simpler designs that lack these features (control–impact, before–after, and after designs). In turn, control–impact and before–after designs are more accurate than after designs. Increasing replication for the more reliable, complex study designs led to increases in both accuracy and precision, but increasing replication for the simpler study designs only led to increases in precision (a more precise but still largely inaccurate figure). Accuracy can thus only be improved by changing the fundamental aspects of a study design (e.g. randomisation, controls, before–after sampling) and not simply by increasing the replication (Table 2.5).
Table 2.5 Comparison of the effectiveness of six experimental and quasi-experimental methods. These give the percentage of estimates that correctly estimated the true effect’s direction (based on the point estimate), magnitude to within 30% and direction (based on point estimate and 95% Confidence Intervals), and the increase in accuracy with greater replication (improvements in performance with increasing replication from two control and two impact sites to 50 control and 50 impact sites). (Source: Christie, 2021)
However, in practice, simpler study designs are commonly used in ecology and conservation (Christie et al., 2020a) due to a whole host of logistical, resource-based, knowledge and awareness-based constraints — for example, having the knowledge and resources to survey before and after an impact (if it is planned), the awareness and knowledge of more powerful statistical study designs, and the ability to conduct randomised experiments (due to ethical or practical barriers).
The main message is that study quality really matters and that some designs, although widely used, are unlikely to give reliable estimates of the effects of impacts and interventions. Some useful quotes to bear in mind are: ‘You can’t fix by analysis what you bungled by design…’ (Light et al., 1990), ‘Study design is to study, as foundation is to building’ (Christie, 2021). Thus it pays to use more reliable study designs whenever possible. This may not necessarily mean using randomised experiments or before-after control-impact designs (due to various constraints), but could involve using matching counterfactuals, synthetic controls, or regression discontinuity designs that are widely used in fields such as economics to evaluate policy implementations (Ferraro, 2009; Christie et al., 2020a).
In addition, any cost-based feasibility assessments of implementing study designs should carefully consider the social, environmental, and political costs of Type I and Type II errors associated with different designs (Mapstone, 1995). Researchers should always explicitly acknowledge the limitations and assumptions behind designs used and make appropriate, cautious conclusions.
2.6.2 Case studies
Case studies are a detailed examination of a particular process or situation in conservation. They are produced frequently in conservation and can provide evidence of the socio-economic context and drivers of loss in specific cases, or the implementation or outcome of a particular project or action. Case studies may be published in several sources including databases, reports or the scientific literature.
Case studies often delve into the detail of the implementation of projects, and investigate why and how outcomes occurred, specifics of the socio-economic contexts or identify challenges that hindered progress. If done well, they provide a highly relevant and detailed account of a particular situation, which can be used to help design more effective programmes in that or similar contexts. Unless they embed a test, they tend to be weaker in providing evidence for specific actions. Case studies can have considerable value in showing how projects can be created, providing potential vision, and identifying challenges and practicalities of programme implementation. The list of online material (Chapter 13) provides a range of websites giving evidence including case studies.
Case studies provide low accuracy of general effectiveness, which is better identified through stronger study designs, with baseline data, controls and replication (Christie et al., 2020a). Secondly, there is a particularly high risk of publication bias if case studies of successful projects tend to be presented but unsuccessful ones are disproportionately underreported. This can also impact the generality of findings from multiple case studies.
2.6.3 Citizen science
Citizen science (also called ‘community science’) is an umbrella term for a large variety of data collection approaches that rely on the active engagement of the general public (Haklay et al., 2021). The scope of citizen science data relevant to conservation science includes a broad suite of data types, especially direct species observations, but also acoustic recordings (Newson et al., 2015; Rowley et al., 2019), environmental DNA samples (eDNA; Buxton et al., 2018), or species records extracted from camera trap photographs (Jones et al., 2020; Swanson et al., 2016).
Long-term structured surveys, which use randomly selected sites and survey methods that are standardised over time and space, are the gold standard for robust status and change assessments. Such structured surveys require large and long-term commitments and can be costly to organise and coordinate (Schmeller et al., 2009) and therefore such monitoring programmes (Sauer et al., 2017; Greenwood et al., 1995; Lee et al., 2022; van Swaay et al., 2019; Pescott et al., 2015) have a long history of leveraging the efforts of amateur naturalists, and thus form one end of the citizen science spectrum.
However, the rapid growth in citizen science biodiversity data is predominantly the result of so-called unstructured or semi-structured projects where data entry is often facilitated through the use of digital technologies (smartphone apps, online portals), but without formal survey designs or standardised protocols, and with less stringent requirements for observer knowledge or long-term observer commitment (Pocock et al., 2017).
Such projects may have a primary goal other than population monitoring, e.g. raising awareness about focal taxa or facilitating personal record keeping for amateur naturalists, but the vast amounts of data collected in this way can potentially contribute substantially to biodiversity monitoring, particularly in parts of the world with little or no formal data collection (Amano et al., 2016b; Bayraktarov et al., 2019).
Assessing status and change from such data is challenging because of known biases in site selection, visit timing, survey effort, and/or to surveyor skill (Boersch-Supan et al., 2019; Isaac and Pocock 2015; Johnston et al., 2018, 2021, 2022). Thus there is usually a trade-off between collecting a large amount of relatively heterogeneous (i.e. lower quality) data or a smaller amount of higher quality data conforming to a defined common structure. The consequences of this quantity versus quality trade-off are an active topic of statistical research, but careful statistical accounting for observer heterogeneity and preferential sampling can turn citizen science data into a powerful tool for the sustainable monitoring of biodiversity.
