5. Improving the Reliability of Judgements
© 2022 Chapter Authors, CC BY-NC 4.0 https://doi.org/10.11647/OBP.0321.05
Judgements underpin all aspects of decision making, whether assessing the nature of the problem, interpreting evidence, or deciding upon the risks and benefits of different actions. Serious problems arise with conventional means of deriving judgements to inform decisions, such as confusing values and facts, listening to a single expert, or deploying processes prone to individual and group biases such as forced consensus. Thankfully there are methods that reduce the impact of such biases including processes such as the Delphi Technique and the IDEA protocol. This chapter outlines these methods to aid in eliciting better judgements in decision making.
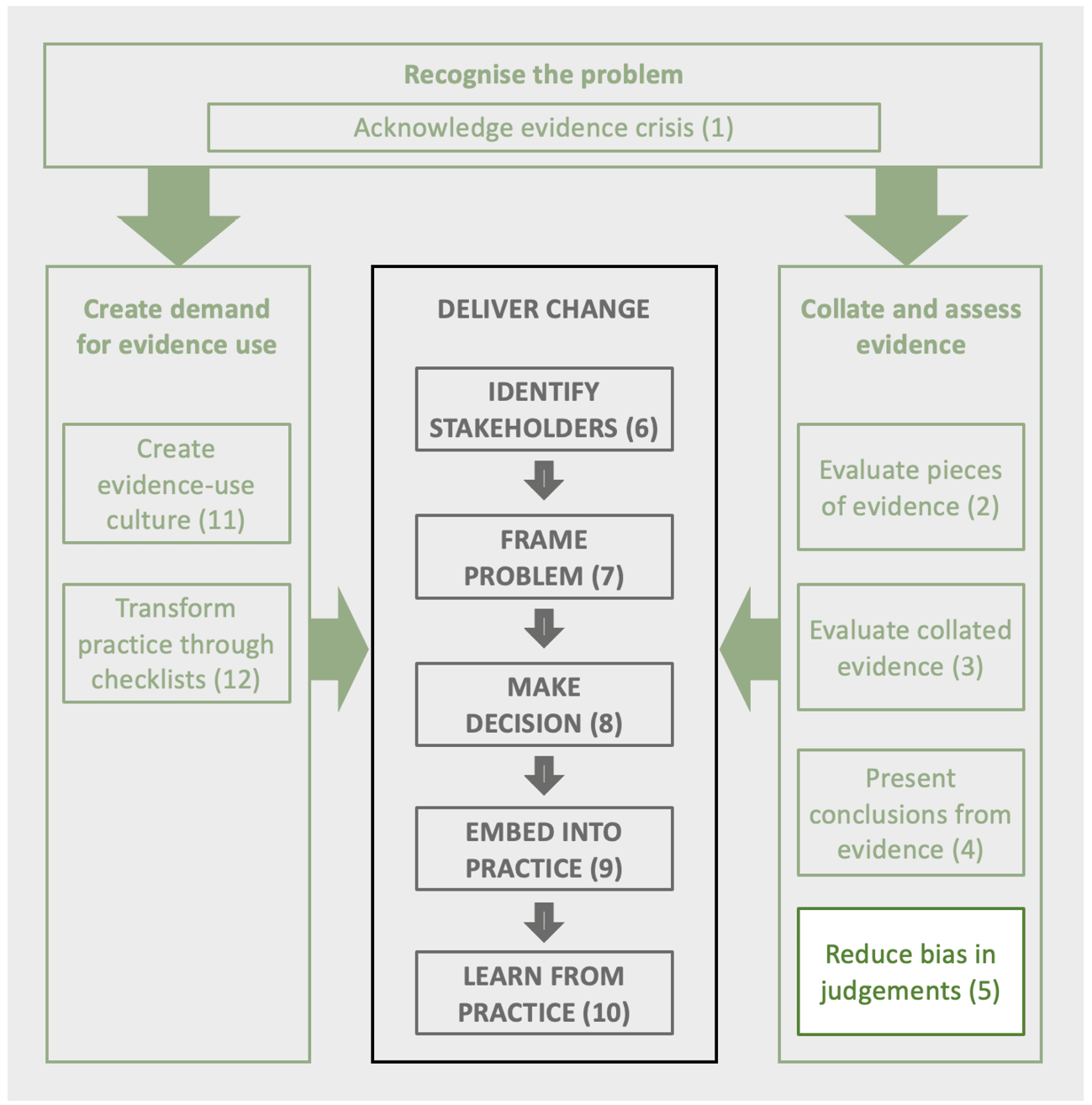
1 School of Ecosystem and Forest Sciences, University of Melbourne, Melbourne, VIC, Australia
2 College of Business, Arts and Social Sciences, Brunel University, London, UK
3 Department of Forest and Conservation Sciences, The University of British Columbia, Forest Sciences Centre, 3041–2424 Main Mall, Vancouver BC, V6T 1Z4 Canada
4 Division of Conservation Biology, Institute for Ecology and Evolution, University of Bern, Switzerland
5 Centre for Environmental Policy, Imperial College, Princes Gardens, South Kensington, London, UK
5.1 The Role of Judgements in Decision-Making
In an ideal world, many of us wish decision makers would ‘just follow the evidence’. Yet, sometimes the evidence is less than clear. As described in the previous three chapters, the evidence is often mixed in format, reliability and relevance, and often insufficient to give a confident, straightforward, definitive answer. In order to proceed with less than perfect data, decision makers routinely rely on the judgements of people, namely experts (Burgman, 2015; Martin et al., 2012; Morgan, 2014).
We take a broad definition of expertise to include anyone who has sufficient familiarity with the subject matter to be able to grasp and knowledgeably answer the question at hand. In this chapter, we take a look at the performance of experts and why some judgements and decisions go horribly wrong. We describe the main sources of cognitive biases that can hinder judgements and then describe methods that can be employed to obtain more reliable judgements. These lessons and techniques can be applied widely, whether the judgement relates to agreeing on the status of a species, predicting the likely benefits from a conservation programme, or deciding which candidate will perform best for the task.
Despite our desire for decision makers to just follow the evidence, there is no such thing as a purely ‘evidence-based’ decision. As described in Chapter 8, all decisions involve judgements about the evidence combined with judgements about values (what are we trying to achieve? what trade-offs are we willing to make?) (Gregory et al., 2012; Hemming et al., 2022). We contend that the use of experts lies in helping to estimate figures and assess facts and evidence; this is our focus. For example, the invasive nature of the cane toad has been declared a failure of experts, but it was in fact predicted and clearly articulated by Froggatt (1936). Unfortunately, their advice was ignored as political and public pressure pushed for the widespread release of the cane toad (Keogh, 2011).
5.2 When Experts Are Good (and Not so Good)
There are competing narratives about the nature of expertise. On the one hand, there is literature describing experts as demonstrating ‘elite, peak, or exceptionally high levels of performance on a particular task or within a given domain’ (Bourne Jr et al., 2014), often due to superior cognitive functioning. For example, chess experts can recognise patterns faster than novices (Bilalic et al., 2010). They have developed expertise through ‘deliberate practice’: that is, a regimen of effortful activities, usually over ten years or more, designed to optimise improvement (Ericsson et al., 1993; Charness et al., 2005). After this, experts are equipped with skills and experience that allow them to solve complex problems with greater accuracy and in a fraction of the time than novices. Not only do they store a great deal of content, but also the best pathways to access that information, which can be likened to a book’s index. For well-defined questions (e.g. physics problems), experts can select and apply appropriate mental algorithms that lead them to a solution faster (Larkin et al., 1980). Rather than working backwards from the unknowns as novices tend to do, experts identify what is known and work forwards until they have solved the desired unknown. However, though experts can show impressive knowledge within their domain, once outside their narrow area of expertise expert performance is surprisingly limited (Ericsson and Lehmann, 1996), and the delineation between expert and lay knowledge is not a sharp one (Jasanoff, 2006).
On the other hand, there are studies that paint a bleak picture of experts who are ‘not immune to the cognitive illusions that affect other people’ (Kahneman, 1991, p.144) (see also Box 5.1). In fact, Tversky and Kahneman (1971) first evidenced the ‘law of small numbers’ bias (i.e. assuming that a small sample is highly representative of the population) with survey responses from a group of mathematical psychologists.
Study after study has found expert judgements to be overconfident (McKenzie et al., 2008), and lacking in validity (Oskamp, 1965) and reliability (Trumbo et al., 1962), to name a few problems. Disastrous decisions have been linked back to flawed judgements, resulting in nuclear reactor disasters (Hollnagel and Fujita, 2013), poor military strategy (Kent, 1964), and diagnostic errors in medicine and clinical psychology (Graber, 2005). Experts are prone to the cognitive biases that befall us all (see Section 5.3). On top of this, they can also be influenced by social and political pressures.
Shanteau (1992) explained the apparent contradiction between the optimistic and pessimistic views of expertise by pointing out that the domains of expertise in each camp vary. The pessimistic story is told by researchers who have focused on judgements in domains that are more dynamic and unpredictable (e.g. behavioural economics, clinical psychology) (e.g. Dawes, 1994). The more optimistic story comes out of studies on judgement in more static, rule-based domains (e.g. physics, chess) (Anderson, 1981).
Studies in naturalistic (non-experimental) settings also provide some evidence that the pessimistic view is indeed too bleak. For example, using a sample of 19,396 observations, Johnson and Bruce (2001) found an almost perfect correlation between the subjective probability judgments of horses’ success (implicit in the bettors’ wagering activities), and the objective probability of success as determined by race outcomes. Murphy and Winkler (1977) found that weather forecasters were strikingly accurate, despite their poor reputation. Similarly, Stewart et al. (1997) found experts were better than an operational model at precipitation and temperature forecasts. One reason why these examples show such good expert performance is because the task is reasonably predictable and the experts in these environments receive constant feedback, giving them an opportunity to learn the regularities of the task (Kahneman and Klein, 2009). If a naturalistic setting is irregular (e.g. a 1-in-100-year flood occurs that year), an expert’s knowledge will not translate to the same high levels of performance.