There is a growing set of modelling approaches to address the challenges of unstructured data sets and/or leverage to the strengths of both structured and unstructured data sources. However, the less structured a data source is, the higher the analytical costs are in terms of strong modelling assumptions, increased model complexity, and computational demands (Fithian et al., 2015; Robinson et al., 2018; Johnston et al., 2021). Furthermore, validating complex statistical models for citizen science remains a challenge given independent validation data are often lacking.
Despite ongoing statistical developments it is therefore crucial to recognise that improvements in data quality often have much greater benefits for robust inferences than increases in data quantity (Gorleri et al., 2021). For existing citizen science data sets, it is crucial to appraise likely sources of bias and error (Dobson et al., 2020) and whether or not the data contain information that allows these biases to be accounted for. Sampling quality will be determined by factors such as observer expertise, observer motivation, spatial and temporal sampling structure (randomness of site and sampling time selection, evenness of coverage across space and/or relevant to ecological gradients) and the sample size, whereas attempts to account for bias require metadata on observer identity, effort measures, the precision of the location and time metadata, and possibly other predictors that may inform reliability of the records. For data sources with heterogeneous metadata availability and/or very uneven to sampling across space and time, stringent quality control or filtering before analysis can greatly improve the quality of inferences (Johnston et al., 2021; Gorleri et al., 2021). For ongoing schemes, it is important to add relevant metadata during data collection into the scheme design, and to educate scheme participants about the value of specific metadata, e.g. by encouraging the collection of presence-absence data or complete lists over presence-only data, or steering observer efforts to achieve more balanced sampling across space and time (Callaghan et al., 2019).
2.6.4 Statements, observations and conclusions
Much of the evidence used in decision making often involves statements and observations. If knowledge is assessed using the aforementioned criteria of information reliability, source reliability, and relevance, then when documenting knowledge we want to ensure that statements elaborate on these three criteria. Thus the claim ‘otters have been seen near the reserve’ is likely to score poorly on all these axes. The statement ‘Eric Jones, a keen local fisherman says in early spring 2022 that he watched an otter within about 10 m viewing distance for fifteen minutes, on the river just after it exits the nature reserve. He says he has seen mink regularly and he was certain it was larger’ is likely to attract a higher score. Table 2.6 lists elements to include in documenting knowledge.
The table lists the elements that can underpin most statements. The more comprehensive the statement is, the more reliable it is likely to be considered. Relevance then needs to be considered case by case in relation to the context and the claim being assessed. The aim is not to show the statement is true but to specify the evidence so that it can be assessed by others.
The acceptance of such information depends on a range of elements, such as those outlined in Table 2.6. One objective is to encourage the creation of statements that can be evaluated and be more likely to be accepted.
Table 2.6 A classification, with examples, of the elements of most statements.
What is the origin of the claim
- Own work (I have seen… My study showed…)
- Experienced (I read Smith’s paper, which showed… Marie told me she had seen…)
- Reported (Carlos said Smith’s paper said… Erica said Marie had seen…)
- Unattributed (I heard a study had shown… I heard people had seen…)
Why should this be believed
Where did the claim come from
- Published
- Grey
- Own experience
- Verbal
- Cultural
Knowledge type
What sort of information is this
- Experiment
- Documented
- Observation
- Stories
Verification
Anything that establishes its veracity
- Peer reviewed
- Verified through a process of critical appraisal of the research
- Verified by named expert
- Object, such as sample or photo, provided
- Acceptance by the community
- Status within the community and how other community members see it — e.g. Matauranga framework
Substance
For example
- Status
- Change
- Interaction
- Use
- Values
- Response
Relevance
The aim here is to consider information that would be needed to judge the relevance of the statement to another context. For example:
- Where was the evidence from?
- When was the evidence from?
- If looking at the evidence for an action, what were the specifics of the action tested?
Two examples of this process are shown below.
Shin So-jung said that she has noticed, based on monitoring water levels on the river, which she has visited weekly for over 30 years, that the sediment load was higher than she had ever seen before both along the coast and the river where our project is.
‘I have fished the lake most days for the past thirty years. After the papyrus swamp was cleared three years ago my observed fish catch is a quarter of what it was a few years ago. All other fishers state the same’. Meshack Nyongesa
2.6.5 Models
We are all familiar with the use of models in everyday decision making through the use of weather forecasts. Weather forecasts are based on the predictions of models that are complex computer simulations, fed with extensive remotely sensed data. The outputs from these models are disseminated in several ways. TV weather forecasts, for instance, make predictions of what the weather will be in the form, ‘it will be sunny tomorrow with cloudy intervals, the temperature will be 12 oC…’. Other forecasts are presented in a probabilistic way, e.g. a 20% probability of rain.
In neither case is the presentation of the output fully satisfactory. The first forecast presents the outcome without expression of uncertainty (e.g. what is the likelihood that the forecast is wrong and that it rains instead?). The second forecast expresses a probability, which seems a better expression of uncertainty. However, the stated probability will be conditional on several constituent uncertainties and the interpretation of the statement ‘20% probability of rain’ depends on these.
Whether the output of a model will be useful or not is difficult to predict. What is critically important is the purpose for which a model is to be used compared with that for which it was originally developed and being clear about the limits of the model in the predictions made.
Evaluating model quality
It may seem obvious that a poor model will generate low-quality output, however, the degree to which this is true depends on what the model is being used for. It is useful to consider four facets of model quality: biological understanding, data quality, assumptions, and quality control.
Biological understanding
For the outputs of a model to be robust, arguably they need to be based on some degree of understanding of the underlying system. The degree of model detail can vary widely, however, and the level of biological understanding required for a model to be useful depends on the situation in which the model is being used. For example, the simple model N(t+1)=rNt allows us to forecast the change in numbers from one year to the next as a function of the intrinsic rate of increase (r). This model is based on minimal biological understanding, beyond an estimate of the rate of population growth.