It follows, then, that the difference between when experts appear to be good and bad is task dependent. In predictable tasks (like chess), experts perform well and can learn from deliberate practice and feedback. Unfortunately, the sorts of judgements that are made in environmental science, such as probability judgements for risk assessments, do not generally develop from deliberate practice. Forecasts, in particular, are difficult because projections — such as future disease rates — are made over relatively long time frames and are not easily validated. The best forecast can never be truly determined because the truth is unknowable at the current time. This introduces yet another challenge: it is often in these dynamic fields (e.g. emerging technologies) where conflict arises, because (a) there is much at stake, and (b) it is near impossible to prove the best judgement or even the best judge.
Problematically, attempts to select better experts by vetting them based on their professional and demographic credentials, such as speciality, years of experience, self or peer recommendation, and age, have often been in vain since these credentials are unreliable predictors of the quality of judgements provided by individuals. That is to say, studies have repeatedly shown there is typically no correlation between these attributes and the performance of experts under uncertainty (Burgman et al., 2011; Hemming et al., 2018b, 2020a; Tetlock, 2005; Tetlock and Gardner, 2016).
The less-than-perfect performance of experts when making judgements and forecasts in unpredictable environments naturally raises questions about their role in decision making, and what, if anything, can be done to improve judgements when experts are required. Despite the pessimistic outlook we’ve just painted, there is hope. A deeper dive into the track record of experts indicates many of the problems may not be with using experts per se, but rather with us using experts inappropriately (Morgan, 2014). For example, asking questions which relate to values rather than facts, giving questions with vague language (Kent, 1964; Morgan, 2014), relying on a single expert (Keogh, 2011), assuming credentials will align with more reliable expertise (Burgman et al., 2011; Shanteau et al., 2003), failing to elicit or account for uncertainty in decision making (Gregory and Keeney, 2017), failing to subject the elicitation of expert judgement to the same level of transparency and reproducibility as is required for other forms of empirical data (Drescher and Edwards, 2018; French, 2012), and ignoring or overlooking techniques that have been shown to help experts provide their best judgements under uncertainty (for example structuring group interactions).
The good news is that the judgements of experts, and the decisions that rely on them, can be improved by understanding why experts may make mistakes (biases and heuristics, Section 5.3), and by employing structured approaches that help experts to provide their best judgements under uncertainty (Section 5.5).
5.3 Blind Spots of the Human Mind
When faced with decisions, the human brain typically seeks to conserve energy by simplifying problems and making them more manageable, applying a range of mental short-cuts known as ‘heuristics’ (Simon, 1977). These short-cuts help us handle thousands of daily decisions effectively and effortlessly, but in some cases they can lead to a range of cognitive biases and poor decisions. Research on such phenomena, initiated by Amos Tversky and Daniel Kahneman in the 1970s, has highlighted a wide range of biases and situations where they can affect our judgments. This is particularly true when we face severe uncertainty, time pressure, strong emotions, and high stakes. When turning to experts to assist decisions, the risk of bias is higher when judgments are elicited in an unstructured, ad hoc way, and when reasoning and decision processes are opaque. Studies in other fields have shown experts are also prone to biases (Berthet, 2022), and there is no reason to assume conservation experts are any different.
Considerable research has investigated the impact of biases in medicine (Saposnik et al., 2016), negotiation (Caputo, 2013), computer engineering (Mohanani et al., 2018), tourist decisions (Wattanacharoensil and La-ornual, 2019), and forensic science (Cooper and Meterko, 2019). Below, we discuss a few examples of common biases that are likely to influence conservation decisions through biased expert judgments, individually or interacting with one another (additional biases can be found in Table 5.1).
Experts are often consulted for their (true or perceived) superior access to information and interpretation of it. Heuristics are typically invoked when interpreting evidence, which can lead to irrational judgements that sometimes violate probability axioms and logic. For example, we tend to give too much importance to events that are easier to recall, possibly because of personal experience or because they have occurred recently (‘availability heuristic’; Tversky and Kahneman, 1973). For example, we may overestimate the danger of flying if an airline crash has been in the news recently. We also tend to classify events or people based on preconceived classes or stereotypes (‘representativeness heuristic’; Kahneman and Tversky, 1972). As a result, we may discard general or baseline information in favour of specific details (‘base rate neglect’; Bar-Hillel, 1980), and even conclude that a specific case is more likely than a general one, which contradicts logic (‘conjunction fallacy’; Tversky and Kahneman, 1983). We also tend to place too much faith in small samples, inferring causality from extreme outcomes that are more likely to be caused by random fluctuations (‘belief in the law of small numbers’; Tversky and Kahneman, 1971). If those random fluctuations are then followed by more normal events, we might be tempted to conclude — incorrectly — that this return to normality was caused by interventions we adopted (‘regression to the mean’; Barnett et al., 2005). All these biases may be especially relevant in conservation, where samples are often small, environmental variability high, and causality difficult to infer, leading to flawed judgements in general (e.g. when interpreting time series; Fournier et al., 2019) and specific cases (e.g. when evaluating management of endangered species; Margalida et al., 2017).
Presenting and asking for information in different ways can shift people’s preferences and change the way they interpret probabilities (‘framing bias’; Tversky and Kahneman, 1985). For example, when asked about the effectiveness of a conservation action, experts give different estimates when the question is framed in terms of mortality than survival, although they should be complementary (Perneger and Agoritsas, 2011). The framing bias is compounded in judgements reflecting loss aversion, that is, our tendency to respond to losses more strongly than to gains, underpinning ‘prospect theory’ (Kahneman and Tversky, 1979), for which Kahneman won a Nobel Prize in Economics in 2002. As with the almost farcical example of estimates being influenced by watching a roulette wheel (Box 5.1), a range of other studies have shown how responses can be affected by irrelevant numbers given in background information, or unrelated information in the previous question with a tendency to anchor on such values and simply adjust estimates up or down from that point, in the direction that seems intuitive given the question (‘anchoring heuristic’; Tversky and Kahneman, 1974).
It is no surprise that we tend to interpret information in a way that is consistent with our pre-existing view of the world (‘motivated reasoning’; Kunda, 1990). For example, a geneticist and a demographer might focus on different evidence to explain a species decline. Further, we tend to seek information that confirms our beliefs, and discard information that does not (‘confirmation bias’; Nickerson, 1998). For example, reviewers who disagree with the conclusions of a scientific paper seek flaws in the methods to justify rejecting it (Mahoney, 1977). Discarding newly acquired cognitions (or pieces of knowledge) that are inconsistent with our existing cognitions helps reduce cognitive dissonance. This might happen, for example, when the results of a conservation management trial contradict prior expectations, but we are reluctant to change those expectations or do so inconsistently (Canessa et al., 2020).
It is tempting to think that we, as rational individuals, are more immune to these well-known biases than are others; studies show that people see others as more susceptible to cognitive and motivational biases than themselves (Ehrlinger et al., 2005). But awareness of these issues is only the first step in properly addressing them, and the best antidote for individual biases is groups.
Although, as discussed below, it is good practice to consult multiple experts (Sutherland and Burgman, 2015), additional social psychological biases can arise if individuals interact in an unstructured way. For example, groups tend to make more extreme and risky judgments than would individuals (‘group think’; Janis, 1982). Groups also tend to become more optimistic about the accuracy of their collective judgement (‘overconfidence’; Sniezek, 1992), particularly when faced with difficult tasks (Sniezek et al., 1990) that result in low accuracy (Puncochar and Fox, 2004). Group decisions can be led astray by charismatic, convincing or extroverted people (‘halo effects’; Thorndike, 1920) who may override the influence of those with better expertise. Kuran and Sunstein (1998) describe the problem of ‘informational cascades’ and ‘reputational cascades’ in which the group consensus is distorted. In informational cascades, early adopters of an idea or perspective can overly influence the acceptance or rejection of it as ‘fact’, with others questioning their own perspective if it is in disagreement, and sometimes failing to disclose potentially important contradictory information. In reputational cascades, people retain private beliefs that they consider correct, although against the emerging consensus, but do not reveal them in case it damages their reputation or causes hostility. Cascades are especially likely when the topic has an emotional resonance. When there is momentum behind a falsehood, it becomes easier to buy into.
Table 5.1 Some of the most common sources of bias. (Source: adapted from Mukherjee et al., 2018)
5.4 Strategies for Improving Judgements
Recognising the serious problems identified in the previous section, researchers have studied how to make the decision-making process better (more accurate, less biased, more factual, more empathetic to diversity, more rational, and fundamentally evidence-driven) (Berthet, 2022; Saposnik et al., 2016). Some simple strategies can considerably overcome biases during elicitation. The first is to restrict expert judgements to the estimation of facts, for example, estimating quantities or probabilities, rather than asking experts what to do. The second is to seek the advice of more than one expert. The third is to apply protocols to help experts provide their best judgements under uncertainty, acknowledging that experts’ beliefs are not in the form of clear probability distributions waiting to be elicited, rather, they are partially shaped by the elicitation procedure (Winkler, 1967).
Such insights can be used to explore methods for improving the quality of judgments. Most of these techniques are adapted from the human judgement literature, some of which is based on experimental results and some based on theory or known good practice generally.
5.4.1 Improving individual judgements
Although the most effective way to mitigate biases is to consult a group of people, it is not always possible to recruit multiple experts. With or without groups, there are still simple strategies that can be used to get the best out of individual experts. These suggestions are described below and summarised in Table 5.2.