Although an extremely simple model such as this can potentially be useful under some circumstances (e.g. low population size, unlimited resources), they lack the ecological reality to be useful under others (e.g. large population sizes, limited resources). Consequently, models of increasing levels of sophistication have been developed, incorporating layers of ecological complexity. At the extreme, complex simulations include many details, in some cases down to the behaviour of individual organisms.
Biologically realistic models and simulations have the advantage that they better represent the true system and can be used to perform more sophisticated analyses. This is especially advantageous when we use the models to consider novel conditions or interventions. In conservation, the utility of a model is often in forecasting the likely outcome of different hypothetical options.
Complex models can have disadvantages, however. The chief limitation is that data or parameters are required to generate each component of the model. In the absence of information, it is impossible to include important details. For example, if we do not know basic information, such as the clutch size of a bird, we cannot model nesting. As the level of granularity increases, the number of parameters explodes. A second major limitation that follows from this is that parameters contain errors. These errors propagate through the model and, if there are many uncertain parameters then the outcome can be very uncertain. Alternatively, if errors are not estimated, then the model output can appear unrealistically certain.
Many useful models include no biological mechanisms whatsoever and are based on statistical analysis, basically driven by correlation. Data-driven statistical models have been used extensively in a range of applications, foremost among which are Species Distribution Models (SDMs) and Ecological Niche Models (ENMs). These are models that predict species occupancy (presence/absence) as a function of environmental and habitat variables. There are several advantages to this approach: first, it is possible to harness large amounts of existing data across many species (e.g. atlas data); second, there exists an extensive range of readily available environmental and land-use datasets; and, third, the predictions of these models can extend over regional, national or even global scales.
Of course, these models are not completely lacking in biological understanding. The choice of predictor variables will be driven by an understanding of the ecology of the species being modelled. However, there is no mechanistic modelling based on ecological or biological processes. A consequence of this is that it may not be possible to robustly predict outside of the range of conditions under which the model was fitted or to consider new variables that are not already included within the model.
Data quality
As models increase in complexity or realism, more data are required to parameterise them. The availability of data can be a major constraint in the modelling process. This is particularly a limitation on the development of models that are applied in a site-specific manner. The finer the resolution (spatial and temporal), the greater the data requirements.
If data are lacking, shortcuts can be taken in the parameterisation of models. For example, if we are modelling a particular species for which data are lacking, data may be available on similar species that can be used. This is a crude form of data imputation, the statistical methods for which are now well developed. Imputation uses the correlations between measured variables to predict values of missing data and can be used to fill in holes in datasets. Importantly, data are frequently not missing at random: for example, in conservation data are typically missing from species/populations that are low density, difficult to assess or elusive. Under such circumstances, missing data can represent a non-random subset and ignoring this could lead to biases in the dataset.
Ideally, the process of data collection and model development should be integrated: as populations are managed, monitoring will generate more data that can be used to improve the precision of models. This idea has been formalised in a couple of ways. Adaptive Management (Section 10.1) is a process of iterative improvement of decisions through feedback between interventions and models. Alternatively, the Value of Information (Section 10.5) is a quantitative measure of how much new information adds to the quality of decision making.
Assumptions
Any model is based on a set of assumptions. For a biological process-based model, these will include assumptions about the structure of the system and the various processes that drive it. Ideally, these should be clearly stated and justified with respect to previous studies and literature. Where alternative assumptions could be made, there should be a clear rationale for the decision taken.
Statistical assumptions are also important whether these are made in the model parameterisation or in the formulation of purely statistical models. All statistical methods have explicitly stated assumptions, and these can be tested through, for example, diagnostic plots. The presentation of these is important to reassure that the parameters of the models are estimated accurately. Failure of statistical assumptions requires that alternative methods of analysis are employed.
Quality control
Models are made available to end-users in a range of formats, which include software, tools (e.g. spreadsheets, web interfaces) and summaries of outputs (e.g. scientific outputs and reports). An obvious question is whether the implementation provided is reliable enough to be trusted.
The scientific peer-review process is intended to ensure that underpinning scientific logic is justified and, ideally, models will have been subjected to this peer review. The peer-review process assesses quality in terms of the assumptions and the formulation of the model. Peer review may not include rigorous testing of the model or evaluation of any implementation of the model, such as a piece of software.
If the model is presented as a piece of software, or is based on complex code, it is difficult to be sure that the model does not contain bugs or errors. Rigorous testing can reveal any issues, however this can take time. Problems can be avoided if model developers employ robust programming practices. These include unit testing of model components, and open workflows (e.g. using platforms such as GitHub) so that code can be inspected and tested. Professional programmers are familiar with such approaches, and scientific journals increasingly require such quality control for complex models.
Will a model be useful?
There are no fixed rules for judging whether a model is likely to be useful or not. A model can be very useful in one context, but hopeless in another. The key to judging whether a model is useful and if the outputs can be trusted is to have clear questions and be able to assess the degree to which the model output can address these objectives. In assessing the model, the following questions might be helpful to consider:
- What are the desired outputs of the model? For example, if we ask, ‘Will grazing increase or decrease the populations of breeding birds?’ then a simple model might be helpful. On the other hand, the answer to the question ‘by how much do we need to reduce grazing to increase breeding bird population sizes by 30%’ would require a model with sufficient sophistication to generate outputs that are numerically accurate enough.
- Has the model been validated? Ideally, a model should be validated by comparing its outputs with independent data. However, this is frequently difficult not least because models are often developed to predict novel management interventions that have not been attempted before. Nonetheless, it may be possible to compare the predictions of the model with data collected under baseline conditions. Note, it is not enough to just compare the model outputs with the input data, as this is likely to be a biased test of ‘predictive’ ability.
- Does the model output include an estimate of uncertainty? As outlined above, the parameters of a model are subject to uncertainty owing to statistical error as well as inherent temporal and spatial variability. These uncertainties propagate through the model and into the model outputs. As in the weather forecast example above, there is a need to express this uncertainty in the model predictions. If models do not include uncertainties in their predictions, there is a danger of undue faith in their outputs.
- How relevant is the model to my site? It is unlikely that a model will have been generated for any specific site that is being considered and, in any case, inter-annual variability can be considerable. If a model has been developed at another site, the relevance of the model will depend on a suite of factors including weather, habitat, soil conditions etc. In some cases, models will have been created by collating data across several studies/sites. If this is true, then an assessment will need to be made of the range of conditions under which the model outputs are valid.
- Are statistical models robust enough to make predictions? Statistical models make efficient use of data and can be used to make predictions. However, using such models requires care: do the conditions under which the data were collected remain valid? Do the predictions of the model involve extrapolation and is this valid?
- Are alternative models available and how do these compare? Sometimes there are several independent models for the same species or system. More commonly, the creators of a model will build a suite of models, typically of increasing levels of complexity from simplest to most sophisticated. Where possible these alternatives should be explored and the implications of different formulations considered.
2.6.6 Expertise
It is common for expert knowledge to be used as a form of evidence. We consider an expert to be anyone knowledgeable or well-informed about a specific context or topic. We consider expert knowledge as providing an assessment from study and accumulated experience. This thus differs from the statements described in the previous section that can be linked to specific sources and thus evaluated. Many experts have remarkable knowledge and can assess, for example, the management needed to achieve an objective.
However, care should be taken to avoid bias and inaccurate information in expert knowledge. As described in Chapter 5, experts may not be as authoritative as they seem and approaches are described that can be used to: i) elicit more reliable judgements from experts when collating evidence, ii) see if an expert makes statements that turn out to be true, and iii) check the basis of assertions. Ideally, when using expertise as a type of evidence, do not rely on a single expert, but rather collate independent advice from a diverse group of experts.
2.6.7 Online open source investigations
Another type of evidence is that from online open-source investigations, also called open source intelligence or open source research. In much of journalism the model comprises trusted anonymous sources so that the reader has to decide whether to trust both the journalist and the journalist’s unknown source. By contrast, open-source investigations comprises the transparent assessment of facts through extensive online communities. The underlying principles are to Identify, Verify and Amplify. The most famous adopter is Bellingcat, an organisation that played a key role in many security issues, including the shooting down of the Malaysian Aircraft MH17 and identifying those who carried out the Salisbury novichok poisoning (Higgins, 2022). They also actively seek to expose fake stories, images and videos and have been used for environmental issues such as identifying participants in illegal wildlife trade or illegal deforestation. The Bellingcat website provides training materials.
2.6.8 Artificial intelligence
It seems likely that in the future more evidence will be provided by artificial intelligence. As examples, literature searches are becoming increasingly automated allowing for ‘living’ reviews, species’ detection and classification algorithms are becoming increasingly accurate and the automated answering of questions is improving in quality and feasibility. Artificial intelligence does, of course, have its own range of errors and biases and is also prone to manipulation.
2.7 Acknowledgements
We thank Jonathan Campbell-James for initiating our thinking on assessing evidence and Tim Lucas for a tip leading to evidentiality.
References
Adams, W. and Sandbrook, C. 2013. Conservation, evidence and policy. Oryx 47: 329–35, https://doi.org/10.1017/S0030605312001470.
Aikhenvald, A.Y. 2003. Evidentiality in typological perspective. Typological Studies in Language 54: 1–32, https://doi.org/10.1075/tsl.54.04aik.
Amano, T. 2022. translatE. OSF, https://doi.org/10.17605/OSF.IO/Y94ZT.
Amano, T., Berdejo-Espinola, V., Christie, A.P., et al. 2021a. Tapping into non-English-language science for the conservation of global biodiversity. PLoS Biology 19: e3001296, https://doi.org/10.1371/journal.pbio.3001296.
Amano, T., González-Varo, J.P. and Sutherland, W.J. 2016a. Languages are still a major barrier to global science. PLoS Biology 14: e2000933, https://doi.org/10.1371/journal.pbio.2000933.
Amano, T., Lamming, J.D. and Sutherland, W.J. 2016b. Spatial gaps in global biodiversity information and the role of citizen science. Bioscience 66: 393–400, https://doi.org/10.1093/biosci/biw022.
Amano, T., Rios Rojas, C., Boum II, Y., et al. 2021b. Ten tips for overcoming language barriers in science. Nature Human Behaviour 5: 1119–22, https://doi.org/10.1038/s41562-021-01137-1.
Amano, T., Sandel, B., Eager, H., et al. 2014. Global distribution and drivers of language extinction risk. Proceedings of the Royal Society B. 281: 20141574, https://doi.org/10.1098/rspb.2014.1574.
Andersen, R. and Winge, T. 2012. The Access and Benefit-Sharing Agreement on Teff Genetic Resources: Facts and Lessons, FNI Report 6/2012 (Laysaker, Norway: Fridtjof Nansen Institute), https://www.fni.no/getfile.php/131843-1469869194/Filer/Publikasjoner/FNI-R0612.pdf.
Artelle, K.A., Zurba, M., Bhattacharyya, J., et al. 2019. Supporting resurgent Indigenous-led governance: A nascent mechanism for just and effective conservation. Biological Conservation 240: 108284, https://doi.org/10.1016/j.biocon.2019.10828.
Avibase. 2022. Avibase — The World Bird Database, https://avibase.bsc-eoc.org/.