Consider the opposite
Biases, such as overconfidence, can be mitigated by explicitly considering counter evidence, or reasons for the alternative view (Hoch, 1985). For example, when asking participants for judgments and their level of confidence in their answer, Koriat et al. (1980) found that calibration improved when participants were also asked specifically for contradicting reasons against their answer.
Reduce linguistic uncertainty
Language-based ambiguity, vagueness and under-specificity in elicitation problems create confusion (Regan et al., 2002) and can be reduced by carefully defining terms, specifying context and thresholds, and asking for quantitative likelihoods rather than qualitative ones (e.g. does very unlikely mean 0.1 or 0.3 probability?) (Wintle et al., 2019). See also the interpretation of terms and probabilities in Table 4.3.
Linguistic uncertainty can be problematic because it also allows for the expression of motivational biases, especially in judgements about risk. Experts — if given the space to do so through ambiguous, vague or underspecified language — may give inflated or conservative judgements of likelihood and consequence, either to be on the safe side of environmental protection, or to avoid obstacles to development. By clarifying language and context, experts will be less inclined to conflate value judgements with factual ones when answering elicitation questions.
Present evidence and questions in frequency formats
Evidence is sometimes presented as a probability, such as the likelihood that a threatened species is present although not detected in surveys. Research shows that most people find these statements easier to comprehend using frequency formats (e.g. 3 in 100) rather than probabilities (e.g. 0.03). When dealing with frequency formats, people make fewer errors of probabilistic reasoning, such as base-rate neglect (Gigerenzer et al., 2008).
Use neutral problem frames
Debiasing may also be achieved by using balanced or neutral problem frames. Williams and Mandel (2007) reframed questions to include the probability complement — for example, consider the chance of having a virus as well as the chance of not having a virus. They found that adding this extra element improved the accuracy and coherence of probability judgements. Where possible, avoid framing the decision problem in purely positive or negative terms (e.g. in terms of loss or gain), or at least, attempt a balanced presentation of information within the question or problem context.
Elicit uncertainty with free choice interval judgements
Judgments about facts and parameters are often elicited in the form of an interval (Lin and Bier, 2008). This expression of uncertainty is essential for decision makers who wish to exercise their risk attitude and base their decision on the best or worst case scenario (the uncertainty bounds), or the nominal case (best estimate) (Burgman, 2005). Interval judgements generally have an attached confidence, for example, they provide an interval that the person is 80% sure contains the true GDP of Canada. Unfortunately, overconfidence is particularly prevalent in interval judgments (Moore and Healy, 2008), with 90% intervals typically containing the answer only 50% or less of the time (Soll and Klayman, 2004). People tend to use a constant interval width over a wide range of confidence levels, leading to a high degree of overconfidence for 90% intervals, but much less for 50% intervals or for intervals without a pre-assigned degree of confidence (free choice intervals) (Teigen and Jørgensen, 2005). For this reason, we suggest allowing experts to assign their own confidence to their interval. Low confidence intervals (e.g. 50%) can later be converted (stretched) to a higher level of confidence (e.g. 80%) as required (Speirs-Bridge et al., 2010).
Elicit estimates over multiple steps
Dividing the question into multiple steps improves the chances that people will think about different kinds of evidence, and be less biased (Soll and Klayman, 2004). Instead of asking for a range of values in which the answer is thought to lie, the question can be broken into three: what is the highest plausible number of individuals, what is the lowest plausible number of individuals, and what is your best estimate of the number of individuals? This helps avoid answers that are too precise, or intervals that are too narrow and overconfident. Question order is also thought to affect such judgments: eliciting a best estimate before the bounds can lead to anchoring on the best estimate and producing overly narrow (overconfident) bounds around it, compared to when the interval is elicited first (Soll and Klayman, 2004). This approach is known as the 3-point interval elicitation method (for quantities) (Speirs-Bridge et al., 2010).
Ask the same person repeatedly for the same judgement
Herzog and Hertwig (2009) demonstrated that simply averaging two estimates of the same quantity from the same person leads to more accurate judgments than either judgement alone, by harnessing the ‘wisdom of the crowd within’. Using techniques to increase the independence of the second estimate, such as encouraging people to consider why their first one might be wrong (as above), they found an accuracy improvement of 4.1 percentage points (a medium effect size (Cohen’s d) of 0.53), which is a substantial gain when compared with 7 percentage points achieved through total independence (from averaging the estimates of two individual people).
Moreover, leaving a longer time between estimates from one individual also increases their independence, further enhancing the accuracy of the internal average (from 6% to 16% error reduction after three weeks) — similar to ‘sleeping on it’ (Vul and Pashler, 2008). Of course, improved accuracy could also be due to more evidence having been acquired in that time, but participants in Vul and Pashler’s experiment were unaware that they would be tested a second time, and the questions were such that an incidental acquisition of evidence was unlikely.
Give individual feedback
Feedback is important for learning and can improve estimation (Kopelman, 1986). A meta-analysis by Kluger and DeNisi (1996) (607 effect sizes; 23,663 observations) showed that while feedback interventions improved performance on average (d = 0.41), over one-third of the feedback interventions decreased performance. This suggests that not all types of feedback are equally effective.
Outcome feedback refers to learning the results or true value (e.g. actual length of the Nile River) and, although commonly provided, tests show it to be ineffective in improving probability forecasts (Fischer, 1982) if the information does not contribute to a pattern that can inform further answers (Benson and Önkal, 1992).
Cognitive feedback focuses on the problem solving process and is more successful (Newell et al., 2009). One effective approach is calibration feedback, which compares a person’s overall correct answers — known as percentage ‘hits’ — with their confidence levels (e.g. Lichtenstein and Fischhoff, 1980). Giving calibration feedback about people’s hit rates (i.e. asking them to compare the percentage of their intervals that captured the ‘true value’ with their levels of confidence) improved participants’ subsequent estimates of species abundance (Wintle et al., 2013).
Table 5.2 Summary of strategies for improving individual experts’ judgements.
5.4.2 Improving group judgements
Numerous studies have shown that the combined judgements of a group of individuals are usually more accurate than any given individual, even those that might be considered to be the ‘best expert’ (e.g. Burgman et al., 2011; Wintle et al., 2013; Mellers et al., 2014; Hemming et al., 2020b). Individual biases tend to cancel each other out when aggregated, leading to a wisdom of crowds effect (Surowiecki, 2004). This is particularly powerful in the group average when different group members’ judgements ‘bracket’ the true value they are attempting to estimate (Larrick and Soll, 2006). If not, groups may give precise but inaccurate judgements.
Eliciting judgements from groups introduces a whole new suite of considerations, including the problems and benefits of interaction, and group composition. The section below touches upon some important considerations and commonly used strategies to reduce bias in groups (some of these are summarised in Table 5.3).
Ask for the judgement from multiple experts
Taking the average of quantitative estimates provided by two people typically achieves better percentage accuracy improvement than choosing the estimate of the best performing judge (e.g. Soll and Larrick, 2009 [experiment 1: 17% improvement from averaging, 12.9% improvement from choosing]).
Benefits of discussion
There is good evidence that group interaction and discussion usually improves the accuracy of judgements, particularly for quantitative tasks, with group members becoming more accurate individually during group interaction (Schultze et al., 2012; Mercier and Claidière, 2022). Deliberation and social influence can also improve the crowd’s collective accuracy when structured in small, independent groups (Navajas et al., 2017). Discussion offers the potential to improve group performance by resolving misunderstanding of the question, providing opportunities for people to introduce new information and learn from each other (Mojzisch and Schulz-Hardt, 2010), encouraging critical thinking (Postmes et al., 2001), and encouraging counterfactual reasoning (Galinsky and Kray, 2003). A study with 211 teams engaged in 969 face-to-face discussions (Silver et al., 2021) found that group interaction improved group accuracy, but only when groups were already collectively well calibrated (i.e. more accurate people were more confident, and less accurate people were less confident going into discussion). Under these conditions, the most knowledgeable (and confident) people were more likely to influence the answers of the less knowledgeable people in the group.
Successful group interaction — that is, interaction that improves the performance of the group — requires ensuring that individuals do learn from the group. This means encouraging group members to share information, and avoiding ‘hidden profiles’ (where pieces of relevant information are not shared by everyone in the group) (Stasser and Titus, 2003). Several factors moderate this, including the type of task (Stasser and Stewart, 1992).
Foster dissent and argumentation
Disagreeing participants are poised to produce an often beneficial discussion, but such a discussion may not necessarily follow unless all group members are encouraged to contribute their perspectives. There are benefits of dissent within groups. Mercier and Sperber (2011) contend that reasoning in defence of an argument, to persuade another, is more rigorous than reasoning in pursuit of truth or to solve abstract problems. Benefits also come from the division of cognitive labour — it is more efficient for one person to adopt a position and gather evidence supporting that position. Schulz-Hardt et al. (2006) found that groups with dissenting members (pre-discussion) made better decisions than homogenous groups.
When pre-existing dissent is lacking, it may need to be artificially fostered using structured methods. Two specific approaches have been explored for stimulating disagreement within a group, which could also be useful for groups of experts: Devil’s Advocacy (DA) and Dialectical Inquiry (DI) (reviewed by Katzenstein, 1996). Dialectical Inquiry involves the development of two plans that rest on a conflicting set of assumptions. Each side then engages in a debate about the relative merits of each. In Devil’s Advocacy, the prevailing plan is criticised by the devil’s advocate, but no alternative plan is proposed. In a meta-analysis of five studies that investigated the effects of DA and DI, Schwenk (1990) found that DA leads to superior decision making, over an expert-based approach (n = 432, effect sizes ranging from d = 0.64 to 0.13), but the effect of DI over the expert-based approach was smaller and not statistically significant (n = 393, average d = 0.16, confidence interval included zero: -0.10 to 0.42).
Critical and counterfactual thinking, not consensus
Postmes et al. (2001) primed ‘consensus’ groups and ‘critical’ groups by participating in different lead up tasks (collaborating in producing a poster and reaching a consensus about the design, or discussing differences of opinion about a new tax law). The experimental task itself involved selecting a job candidate, where there was an optimal solution to be uncovered via information sharing, to reduce hidden profiles. In both consensus and critical thinking groups, 11% of group members selected the correct candidate for the job before discussion. After discussion, the critical thinking primed groups substantially outperformed the consensus primed groups — 67% of the former made the correct decision, compared to only 22% of the latter. In a similar study, Galinsky and Kray, (2003) found that counterfactually primed groups made the correct decision 40–67% of the time, compared to 15–23% for non-counterfactual primed groups (the range here reflects lowest to highest best estimates from multiple experiments).
Anonymity
Senior, high-status team members often dominate the lower-status members, even though the junior members are often more accurate (Kerr and Tindale, 2011). In workshop conditions, junior members are often reluctant to speak up if their view contradicts more senior members, to the detriment of group accuracy (Thomas and Fink, 1961; Galinsky et al., 2008), and halo effects mean that junior people defer to the wisdom of senior people (Thorndike, 1920), even though seniority does not tend to correlate with performance (Burgman et al., 2011). Anonymity, such as adding material online, can buffer against this, reducing social barriers to participation in group deliberations by insulating group members from reputational pressures (Valacich et al., 1992) and reducing the pressure to conform with scoring.
Give group feedback
Providing feedback, such as seeing the distribution of anonymously provided judgements, may improve estimation performance (Mukherjee et al., 2015; Wintle et al., 2013). Encouraging discussion on the distribution, and getting feedback on the judgements and reasoning of other group members before revising judgements, is an essential component of the Delphi Technique and IDEA protocols (described in more detail in Section 5.5).
Hold group members accountable (for the process)
Group members who are held accountable for their decisions show increased motivation, self-criticism, and information processing (Scholten et al., 2007). For example, professional auditors who are held accountable make more accurate classifications of industrial bond issues into financial rating categories (Ashton, 1992). But not all kinds of accountability are effective.
‘Process accountability’ (being accountable for the way judgements were reached) is typically thought to improve information processing because people need to demonstrate a comprehensive understanding of the decision problem (e.g. Lerner and Tetlock, 1999; De Dreu et al., 2000). It has been found to improve accuracy by encouraging people to take more of the available information into account; without it, people are more likely to formulate judgements based on insufficient evidence. ‘Outcome accountability’ involves evaluating groups based on the outcomes of their decisions. It affects information processing in a different way because people focus on pleasing an audience, introducing a question of whether accountability alters how people think or merely what people say they think (Lerner and Tetlock, 1999). It can erode accuracy by increasing the amount of noise (or ‘scatter’) in participants’ judgments (Siegel-Jacobs and Yates, 1996) and might cause people to focus on irrelevant details (Stewart et al., 1998).
Evaluate each other’s judgements
Studies have shown that people are better at ‘evaluation’ (assigning a probability that their selection is true or contains the truth, in the case of intervals) than ‘production’ (also known as ‘generation’). Winman et al. (2004) give an example: imagine you are about to buy a house and you want to know what the interest rates will be over the coming year; you could either ask your financial advisor for an interval of probable interest rates, or you could create your own interval and ask the financial advisor to evaluate the probability that your interval will contain the true value. These are formally equivalent ways of expressing uncertainty about the same event, yet Winman et al. show that the latter format almost abolishes overconfidence. This prompted Aidan Lyon, Fiona Fidler, Bonnie C. Wintle and Mark A. Burgman (unpublished) to conduct an experiment where participants produced and evaluated their own interval judgments for a series of quantitative questions before also evaluating someone else’s. Four replications of this experiment (total n = 111) showed that judgement swapping resulted in a 6.88% percentage point reduction in overconfidence compared to evaluating their own interval.
Consider the judgements of others
Group members could practically benefit from simple elicitation techniques that encourage them to think about what other people would do, or what sort of judgements they might make. Yaniv and Choshen-Hillel (2012a) asked participants to estimate the calories contained in 20 target foods — what is the calorie value of an orange? — then view five additional randomly drawn answers suggested by group members displayed below their own. After viewing the five advisory estimates, participants made a second, final judgement. Participants had a mean absolute error of 91.2 for their initial judgements and a more accurate subsequent final judgement (76.2), indicating that they integrated — to a good extent — the judgments of the five anonymous advisors.
This is similar logic to another study (Yaniv and Choshen-Hillel, 2012b) showing that people improve their accuracy when putting themselves in someone else’s shoes, that is, when asked to predict the judgement that another (matched) participant would give if they were shown the same set of estimates. Related research shows that voting polls are more accurate when people are asked for their expectations (who do you think will win the upcoming election?) rather than their intentions (if the election were held today, who would you vote for?) (Rothschild and Wolfers, 2011). Similarly, ‘social circle’ polls that ask participants about the voting intentions of their social contacts are also more accurate (Galesic et al., 2018).
In expert elicitation, there is a benefit to seeking an initial, independent judgement from each expert that genuinely reflects that expert’s knowledge and perspective, before integrating other perspectives.
Diversity
The benefit of groups depends on the diversity of their members (Hong and Page, 2004, 2020; Page, 2007), partly because biases in different directions cancel each other out in the group average, but also because individual members of a diverse group bring different perspectives and ignite interesting debates. When planning for a hydroelectric facility in Canada, Failing et al. (2007) show that combining technical expertise with other knowledge sources drawn from local residents and Indigenous communities promoted a broader understanding of causal pathways and the consequences of flow changes to the river system, and made it more acceptable to a broader range of stakeholders. Theoretically, it has been shown that the benefit of groups is greatest where the overlap between the knowledge bases of individual members is least (i.e. members possess independent knowledge) (Clemen and Winkler, 1985). Homogenous groups induce spurious consensus and interdependence, producing unduly high confidence without the accuracy to match (Yaniv et al., 2009). Selecting for member diversity using information on demographics, experience, worldview, and cognitive reasoning style may be one way to reduce dependency between members, and studies have shown diverse groups to outperform homogenous groups in terms of quality of problem solutions (Worchel et al., 1992).
Where group members are not already diverse, then it may be possible to stimulate cognitive diversity (different ways of thinking within a group), for example, different mindsets can be fostered or primed via framing a problem positively for some group members (e.g. money saved), and negatively for others (e.g. money spent) (Yaniv, 2011).
Role playing within a group has been found to improve forecasting accuracy (Armstrong, 2001). This might involve giving members different cost functions for the question at hand or getting them to act out an interaction that they might expect to see from representatives of different parties.
Managing communication and conflict in diverse groups
Much of the literature on group decision making assumes that members will cooperate, share information objectively and work towards more informed decisions. However, diverse groups can be prone to conflict and communication breakdowns (e.g. O’Reilly and Chatman, 1986). Process losses such as these will affect group performance. In highly value-laden cases, there is likely to be some extent of polarisation. This may be exacerbated where experts are involved, because they have a long history of gathering evidence to support their position (Mercier and Sperber, 2011). Taber and Lodge (2006) studied how people evaluate arguments about affirmative action and gun control, finding attitude polarisation to be more pronounced in subjects who are more knowledgeable.
But conflict is not all bad. It can be a source of cognitive growth, where individuals have different responses to the same problem and are motivated to achieve a joint solution. For example, certain forms of interpersonal disagreement can facilitate intellectual development in children (Azmitia and Perlmutter, 1989). Jehn et al. (1999) hypothesised that informational diversity would lead to task conflict in groups. That is to say, group members with different expertise, education and training may take different approaches to the task, both in content (what to do) and process (how to do it). Their results suggested that informational diversity did indeed lead to task conflict when it came to content, but not process. But nonetheless, informational diversity improved group performance and efficiency, especially for complex tasks.
When groups are not communicating well, the potential benefits of informational diversity often go unrecognised (Steiner, 1972). For example, Dougherty (1992) found that product teams of group members with different functional training struggled to get their products to market. This is the same reason why group members often fail to uncover hidden profiles (Stasser and Titus, 1985), because they fixate too much on common ground, which — in the case of diverse groups — is rather limited. Importantly, it illustrates that structured approaches to elicitation and discussion are critical for diverse groups, by explicitly eliciting unique information and reasons for/against from each group member, individuals are prompted to share information.
Providing feedback on positive performance and achievement to diverse groups is likely to increase motivation and relieve frustration, even if some level of conflict remains. Indeed, Jehn et al. (1999) found that diverse groups that were performing well reported high morale, yet still reported relationship conflict. Therefore, the positive effect of group performance overwhelmed the negative effect of relationship conflict on morale.
Socratic questioning
‘Dialectical bootstrapping’ as outlined by (Herzog and Hertwig, 2009) is akin to ‘Socratic questioning’. Socratic questioning is a way to get experts to consider alternative models, explore hidden assumptions and sources of information, consider alternative ways to express their opinions or provide meaning, and consider the relevance of questions themselves (Elder and Paul, 1998). This type of questioning is commonly used in the IDEA protocol (see Section 5.5.3) by the facilitator, however, it could be taught to participants as a way of cross-examining one another’s reasoning and assumptions. While more evidence is required, Yang (2008) demonstrated a statistically significant improvement in how groups of students equipped to question reasoning and assumptions through Socratic questioning improved their cognitive thinking skills more than groups that were enabled to simply undertake unstructured deliberations without the training.