Barbier, E.B. 2022. The policy implications of the Dasgupta Review: Land use change and biodiversity. Environmental and Resource Economics Special Issue, https://doi.org/10.1007/s10640-022-00658-1.
Bayraktarov, E., Ehmke, G., O’Connor, J., et al. 2019. Do big unstructured biodiversity data mean more knowledge? Frontiers in Ecology and Evolution 6: 239, https://doi.org/10.3389/fevo.2018.00239.
Berkes, F. 2017. Sacred Ecology, Fourth edition (New York: Routledge), https://doi.org/10.4324/9781315114644.
Boersch-Supan, P.H., Trask, A.E. and Baillie, S.R. 2019. Robustness of simple avian population trend models for semi-structured citizen science data is species-dependent. Biological Conservation 240: 108286, https://doi.org/10.1016/j.biocon.2019.108286.
Buxton, A., Groombridge, J. and Griffiths, R. 2018. Comparison of two citizen scientist methods for collecting pond water samples for environmental DNA studies. Citizen Science: Theory and Practice 3(2): Article 2, https://doi.org/10.5334/cstp.151.
Callaghan, C.T., Poore, A.G., Major, R.E., et al. 2019. Optimizing future biodiversity sampling by citizen scientists. Proceedings of the Royal Society B. 286: 20191487, https://doi.org/10.1098/rspb.2019.1487.
Chapman, J.M. and Schott, S. 2020. Knowledge coevolution: Generating new understanding through bridging and strengthening distinct knowledge systems and empowering local knowledge holders. Sustainability Science 15: 931–43, https://doi.org/10.1007/s11625-020-00781-2.
Chowdhury, S., Gonzalez, K., Aytekin, M.Ç.K., et al. 2022. Growth of non-English-language literature on biodiversity conservation. Conservation Biology 36, e13883, https://doi.org/10.1111/cobi.13883.
Christie, A. 2021. Determining the Biases and Consistencies in the Evidence for Conservation (PhD thesis, Cambridge: University of Cambridge), https://doi.org/10.17863/CAM.68583.
Christie, A.P., Abecasis, D., Adjeroud, M., et al. 2020a. Quantifying and addressing the prevalence and bias of study designs in the environmental and social sciences. Nature Communications 11: 6377, https://doi.org/10.1038/s41467-020-20142-y.
Christie, A.P., Amano, T., Martin, P.A., et al. 2019. Simple study designs in ecology produce inaccurate estimates of biodiversity responses. Journal of Applied Ecology 56: 2742–54, https://doi.org/10.1111/1365-2664.13499.
Christie, A.P., Amano, T., Martin, P.A., et al., 2020b. Poor availability of context-specific evidence hampers decision-making in conservation. Biological Conservation 248: 108666, https://doi.org/10.1016/j.biocon.2020.108666.
Christie, A.P., Chiaravalloti, R.M., Irvine, R., et al. 2022. Combining and assessing global and local evidence to improve decision-making. OSF, https://osf.io/wpa4t/?view_only=05e59759bba74302b52f9d8ef41c04b1.
Christie, A.P., White, T.B., Martin, P.A., et al., 2021. Reducing publication delay to improve the efficiency and impact of conservation science. PeerJ 9: e12245, https://doi.org/10.7717/peerj.12245.
Cooke, S.J., Nguyen, V.M., Wilson, A.D.M., et al. 2016. The need for speed in a crisis discipline: Perspectives on peer review duration and implications for conservation science. Endangered Species Research 30: 11–18, https://doi.org/10.3354/esr00721.
Deutz, A., Heal, G.M., Niu, R., et al. 2020. Financing Nature: Closing the Global Biodiversity Financing Gap (The Paulson Institute, The Nature Conservancy, and the Cornell Atkinson Center for Sustainability), https://www.paulsoninstitute.org/conservation/financing-nature-report/.
Dickson, I., Butchart, S.H., Catalano, A., et al. 2022. Introducing a common taxonomy to support learning from failure in conservation. Conservation Biology Accepted Author Manuscript, https://doi.org/10.1111/cobi.13967.
Dobson, A.D.M., Milner-Gulland, E.J., Aebischer, N.J., et al. 2020. Making messy data work for conservation. One Earth 2: 455–65, https://doi.org/10.1016/j.oneear.2020.04.012.
Ferraro, P.J. 2009. Counterfactual thinking and impact evaluation in environmental policy. New Directions for Evaluation Special Issue Summer 2009: 75–84, https://doi.org/10.1002/ev.297.
Fithian, W., Elith, J., Hastie, T., et al. 2015. Bias correction in species distribution models: Pooling survey and collection data for multiple species. Methods in Ecology and Evolution 6: 424–38, https://doi.org/10.1111/2041-210X.12242.
Fleerackers, A., Riedlinger, M., Moorhead, L., et al. 2022. Communicating scientific uncertainty in an age of COVID-19: An investigation into the use of preprints by digital media outlets. Health Communication 37: 726–38, https://doi.org/10.1080/10410236.2020.1864892.
Freckleton, R.P., Watkinson, A.R., Green, R.E. et al. 2006. Census error and the detection of density dependence. Journal of Animal Ecology 75: 837–851, https://doi.org/10.1111/j.1365-2656.2006.01121.x
Garnett, S.T., Burgess, N.D., Fa, J.E., et al. 2018. A spatial overview of the global importance of Indigenous lands for conservation. Nature Sustainability 1: 369–74, https://doi.org/10.1038/s41893-018-0100-6.
Gavin, M.C., McCarter, J., Berkes, F., et al. 2018. Effective biodiversity conservation requires dynamic, pluralistic, partnership-based approaches. Sustainability 10: 1846, https://doi.org/10.3390/su10061846.