Consider the aggregation method
When combining the judgements of multiple experts, different methods can yield better-aggregated judgements in different contexts. In his review of methods for combining forecasts, Clemen (1989) reports that simple aggregation methods are more effective in most cases, and the median and the mean often perform similarly (e.g. Palan et al., 2020). An important consideration is that the median is more robust to outliers, whereas the mean is sensitive to outliers. This is also an issue if individuals are trying to manipulate the outcome by giving extreme values. If you have reason to believe that outliers, or more extreme judgements, contain important information, then the mean may be more appropriate than the median, perhaps even an extremised aggregate or the geometric mean of odds, which have been found to perform well in probability aggregation (Satopää et al., 2014; Baron et al., 2014; see also Hanea et al., 2021b). For a useful summary that includes empirical data, see https://forum.effectivealtruism.org/posts/acREnv2Z5h4Fr5NWz/my-current-best-guess-on-how-to-aggregate-forecasts. Means are usually recommended if the judgements are normally distributed, and medians better reflect skewed data distributions. Taking an equally weighted average of the group has been found to be a robust method for achieving accurate and well calibrated expert judgements (Hemming et al., 2020b).
Scoring and weighting individuals
The alternative to equally weighting judgements from each expert is to give greater weight to the judgements of those who you have reason to believe might perform better. One of the few methods for weighting experts that has support is to use ‘seed’ questions (also known as ‘calibration’ or ‘test’ questions) (Aspinall and Cooke, 2013; French, 2011). Seed questions are questions which relate to main elicitation questions, but for which a resolution to the question can be obtained by the time the judgements of experts need to be aggregated. Experts are scored on the seed questions. The performance of experts on seed questions is used to develop weights for aggregating expert judgements (Hemming et al., 2020b). This method underpins the Classical Model for Structured Expert Judgement (Cooke, 1991). The Classical Model is most often used for assessing continuous random variables (quantities). However, it can be used to assess probabilities assigned to discrete events (Hanea et al., 2016). A recent review by Colson and Cooke (2017), demonstrated that across the 73 case studies to which the Classical Model has been applied, performance-weights out-performed equal weights in 74% of those cases.
In order to develop weights for experts based on test questions, scoring rules are required. An important property of a scoring rule is that it should not influence the forecaster in an undesirable way (Brier, 1950). When applying scoring rules it is important that the assessor and the experts understand the scoring rules, as different scoring rules have different underlying assumptions and properties for which they aim to maximise (e.g. different theoretical distributions (Cooke, 1991), and can lead to a different ranking of experts (Winkler and Murphy, 1968).
Table 5.3 Summary of strategies for improving group judgements.
Consider group size
Studies on the number of people required to see the wisdom of crowds effect draw different conclusions, with diminishing returns found beyond anywhere from 5–6 (Hora, 2004), 8–12 (Hogarth, 1978), and 50 participants (Satopää et al., 2014). Hemming et al. (2018b) found that groups containing 5–9 participants considerably outperformed the median individual, with six of the eight groups being more accurate than 75% of individuals in round two. On combining the judgements of all 58 participants into a ‘supergroup’, it performed as well as the average group, but no better.
The wisdom of the crowd effect can be largely explained as a statistical phenomenon whereby judgements of individuals are random independent samples. If the samples are diverse then the information pool related to the questions will increase (Clemen and Winkler, 1999), and the errors of individuals are likely to cancel each other out (Larrick and Soll, 2006). Fewer than five individuals can lead to groups which are heavily influenced by outliers, while beyond nine individuals the contribution of each new member often bears little weight on the final aggregation.
The ideal group size depends on a great many factors (e.g. whether interactions are allowed, whether the discussion is facilitated, whether the elicitation is online or face-to-face, synchronous or asynchronous, etc.). Group size effects are particularly moderated by the type of task (see Kerr and Tindale, 2011, for a discussion). For example, it can depend on the extent to which the correct answer can be demonstrated (Bonner and Baumann, 2008), or the creativity of the task; when brainstorming, larger groups can generate more creative ideas (Coskun, 2011). Group size can also moderate the extent to which participants share information (Stasser et al., 1989). Bonner and Baumann (2008) suggest that when group size is small (e.g. 3 people), all members may participate in the discussion and consider the input of others while as group sizes become large (6+), evaluating each individual contribution becomes burdensome and so expert status becomes more influential (underscoring the importance of anonymity). Larger groups may also be more prone to ‘social loafing’ (relying on others to contribute).
Taking these considerations into account, we suggest 5–12 people is an appropriate group size for expert elicitation. Advice for IDEA groups (see Section 5.5.3) is to aim for about 8–12 participants to account for the loss of a few from attrition, and no more than 20 (Hemming et al., 2018a). This strikes a useful balance between the sensitivity of the aggregated estimate to the assessments of single individuals (seen with smaller groups), with the washing out of individual contributions (seen with larger groups).
5.5 Structured Frameworks for Making Group Judgements
The approaches to reduce bias described above can be easily integrated into a broader framework for eliciting expert judgements. It may be tempting to just use unstructured face-to-face groups: a minimally-facilitated discussion where participants sit at a single table and discuss a question until consensus is considered reached. While unstructured groups are ubiquitous (Graefe and Armstrong, 2011; Kerr and Tindale, 2011), and an improvement on relying on a single expert judgement, the lack of use of any of the strategies outlined above for mitigating biases greatly reduces the effectiveness.
This section describes five structured methods for incorporating a set of principles, including anonymity, considering the opposite/counterfactual thinking, diversity, feedback, information sharing, mathematical (rather than behavioural) aggregation and weighting. A comprehensive controlled experiment (Graefe and Armstrong, 2011, n = 227) comparing four group formats, Face-to-Face (FTF), Nominal Group Technique (NGT), Delphi technique (Delphi), and Prediction Market (PM), found that judgments from the most structured elicitation formats (NGT, Delphi) were more accurate (lower mean absolute error, MAE) than PMs or unstructured formats (FTF). This supports similar findings from earlier reviews (e.g. Rowe and Wright, 1999). Graefe and Armstrong also compared the accuracy of structured group judgements to their prior individual estimates. For all three structured techniques (NGT, Delphi, PMs), the mean group result was statistically significantly (p<.01) more accurate than the average of the initial estimates. The MAE reductions were: NGT =3.1; Delphi = 3.6 and PM = 3.05. Differences between these techniques were small, and not statistically significant. FTF was not included, as this method provided no benchmark to assess error reduction against.
Each of these techniques is briefly outlined in the following sections together with a variant of the Delphi technique (the IDEA protocol) and ‘superforecasters’.
5.5.1 Delphi technique
The Delphi technique (Linstone and Turoff, 1975) is an elicitation procedure developed in the mid-1940s to improve forecasting technology during the Cold War. The purpose of the Delphi design is to mitigate dominating individuals, and problems such as the halo effect and groupthink (Mukherjee et al., 2015). In traditional Delphi groups, participants make estimates remotely and anonymously. That is, they do not meet face-to-face or learn each other’s identities. In practice, this may vary, for example, not all Delphi groups will be entirely anonymous. Participants are usually invited to make written comments justifying or explaining their estimates, so there may be minimal interaction between group members, but it is substantially less than in FTF and NGT. The Delphi process is often described as iterative, meaning that estimates are made in ‘rounds’, with feedback about each other’s estimates, and the accompanying comments, provided to participants between rounds by a facilitator. The number of rounds can vary, but is typically limited to two or three, although some stop once there is little change in the judgements by experts (e.g. behaviour consensus is achieved). The group result is usually the mean or median of the final round of estimates.
Delphi technique is also used for structuring group discussions for qualitative judgements (e.g. horizon scanning, problem formulation, objective setting, and identifying options for action) so is not exclusively used for numerical estimates (Mukherjee et al., 2015). See Box 5.2 for a step-by-step guide to the Delphi technique.
5.5.2 Nominal Group technique
The Nominal Group Technique (NGT) was developed in the early 1970s (Van de Ven and Delbecq, 1971). In an NGT, participants are asked by a facilitator to individually reflect and generate ideas, typically based on a structured questionnaire. Subsequently, participants are asked to collectively prioritise the ideas and suggestions issued by the group members (Hugé and Mukherjee, 2018). The process of individual and collective reflection and co-production of knowledge among participants in NGT allows for a depolarising approach to the study and management of contentious issues (Hugé and Mukherjee, 2018). NGT interactions also fare well in satisfaction ratings by participants, considerably outranking Delphi and PMs and beating FTF by a small margin (Graefe and Armstrong, 2011).
Note that studies that compare the performance of consensus group judgement with a straight mathematical aggregate of the individual judgments within a group (e.g. the group average) often refer to this control as the ‘nominal group’ (e.g. Schultze et al., 2012). This use of the term nominal is different to its use in NGT. The NGT steps are outlined in Box 5.3.
5.5.3 IDEA and related protocols
IDEA — Investigate, Discuss, Estimate and Aggregate (Burgman, 2015; Hanea et al., 2017) — is a structured expert elicitation protocol designed to help elicit more accurate quantitative and probabilistic judgements (along with rationales) from experts. Akin to the Delphi technique it involves the essential steps of allowing individuals to make a private individual estimate, before viewing other people’s anonymised judgements and rationales, and revising their estimates. It is designed explicitly for the elicitation of quantities and probabilities (facts, rather than qualitative opinions); in contrast to Delphi technique it is more prescriptive in its guidance for making these estimates (e.g. using three-step and four-step interval estimates; Burgman, 2015), and uses guided social interactions to avoid the biassing elements of group deliberation and behavioural aggregation (Hanea et al., 2017). Final round judgements are usually aggregated by taking equal-weighted means. Performance weighting may also be used, but requires that calibration questions are seeded in the survey from which to develop weights (e.g. see Hemming et al., 2020b). See Box 5.4 for an outline of the basic steps.
Two other notable protocols for eliciting expert judgements that are also applied in ecology include the Sheffield Elicitation Framework (SHELF) (Gosling, 2018), which is also akin to the Delphi technique but which relies on behavioural aggregation (Fitzgerald et al., 2021; O’Hagan, 2019), and the Classical Model (also known as Cooke’s Method), which typically only allows a single estimate from experts and uses performance-weighted aggregation (Cooke, 1991). Recent applications of the IDEA protocol have adopted the performance-weighted aggregations of the Classical Model, that is, giving greater weight to the judgements of those who performed better on test questions (Barons and Aspinall, 2020; Hanea et al., 2021a).
Testing of the protocol has provided compelling evidence that: 1) entrusting an equal-weighted aggregate of a diverse group of individuals typically provides a better judgement than a single well-credentialled individual (Burgman et al., 2011; Hemming et al., 2020a, 2018b); 2) discussion after providing an initial, private estimate typically improves group judgements of individuals and groups (Wintle et al., 2021) — sometimes this is simply because linguistic ambiguity and mistakes made in the first round are addressed, other times it is because critical evidence has been introduced, and diverse opinions discussed; and 3) the way questions are asked can help experts to better communicate their judgements, for example, a four-step question format by Speirs-Bridge et al. (2010) helps overcome overconfidence. The countless case studies that have applied the protocol show it is also practical to apply regardless of whether experts are to be convened remotely (e.g. McBride et al., 2012), in a face-to-face workshop (e.g. Wintle et al., 2021), or a hybrid approach (e.g. Hanea et al., 2017), and regardless of whether estimation and discussion take place synchronously or asynchronously.
5.5.4 Prediction Markets
Prediction Markets (PMs) allow individuals to trade on the outcome of future events (Wolfers and Zitzewitz, 2004). They create contracts that pay a fixed amount if an event occurs, and then allow people to trade on the contract by buying or selling in a manner comparable to financial markets. For example, a contract may pay $1 if an event occurs by a prespecified date (e.g. will average oil prices be higher at midnight the last day of this month than at 00.01am on the first day?), and $0 otherwise. Participants are incentivised to buy shares when they think the current estimate is too low, and to sell when they think it is too high. The price at which the contract trades at a given time can then be interpreted, with some caveats (Manski, 2006), as the market’s collective forecast of the probability of the event occurring.
Similar to the Delphi technique, PMs are typically anonymous. They differ from the Delphi technique in that they are continuously updated by participants, who typically do not share information with each other. Rather, they respond directly to the price signal. These features mean that PMs often correct, rather than amplify, the effects of individual errors by creating powerful incentives to disclose, rather than to conceal, privately held views. PMs have been successfully applied in domains such as politics, sports and business, and have been found to generate relatively accurate forecasts, in many cases outperforming the statistical aggregation of prior individual estimates (e.g. Graefe and Armstrong, 2011; Dreber et al., 2015), but not always (e.g. see Atanasov et al., 2017). A drawback is that to work they require a large enough number of active traders with different opinions, and participants’ satisfaction with them is usually low (Graefe and Armstrong, 2011; Kerr and Tindale, 2011). The PM steps are outlined in Box 5.5.
5.5.5 Superforecasters
The notion of superforecasting (Mellers et al., 2014, 2015; Tetlock and Gardner, 2016) arose from a large forecasting tournament funded by the US government (IARPA) to overcome some of the forecasting challenges faced by the US intelligence community. Elite teams, comprising the top 2% of performers from the first year of the forecasting tournament — the superforecasters — worked together in the second year, outperforming all the other teams by a wide margin for two years in a row, rather than regressing to the mean. Mellers et al. (2014) argue that team communication, in particular, the exchange of rationales, news articles and other information, and debating differences of opinion, produced ‘enlightened cognitive altruism’ among group members. This, combined with psychological interventions, including training on probability and scenarios, proved key to the success of their team’s forecasting. The researchers suggest that simply receiving the ‘exalted’ title of superforecasters substantially boosted effort and engagement from team members. See Box 5.6 for an outline of the basic steps.
5.6 Practical Methods for Improving Routine Judgements
The last section described five structured approaches that warrant much greater use. The aim of this section is to recognise that there are occasions when these methods might be too complex or time consuming to adopt comprehensively, but that some features can usually be included to improve the rigour of judgements. This section illustrates how some of these features might be applied to a series of those routine judgements. We envisage that the organiser of the job selection panel or the group deciding which projects to fund will introduce these more rigorous processes.
Regardless of the issue, there are a series of general considerations that apply to all methods. Adding any of these components will improve the rigour. Section 5.4 describes options for improving further.
- Decide on the precise issue that is being evaluated.
- Determine whether this is about making a choice (e.g. employing someone, deciding which projects to fund) or considering a fact (the veracity of an observation or estimating a parameter).
- Decide whether to break down the issue into intermediate stages, such as the probability of rats colonising an island, and the conditional probability of various reptile species going extinct if they do. This helps decompose the problem.
- Clearly define the exact questions to avoid ambiguity.
- Decide on a scoring process for eliciting responses. A wider score range, say 1–10 or 1–20 reduces the number of ties; a 1–5 range tends to result in many rather uninformative 3s and 4s. Where there is a threshold of acceptability, or you wish to avoid fence-sitting, you might use, say, 1–10, where 1 = definitely not; 5 = on balance, no; 6 = on balance; yes, 10 = definitely yes.
- Taking the median reduces the impact of extreme values or those wishing to manipulate the outcome.
- Decide how to present evidence, such as giving reasons for and against and elicit further evidence from the group.
- Decide how to enhance the independence and ideally anonymity of judgements. A key element is that the participants do not state their views prior to scoring, to retain independence. The minimum approach is for everyone to write down their score but not show others and then state this in turn. A better strategy is one where everyone writes their score on paper or cards, which are collated (e.g. can just be laid out on the table — providing identical pens increases anonymity). Better still is to score online using a template, survey or software, so anonymous scores can be easily compiled.
- Decide on the scoring sequence. The minimum is discussion, then scoring. Introducing an initial round of scoring, then discussion, then scoring again, improves judgements.
- Decide on how the discussions will be run, including ground rules for participation (e.g. steps to avoid exposing who produced which judgements).
The following methods describe minimal ways of improving three of the most common types of expert elicitation. Each of these are inferior to adopting more comprehensive methods described above and in other chapters but superior to conventional practice.
5.6.1 Judging the veracity of a statement
Evidence is very often expressed as a statement. This could be a claim to have seen a species, the assertion that a species is locally extinct, or the conclusion that the species usually nests in old woodpecker holes. Such a process could be adopted for record committees, such as bird recorders deciding which records they accept. It can be part of the decision-making processes described in Chapter 8 or used when embedding evidence into processes, such as management plans, policies or models, as described in Chapter 9. Courts could be changed so that jurors have to apply a similar process in deciding whether or not the accused is guilty. Box 5.7 outlines a simple process for undertaking such a task.
If making repeated decisions, one option to improve efficiency is to accept the statement if everyone gives a high score above, say 7 or above, and reject if everyone gives a low score, say, 3 or lower. Thus a panel deciding upon bird records can concentrate on those records that all are uncertain about, and those where there is disagreement.
5.6.2 Selecting options
Ranking items is a regular component of decision making in which there are a series of options of which some need to be selected, for example deciding which research projects to support or which species or areas are prioritised for conservation action. Sometimes the task is to identify a subset placed in rank order, for example, judging the most suitable job applicant and three backups.
A common practice is to score a range of criteria and then add to give a total sum. However, there are various problems with this, including how to weight each criteria, whether they are additive, and whether scores can be considered linear and equidistant (is the distance between a 4 and 5 the same as the distance between a 9 and 10? Do scores of 10, 10, 2 beat 8, 7, 7?). It is now accepted that adding scores is deeply flawed and better replaced by decision science methods (Klein et al., 2014), as described in Section 8.5. This process entails removing options that are below the minimum threshold, those that are inferior across all criteria, i.e. ‘dominated alternatives’, and also removing criteria that are no longer informative, i.e. ‘redundant criteria’, for example, criteria on which all remaining options score about the same, or all options are at a sufficient level. Applying methods from decision science prompts us to explicitly grapple with trade-offs, for example, whether the more impressive research project justifies the increased cost or whether to support the project with lower expected conservation gains but exciting opportunities for education.
5.6.3 Estimating a numeric value
Estimating a quantity or probability is often called for, but the evidence may be unclear, complicated, or disputed. This could be the population size of a species, the change in population size over the last decade, or parameters for a model. The process outlined below is effectively the same as the IDEA or Delphi technique protocols.
References
Anderson, J.R. 1981. Cognitive Skills and Their Acquisition (Psychology Press), https://doi.org/10.4324/9780203728178.
Armstrong, J.S. 2001. Role playing: A method to forecast decisions. In: Principles of Forecasting, International Series in Operations Research & Management Science, Vol. 30, ed. by J.S. Armstrong (Boston, MA: Springer), pp.15–30, https://doi.org/10.1007/978-0-306-47630-3_2.
Ashton, R.H. 1992. Effects of justification and a mechanical aid on judgment performance. Organizational Behavior and Human Decision Processes 52: 292–306, https://doi.org/10.1016/0749-5978(92)90040-e.
Aspinall, W.P. and Cooke, R.M. 2013. Quantifying scientific uncertainty from expert judgement elicitation. In: Risk and Uncertainty Assessment for Natural Hazards, ed. by J. Rougier, et al. (Cambridge: Cambridge University Press), pp.64–99, https://doi.org/10.1017/CBO9781139047562.005.
Atanasov, P., Rescober, P., Stone, E. et al. 2017. Distilling the wisdom of crowds: Prediction markets vs. prediction polls. Management Science 63: 691–706, https://doi.org/10.1287/mnsc.2015.2374.