Gill, F., Donsker, D. and Rasmussen, P. (eds.) 2022. IOC World Bird List (v12.1), available at https://www.worldbirdnames.org/new/.
Gillispie, C.C., Fox, R. and Gratton-Guinness, I. 1999. Pierre Simon Laplace, 1749–1827: A Life in Exact Science (Princeton, NJ: Princeton University Press).http://www.environmentalevidence.org/information-for-authors
Gough, D. 2007. Weight of evidence: A framework for the appraisal of the quality and relevance of evidence. Research Papers in Education 22: 213–28, https://doi.org/10.1080/02671520701296189.
Gorleri, F.C., Hochachka, W.M. and Areta, J.I. 2021. Distribution models using semi-structured community science data outperform unstructured-data models for a data-poor species, the Plain Tyrannulet. Ornithological Applications 123: duab038, https://doi.org/10.1093/ornithapp/duab038.
Greenwood, J.J., Baillie, S.R., Gregory, R.D., et al. 1995. Some new approaches to conservation monitoring of British breeding birds. Ibis 137: S16–S28, https://doi.org/10.1111/j.1474-919X.1995.tb08437.x.
Gregory, R.S. 2002. Incorporating value trade-offs into community-based environmental risk decisions. Environmental Values 11: 461–88, https://doi.org/10.3197/096327102129341181.
Gutzat, F. and Dormann, C. 2020. Exploration of concerns about the evidence-based guideline approach in conservation management: Hints from medical practice. Environmental Management 66: 435–49, https://doi.org/10.1007/s00267-020-01312-6.
Haklay, M., Dörler, D., Heigl, F., et al. 2021. What is citizen science? The challenges of definition. In: The Science of Citizen Science, ed. by K. Vohland, et al. (Cham, Switzerland: Springer), https://doi.org/10.1007/978-3-030-58278-4_2.
Hausknechta, S., Freeman, S., Martinc, J., et al. 2021. Sharing Indigenous knowledge through intergenerational digital storytelling: Design of a workshop engaging elders and youth, Educational Gerontology 47: 285–96, https://doi.org/10.1080/03601277.2021.1927484.
Higgins, E. 2022. We Are Bellingcat. An Intelligence Agency for the People (London: Bloomsbury).
Hoy, M.B. 2020. Rise of the Rxivs: How preprint servers are changing the publishing process. Medical Reference Services Quarterly 39: 84–89, https://doi.org/10.1080/02763869.2020.1704597.
Iacona, G.D., Sutherland, W.J. and Mappin, B., 2018. Standardized reporting of the costs of management interventions for biodiversity conservation. Conservation Biology 32: 979–88, https://doi.org/10.1111/cobi.13195.
IPBES. 2017. Annex II to decision IPBES-5/1: Approach to recognizing and working with Indigenous and local knowledge in the Intergovernmental Science-Policy Platform on Biodiversity and Ecosystem Services. April 2017, https://ipbes.net/sites/default/files/inline/files/ipbes_ilkapproach_ipbes-5-15.pdf.
IPBES. 2019. Summary for policymakers of the global assessment report on biodiversity and ecosystem services of the Intergovernmental Science-Policy Platform on Biodiversity and Ecosystem Services, ed. by. S. Díaz, et al. (Bonn, Germany; IPBES secretariat), https://doi.org/10.1111/padr.12283.
Isaac, N.J. and Pocock, M.J. 2015. Bias and information in biological records. Biological Journal of the Linnean Society 115: 522–31, https://doi.org/10.1111/bij.12532.
Jakobsson, S., Bernes, C., Bullock, J.M. et al. 2018. How does roadside vegetation management affect the diversity of vascular plants and invertebrates? A systematic review. Environmental Evidence 7: Article 17, https://doi.org/10.1186/s13750-018-0129-z.
Johnston, A., Fink, D., Hochachka, W.M., et al. 2018. Estimates of observer expertise improve species distributions from citizen science data. Methods in Ecology and Evolution 9: 88–97, https://doi.org/10.1111/2041-210X.12838.
Johnston, A., Hochachka, W.M., Strimas-Mackey, M.E., et al. 2021. Analytical guidelines to increase the value of community science data: An example using eBird data to estimate species distributions. Diversity and Distributions 27: 1265–77, https://doi.org/10.1111/ddi.13271.
Johnston, A., Matechou, E. and Dennis, E.B. 2022. Outstanding challenges and future directions for biodiversity monitoring using citizen science data. Methods in Ecology and Evolution 00: 1–14 (early view online), https://doi.org/10.1111/2041-210X.13834.
Jones, F.M., Arteta, C., Zisserman, A. et al. 2020. Processing citizen science- and machine-annotated time-lapse imagery for biologically meaningful metrics. Scientific Data 7: Article 102, https://doi.org/10.1038/s41597-020-0442-6.
Kokko, H. and Sutherland, W.J. 1999. What do impact factors tell us? Trends in Ecology and Evolution 14: 382–84, https://doi.org/10.1016/S0169-5347(99)01711-5.
Konno, K., Akasaka, M., Koshida, C., et al. 2020. Ignoring non-English-language studies may bias ecological meta-analyses. Ecological Evolution 10: 6373–84, https://doi.org/10.1002/ece3.6368.
Krug, C.B., Sterling, E. and Cadman, T. 2020. Stakeholder participation in IPBES: Connecting local environmental work with global decision making. Ecosystems and People 16: 197–211, https://doi.org/10.1080/26395916.2020.1788643.
Lee, A.T., Brooks, M. and Underhill, L.G. 2022. The SABAP2 legacy: A review of the history and use of data generated by a long-running citizen science project. South African Journal of Science 118: Article 12030, https://doi.org/10.17159/sajs.2022/12030.