Azmitia, M. and Perlmutter, M. 1989. Social influences on children’s cognition: State of the art and future directions. Advances in Child Development and Behavior 22: 89–144, https://doi.org/10.1016/S0065-2407(08)60413-9.
Bar-Hillel, M. 1980. The base-rate fallacy in probability judgments. Acta Psychologica 44: 211–33, https://doi.org/10.1016/0001-6918(80)90046-3.
Barnett, A.G., van der Pols, J.C,. and Dobson, A.J. 2005. Regression to the mean: What it is and how to deal with it. International Journal of Epidemiology 34: 215–20, https://doi.org/10.1093/ije/dyh299.
Baron, J., Mellers, B.A., Tetlock, P.E., et al. 2014. Two reasons to make aggregated probability forecasts more extreme. Decision Analysis 11: 133–45, https://doi.org/10.1287/deca.2014.0293.
Barons, M.J. and Aspinall, W. 2020. Anticipated impacts of Brexit scenarios on UK food prices and implications for policies on poverty and health: A structured expert judgement approach. BMJ Open 10: e032376, https://doi.org/10.1136/bmjopen-2019-032376.
Benson, P.G and Önkal, D. 1992. The effects of feedback and training on the performance of probability forecasters. International Journal of Forecasting 8: 559–73, https://doi.org/10.1016/0169-2070(92)90066-I.
Berthet, V. 2022. The impact of cognitive biases on professionals’ decision-making: A review of four occupational areas. Frontiers in Psychology, 12: Article 802439, https://doi.org/10.3389/fpsyg.2021.802439.
Bilalic, M., Langner, R., Erb, M., et al. 2010. Mechanisms and neural basis of object and pattern recognition: A study with chess experts. Journal of Experimental Psychology 139: 728–42, https://doi.org/10.1037/a0020756.
Bonner, B.L. and Baumann, M.R. 2008. Informational intra-group influence: The effects of time pressure and group size. European Journal of Social Psychology 38: 46–66, https://doi.org/10.1002/ejsp.400.
Bourne, L., Jr, Kole, J. and Healy, A. 2014. Expertise: Defined, described, explained. Frontiers in Psychology 5: Article 186, https://doi.org/10.3389/fpsyg.2014.00186.
Brier, G.W. 1950. Verification of forecasts expressed in terms of probability. Monthly Weather Review 78: 1–3, https://doi.org/10.1175/1520-0493(1950)078<0001:VOFEIT>2.0.CO;2.
Burgman, M.A. 2005. Risks and Decisions for Conservation and Environmental Management, Ecology, Biodiversity, and Conservation (New York: Cambridge University Press), https://doi.org/10.1017/CBO9780511614279.
Burgman, M.A. 2015. Trusting Judgements: How to Get the Best out of Experts (Cambridge: Cambridge University Press), https://doi.org/10.1017/CBO9781316282472.
Burgman, M.A., McBride, M., Ashton, R., et al. 2011. Expert status and performance. PLoS ONE 6: e22998., https://doi.org/10.1371/journal.pone.0022998.
Canessa, S., Taylor, G., Clarke, R.H., et al. 2020. Risk aversion and uncertainty create a conundrum for planning recovery of a critically endangered species. Conservation Science and Practice 2: e138, https://doi.org/10.1111/csp2.138.
Caputo, A. 2013. A literature review of cognitive biases in negotiation processes. International Journal of Conflict Management 24: 374−98, https://doi.org/10.1108/IJCMA-08-2012-0064.
Charness, N., Tuffiash, M., Krampe, R., et al. 2005. The role of deliberate practice in chess expertise. Applied Cognitive Psychology 19: 151–65, https://doi.org/10.1002/acp.1106.
Clemen, R. 1989. Combining forecasts: A review and annotated bibliography. International Journal of Forecasting 5: 559−83, https://doi.org/10.1016/0169-2070(89)90012-5.
Clemen, R. and Winkler, R.L. 1985. Limits for the precision and value of information from dependent sources. Operations Research 33: 427–42, https://doi.org/10.1287/opre.33.2.427.
Clemen, R. and Winkler, R.L. 1999. Combining probability distributions from experts in risk analysis. Risk Analysis: An International Journal 19: 187–203, https://doi.org/10.1111/j.1539-6924.1999.tb00399.x.
Colson, A.R. and Cooke, R.M. 2017. Cross validation for the classical model of structured expert judgment. Reliability Engineering & System Safety 163: 109–20,http://dx.doi.org/10.1016/j.ress.2017.02.003.
Cooke, R. 1991. Experts in Uncertainty: Opinion and Subjective Probability in Science (New York: Oxford University Press on Demand).
Cooper, G.S. and Meterko, V. 2019. Cognitive bias research in forensic science: A systematic review. Forensic Science International 297: 35–46, https://doi.org/10.1016/j.forsciint.2019.01.016.
Coskun, H. 2011. The effects of group size, memory instruction, and session length on the creative performance in electronic brainstorming groups. Kuram Ve Uygulamada Egitim Bilimleri 11: 91–95, https://files.eric.ed.gov/fulltext/EJ919891.pdf.
Dawes, R.M. 1994. House of Cards: Psychology and Psychotherapy Built on Myth (New York: Free Press).
De Dreu, C.K.W., Koole, S.J. and Steinel, W. 2000. Unfixing the fixed pie: A motivated information-processing approach to integrative negotiation. Journal of Personality and Social Psychology 79: 975–87, https://doi.org/10.1037//0022-3514.79.6.975.
Dougherty, D. 1992. Interpretive barriers to successful product innovation in large firms. Organization Science 3: 179–202, https://doi.org/10.1287/orsc.3.2.179.
Dreber, A., Pfeiffer, T., Almenberg, J., et al. 2015. Using prediction markets to estimate the reproducibility of scientific research. Proceedings of the National Academy of Sciences 112: 15343-7, https://doi.org/10.1073/pnas.1516179112.
Drescher, M. and Edwards, R.C. 2018. A systematic review of transparency in the methods of expert knowledge use. Journal of Applied Ecology 56: 436–49, .
Ehrlinger, J., Gilovich, T. and Ross, L. 2005. Peering into the bias blind spot: Peoples’ assessments of bias in themselves and others. Personality and Social Psychology Bulletin 31: 680–92, https://doi.org/10.1177/0146167204271570.
Elder, L. and Paul, R. 1998. The role of Socratic questioning in thinking, teaching, and learning. The Clearing House 71: 297–301, https://doi.org/10.1080/00098659809602729.
Ericsson, K.A., Krampe, R.T. and Tesch-Roemer, C. 1993. The role of deliberate practice in the acquisition of expert performance. Psychological Review 100: 363–406, https://doi.org/10.1037/0033-295X.100.3.363.
Ericsson, K.A. and Lehmann, A.C. 1996. Expert and exceptional performance: Evidence of maximal adaptation to task constraints. Annual Review of Psychology 47: 273–305, https://doi.org/10.1146/annurev.psych.47.1.273.
Failing, L., Gregory, R. and Harstone, M. 2007. Integrating science and local knowledge in environmental risk management: A decision-focused approach. Ecological Economics 64: 47–60, https://doi.org/10.1016/j.ecolecon.2007.03.010.
Fischer, G.W. 1982. Scoring-rule feedback and the overconfidence syndrome in subjective probability forecasting. Organizational Behavior and Human Performance 29: 352–69, https://doi.org/10.1016/0030-5073(82)90250-1.
Fitzgerald, D.B., Smith, D.R., Culver, D.C., et al. 2021. Using expert knowledge to support endangered species act decision‐making for data‐deficient species. Conservation Biology 35: 1627–38, https://doi.org/10.1111/cobi.13694.
Fournier, A.M.V, White, E.R. and Heard, S.B. 2019. Site‐selection bias and apparent population declines in long‐term studies. Conservation Biology 33: 1370–79, https://doi.org/10.1111/cobi.13371.
French, S. 2011. Aggregating expert judgement. Revista de La Real Academia de Ciencias Exactas, Fisicas y Naturales. Serie A. Matematicas 105: 181–206, https://doi.org/10.1007/s13398-011-0018-6.
French, S. 2012. Expert judgment, meta-analysis, and participatory risk analysis. Decision Analysis 9: 119–27, https://doi.org/10.1287/deca.1120.0234.
Froggatt, W.W. 1936. The introduction of the great Mexican toad Bufo Marinus into Australia. The Australian Naturalist 9: 163–64.
Galesic, M., Bruine de Bruin, W., Dumas, M., et al. 2018. Asking about social circles improves election predictions. Nature Human Behaviour 2: 187–93, https://doi.org/10.1038/s41562-018-0302-y.
Galinsky, A. and Kray, L. 2003. From thinking about what might have been to sharing what we know: The effects of counterfactual mind-sets on information sharing in groups. Journal of Experimental Social Psychology 40: 606–18, https://doi.org/10.1016/j.jesp.2003.11.005.
Galinsky, A.D., Magee, J.C., Gruenfeld, D.H., et al. 2008. Power reduces the press of the situation: Implications for creativity, conformity, and dissonance. Journal of Personality and Social Psychology 95: 1450–66, https://doi.org/10.1037/a0012633.
Gigerenzer, G., Gaissmaier, W., Kurz-Milcke, E., et al. 2008. Helping doctors and patients make sense of health statistics. Psychological Science in the Public Interest: 53–96, https://doi.org/10.1111/j.1539-6053.2008.00033.x.
Gosling, J.P. 2018. SHELF: The Sheffield Elicitation Framework. In: Elicitation. International Series in Operations Research & Management Science, vol CCLXI, ed. by L. Dias, et al. (Cham, Switzerland: Springer), https://doi.org/10.1007/978-3-319-65052-4_4.
Graber, M. 2005. Diagnostic errors in medicine: A case of neglect. The Joint Commission Journal on Quality and Patient Safety 31: 106–13, https://doi.org/10.1016/S1553-7250(05)31015-4.