Lees, A.C., Attwood, S., Barlow, J., et al. 2020. Biodiversity scientists must fight the creeping rise of extinction denial. Nature Ecology & Evolution 4: 1440–43, https://doi.org/10.1038/s41559-020-01285-z.
Light, R.J., Singer, J.D. and Willett, J.B. 1990. By Design: Planning Research on Higher Education (Cambridge, MA: Harvard University Press).
Lynch, A.J., Fernández-Llamazares, Á., Palomo, I., et al. 2021. Culturally diverse expert teams have yet to bring comprehensive linguistic diversity to intergovernmental ecosystem assessments. One Earth 4: 269–78, https://doi.org/10.1016/j.oneear.2021.01.002.
Maffi, L. 2005. Linguistic, cultural, and biological diversity. Annual Review of Anthropology 34: 599–617, https://doi.org/10.1146/annurev.anthro.34.081804.120437.
Mapstone, B.D. 1995. Scalable decision rules for environmental impact studies: Effect size, type I, and type II errors. Ecological Applications 5: 401–10, https://doi.org/10.2307/1942031.
McNamara K.E. and Westoby, R. 2016. Intergenerational sharing of Indigenous environmental knowledge in the Torres Strait. In: Geographies of Global Issues: Change and Threat. Geographies of Children and Young People, vol 8, ed. by N. Ansell, et al. (Singapore: Springer), https://doi.org/10.1007/978-981-4585-54-5_6.
Miles, A. and Loughlin, M. 2011. Models in the balance: Evidence-based medicine versus evidence-informed individualized care. Journal of Evaluation in Clinical Practice 17: 531–53, https://doi.org/10.1111/j.1365-2753.2011.01713.x.
Musgrave, W. and Musgrave, T. 2010. An Empire of Plants: People and Plants that Changed the World (London: Cassell Illustrated).
Nettle, D. and Romaine, S. 2000. Vanishing Voices: The Extinction of the World’s Languages (New York: Oxford University Press), https://vdoc.pub/download/vanishing-voices-the-extinction-of-the-worlds-languages-6n5a4e0qa5o0.
Newson, S.E., Evans, H.E. and Gillings, S. 2015. A novel citizen science approach for large-scale standardised monitoring of bat activity and distribution, evaluated in eastern England. Biological Conservation 191: 38–49, https://doi.org/10.1016/j.biocon.2015.06.009.
Nutley, S.M. and Davies, H.T.O. 2000. Getting research into practice: Making a reality of evidence based practice: Some lessons from the diffusion of innovations. Public Money and Management 20: 35–42, https://doi.org/10.1111/1467-9302.00234.
Pescott, O.L., Walker, K.J., Pocock, M.J.O., et al. 2015. Ecological monitoring with citizen science: The design and implementation of schemes for plants in Britain and Ireland. Biological Journal of the Linnean Society 115: 505–21, https://doi.org/10.1111/bij.12581.
Pimm, S.L., Donohue, I., Montoya, J.M. et al. 2019. Measuring resilience is essential to understand it. Nature Sustainability 2: 895–97, https://doi.org/10.1038/s41893-019-0399-7.
Pocock, M.J.O., Tweddle, J.C., Savage, J., et al. 2017. The diversity and evolution of ecological and environmental citizen science. PLoS ONE 12: e0172579, https://doi.org/10.1371/journal.pone.0172579.
Reyes-García, V., Fernández-Llamazares, Á., Aumeeruddy-Thomas, Y., et al. 2022. Recognizing Indigenous peoples’ and local communities’ rights and agency in the post-2020 Biodiversity Agenda. Ambio 51: 84–92, https://doi.org/10.1007/s13280-021-01561-7.
Robinson, O.J., Ruiz-Gutierrez, V. and Fink, D. 2018. Correcting for bias in distribution modelling for rare species using citizen science data. Diversity and Distributions 24: 460–72, https://doi.org/10.1111/ddi.12698.
Rovira, C., Codina, L. and Lopezosa, C. 2021. Language bias in the Google Scholar ranking algorithm. Future Internet 13: Article 31, https://doi.org/10.3390/fi13020031.
Ross, J.B. 2016. Indigenous intergenerational teachings: The transfer of culture, language, and knowledge in an intergenerational summer camp. American Indian Quarterly 40: 216–50, https://doi.org/10.5250/amerindiquar.40.3.0216.
Rowley, J.J.L., Callaghan, C.T. and Cutajar, T. 2019. FrogID: Citizen scientists provide validated biodiversity data on frogs of Australia. Herpetological Conservation and Biology 14: 155–70, http://www.herpconbio.org/Volume_14/Issue_1/Rowley_etal_2019.pdf.
Sackett, D.L., Straus, S.E., Richardson, W.S., et al. 2000. Evidence-Based Medicine: How to Practice and Teach EBM, Second edition (Edinburgh: Churchill Livingstone).
Salafsky, N., Boshoven, J., Burivalova, Z., et al. 2019. Defining and using evidence in conservation practice. Conservation Science and Practice 1: e27, https://doi.org/10.1111/csp2.27.
Salafsky, N. and Margoluis, R. 2022. Pathways to Success: Taking Conservation to Scale in Complex Systems (Washington: Island Press).
Satō T., Chabay, I. and Helgeson, J. (eds.). 2018. Transformations of Social-Ecological Systems: Studies in Co-creating Integrated Knowledge Toward Sustainable Futures (Ecological Research Monographs, Springer), https://doi.org/10.1007/978-981-13-2327-0.
Sauer, J.R., Pardieck, K.L., Ziolkowski, D.J., et al. 2017. The first 50 years of the North American Breeding Bird Survey. The Condor 119: 576–93, https://doi.org/10.1650/CONDOR-17-83.1.
Savaresi, A. 2016. Doing the right thing with traditional knowledge in international law: Lessons for the climate regime. BENELEX Working Paper No.8, Edinburgh School of Law Research Paper 2016/16, https://doi.org/10.2139/ssrn.2780332.
Schmeller, D.S., Henry, P.-Y., Julliard, R., et al. 2009. Advantages of volunteer-based biodiversity monitoring in Europe. Conservation Biology 23: 307–16, https://doi.org/10.1111/j.1523-1739.2008.01125.x.
Schwartz, S.H. and Bilsky, W. 1987. Toward a universal psychological structure of human values. Journal of Personality and Social Psychology 53: 550–62, https://doi.org/10.1037/0022-3514.53.3.550.
Sherman, L.W. 2003. Misleading evidence and evidence-led policy: Making social science more experimental. The Annals of the American Academy of Political and Social Science 589: 6–19, https://doi.org/10.1177/0002716203256266.
Steigerwald, E., Ramírez-Castañeda, V., Brandt, D.Y.C., et al. 2022. Overcoming language barriers in academia: Machine translation tools and a vision for a multilingual future. BioScience biac062,https://doi.org/10.1093/biosci/biac062.
Stephenson, P.J. and Stengel, C. 2020. An inventory of biodiversity data sources for conservation monitoring. PLoS ONE 15: e0242923, https://doi.org/10.1371/journal.pone.0242923.
Stern, B.M. and O’Shea, E.K. 2019. A proposal for the future of scientific publishing in the life sciences. PLoS Biology 17: e3000116, https://doi.org/10.1371/journal.pbio.3000116.
Sutherland, W.J., Spiegelhalter, D. and Burgman, M. 2013. Twenty tips for interpreting scientific claims. Nature 503: 335–37, https://doi.org/10.1038/503335a.
Sutherland, W.J., Gardner, T.A., Haider, J., et al. 2014. How can local and traditional knowledge be effectively incorporated into international assessments?http://journals.cambridge.org/action/displayJournal?jid=ORX 48: 1–2, https://doi.org/10.1017/S0030605313001543.
Sutherland, W.J., Taylor, N.G., MacFarlane, D., et al. 2019. Building a tool to overcome barriers in the research-implementation space: the Conservation Evidence database. Biological Conservation 238: 108199, https://doi.org/10.1016/j.biocon.2019.108199.
van Swaay, C.A., Dennis, E.B., Schmucki, R., et al. 2019. The EU Butterfly Indicator for Grassland Species: 1990–2017: Technical Report (Butterfly Conservation Europe; ABLE/eBMS), https://butterfly-monitoring.net/.
Swanson, A., Kosmala, M., Lintott, C., et al. 2016. A generalized approach for producing, quantifying, and validating citizen science data from wildlife images. Conservation Biology 30: 520–31, https://doi.org/10.1111/cobi.12695.
Tengö, M., Hill, R., Malmer, P., et al. 2017. Weaving knowledge systems in IPBES, CBD and beyond—lessons learned for sustainability. Current Opinion in Environmental Sustainability 26–27: 17–25, https://doi.org/10.1016/j.cosust.2016.12.005.
Thomas, A. and Eaves, F.F., 3rd. 2015. A brief history of evidence-based medicine (EBM) and the contributions of Dr David Sackett. Aesthetic Surgery Journal 35: NP261–63,https://doi.org/10.1093/asj/sjv130.
translatE Project. 2020. List of non-English-language journals in ecology and conservation. Version 1, https://translatesciences.com/resources/#journals.
Veríssimo, D., Pienkowski, T., Arias, M., et al. 2020. Ethical publishing in biodiversity conservation science. Conservation and Society 18: 220–25, https://www.biology.ox.ac.uk/publication/1127544/hyrax.
Verschuuren, B., Mallarach, J.M., Bernbaum, E., et al. 2021. Cultural and Spiritual Significance of Nature: Guidance for Protected and Conserved Area Governance and Management. Best Practice Protected Area Guidelines Series (Gland, Switzerland: IUCN), https://doi.org/10.2305/IUCN.CH.2021.PAG.32.en.
White, T.B, Petrovan, S.O, Booth, H., et al. 2022b. Determining the economic costs and benefits of conservation actions: A framework to support decision making. Conservation Science & Practice. https://doi.org/10.1111/csp2.12840.
White, T.B., Petrovan, S.O., Christie, A.P., et al. 2022a. What is the price of conservation? A review of the status quo and recommendations for improving cost reporting. BioScience 72: 461–71, https://doi.org/10.1093/biosci/biac007.
Whyte, K.P., Brewer, J.P. and Johnson, J.T. 2016. Weaving Indigenous science, protocols and sustainability science. Sustainability Science 11: 25–32, https://doi.org/10.1007/s11625-015-0296-6.
Young, N., Corriveau, M., Nguyen, V.M., et al. 2016. How do potential knowledge users evaluate new claims about a contested resource? Problems of power and politics in knowledge exchange and mobilization. Journal of Environmental Management 184: 380–88, https://doi.org/10.1016/j.jenvman.2016.10.006.
Zulfiqar, S., Wahab, M.F., Sarwar, M.I., et al. 2018. Is machine translation a reliable tool for reading German scientific databases and research articles? Journal of Chemical Information and Modeling 58: 2214–23, https://doi.org/10.1021/acs.jcim.8b00534.
Zurba, M., Petriello, M.A., Madge, C., et al. 2022. Learning from knowledge co-production research and practice in the twenty-first century: Global lessons and what they mean for collaborative research in Nunatsiavut. Sustainability Science 17: 449–67, https://doi.org/10.1007/s11625-021-00996-x.