Graefe, A. and Armstrong, J.S. 2011. Comparing face-to-face meetings, nominal groups, Delphi and prediction markets on an estimation task. International Journal of Forecasting 27: 183–95, https://doi.org/10.1016/j.ijforecast.2010.05.004.
Gregory, R, Failing, L., Harstone, M., et al. 2012. Structured Decision Making: A Practical Guide to Environmental Management Choices (Chichester, West Sussex: Wiley-Blackwell), https://doi.org/10.1002/9781444398557.
Gregory, R. and Keeney, R.L. 2017. A practical approach to address uncertainty in stakeholder deliberations. Risk Analysis 37: 487–501, https://doi.org/10.1111/risa.12638.
Hanea, A.M., McBride, M.F., Burgman, M.A., et al. 2016. Classical meets modern in the IDEA protocol for structured expert judgement. Journal of Risk Research 21: 417–33, https://doi.org/10.1080/13669877.2016.1215346.
Hanea, A.M., McBride, M.F., Burgman, M.A., et al. 2017. Investigate Discuss Estimate Aggregate for structured expert judgement. International Journal of Forecasting 33: 267–79, https://doi.org/10.1016/j.ijforecast.2016.02.008.
Hanea, A.M., Hemming, V. and Nane, G.F. 2021a. Uncertainty quantification with experts: Present status and research needs. Risk Analysis 42: 254–63, https://doi.org/10.1111/risa.13718.
Hanea, A.M., Wilkinson, D.P., McBride, M., et al. 2021b. Mathematically aggregating experts’ predictions of possible futures. PLoS ONE 16: e0256919, https://doi.org/10.1371/journal.pone.0256919.
Hemming, V., Armstrong, N., Burgman, M.A., et al. 2020a. Improving expert forecasts in reliability: Application and evidence for structured elicitation protocols. Quality and Reliability Engineering International 36: 623–41, https://doi.org/10.1002/qre.2596.
Hemming, V., Burgman, M.A., Hanea, A.M., et al. 2018a. A practical guide to structured expert elicitation using the IDEA protocol. Methods in Ecology and Evolution 9: 169–80, https://doi.org/10.1111/2041-210X.12857.
Hemming, V., Camaclang, A.E., Adams, M.S., et al. 2022. An introduction to decision science for conservation. Conservation Biology 36: e13868, https://doi.org/10.1111/cobi.13868.
Hemming, V., Hanea, A., Walshe, T., et al. 2020b. Weighting and aggregating expert ecological judgements. Ecological Applications 30: e02075, https://doi.org/10.1002/eap.2075.
Hemming, V., Walshe, T.V., Hanea, A.M., et al. 2018b. Eliciting improved quantitative judgements using the IDEA protocol: A case study in natural resource management. PloS ONE 13: e0198468, https://doi.org/10.1371/journal.pone.0198468.
Herzog, S.M. and Hertwig, R. 2009. The wisdom of many in one mind: Improving individual judgments with dialectical bootstrapping. Psychological Science 20: 231–37, https://doi.org/10.1111/j.1467-9280.2009.02271.x.
Hoch, S.J. 1985. Counterfactual reasoning and accuracy in predicting personal events. Journal of Experimental Psychology-Learning Memory and Cognition 11: 719–31, https://doi.org/10.1037//0278-7393.11.1-4.719.
Hogarth, R.M. 1978. A note on aggregating opinions. Organizational Behavior and Human Performance 21: 40–46, https://doi.org/10.1016/0030-5073(78)90037-5.
Hollnagel, E. and Fujita, Y. 2013. The Fukushima disaster–Systemic failures as the lack of resilience. Nuclear Engineering and Technology 45: 13–20, https://doi.org/10.5516/NET.03.2011.078.
Hong, L. and Page, S.E. 2004. Groups of diverse problem solvers can outperform groups of high-ability problem solvers. Proceedings of the National Academy of Sciences of the United States of America 101: 16385–89, https://doi.org/10.1073/pnas.0403723101.
Hong, L. and Page, S.E. 2020. The contributions of diversity, accuracy, and group size on collective accuracy. DecisionSciRN: Other Human Behavior & Game Theory (Topic), October 15 2020, https://doi.org/10.2139/ssrn.3712299.
Hora, S.C. 2004. Probability judgments for continuous quantities: Linear combinations and calibration. Management Science 50: 597–604, https://doi.org/10.1287/mnsc.1040.0205.
Hugé, J. and Mukherjee, N. 2018. The nominal group technique in ecology and conservation: Application and challenges. Methods in Ecology and Evolution 9: 33–41, https://doi.org/10.1111/2041-210X.12831.
Janis, I.L. 1982. Groupthink, Second edition (Boston: Houghton Mifflin).
Jasanoff, S. 2006. Transparency in public science: Purposes, reasons, limits. Law and Contemporary Problems 69: 21–45, https://scholarship.law.duke.edu/cgi/viewcontent.cgi?referer=&httpsredir=1&article=1385&context=lcp.
Jehn, K.A., Northcraft, G.B. and Neale, M.A. 1999. Why differences make a difference: A field study of diversity, conflict, and performance in workgroups. Administrative Science Quarterly 44: 741–63, https://doi.org/10.2307/2667054.
Johnson, J. and Bruce, A.C. 2001. Calibration of subjective probability judgments in a naturalistic setting. Organizational Behavior and Human Decision Processes 85: 265–90, https://doi.org/10.1006/obhd.2000.2949.
Kahneman, D. 1991. Judgment and decision making: A personal view. Psychological Science 2: 142–45, https://doi.org/10.1111/j.1467-9280.1991.tb00121.x.
Kahneman, D. and Klein, G. 2009. Conditions for intuitive expertise: A failure to disagree. American Psychologist 64: 515–26, https://doi.org/10.1037/a0016755.
Kahneman, D. and Tversky, A. 1972. Subjective probability: A judgment of representativeness. Cognitive Psychology 3: 430–54, https://doi.org/10.1016/0010-0285(72)90016-3.
Kahneman, D. and Tversky, A. 1979. Prospect theory: Analysis of decision under risk. Econometrica 47: 263–91, https://doi.org/10.2307/1914185.
Katzenstein, G. 1996. The debate on structured debate: Toward a unified theory. Organizational Behavior and Human Decision Processes 66: 316–32, https://doi.org/10.1006/obhd.1996.0059.
Kent, S. 1964. Words of estimative probability. Studies in Intelligence 8: 49–65, https://www.cia.gov/static/0aae8f84700a256abf63f7aad73b0a7d/Words-of-Estimative-Probability.pdf.
Keogh, L. 2011. Introducing the cane toad. Queensland Historical Atlas, 25 March 2011, https://www.qhatlas.com.au/introducing-cane-toad.
Kerr, N. and Tindale, R.S. 2011. Group-based forecasting?: A social psychological analysis. International Journal of Forecasting 27: 14–40, https://doi.org/10.1016/j.ijforecast.2010.02.001.
Klein, C.J., Jupiter, S and Possingham, H.P. 2014. Setting conservation priorities in Fiji: Decision science versus additive scoring systems Marine Policy 48: 204–05, https://doi.org/10.1016/j.marpol.2014.03.008.
Kluger, A.N. and DeNisi, A. 1996. The effects of feedback interventions on performance: A historical review, a meta-analysis, and a preliminary feedback intervention theory. Psychological Bulletin 119: 254–84, https://doi.org/10.1037//0033-2909.119.2.254.
Kopelman, R.E. 1986. Objective feedback. In: Generalizing From Laboratory to Field Settings, ed. by E.A. Locke (Lexington, MA: Lexington Books), pp.119–45.
Koriat, A., Lichtenstein, S. and Fischhoff, B. 1980. Reasons for confidence. Journal of Experimental Psychology-Human Learning and Memory 6: 107–18, https://doi.org/10.1037/0278-7393.6.2.107.
Kunda, Z. 1990. The case for motivated reasoning. Psychological Bulletin 108: 480–98, https://doi.org/10.1037/0033-2909.108.3.480.
Kuran, T. and Sunstein, C.R. 1999. Availability cascades and risk regulation. Stanford Law Review 51: 683–768, https://doi.org/10.2307/1229439.
Larkin, J., McDermott, J., Simon, D.P., et al. 1980. Expert and novice performance in solving physics problems. Science 208: 1335–42, https://doi.org/10.1126/science.208.4450.1335.
Larrick, R.P. and Soll, J.B. 2006. Intuitions about combining opinions: Misappreciation of the averaging principle. Management Science 52: 111–27,https://doi.org/10.1287/mnsc-1050.0459.
Lerner, J.S and Tetlock, P.E. 1999. Accounting for the effects of accountability. Psychological Bulletin 125: 255–75, https://doi.org/10.1037/0033-2909.125.2.255.
Lichtenstein, S. and Fischhoff, B. 1980. Training for calibration. Organizational Behavior and Human Performance 26: 149–71, https://doi.org/10.1016/0030-5073(80)90052-5.
Lin, S.W. and Bier, V.M. 2008. A study of expert overconfidence. Reliability Engineering & System Safety 93: 711–21, https://doi.org/10.1016/j.ress.2007.03.014.
Linstone, H.A. and Turoff, M. 1975. The Delphi Method: Techniques and Applications (Reading, MA: Addison-Wesley Publishing Company), https://web.njit.edu/~turoff/pubs/delphibook/index.html.
Mahoney, M.J. 1977. Publication prejudices: An experimental study of confirmatory bias in the peer review system. Cognitive Therapy and Research 1: 161–75, https://doi.org/10.1007/BF01173636.
Manski, C.F. 2006. Interpreting the predictions of prediction markets. Economics Letters 91: 425–29, https://doi.org/10.1016/j.econlet.2006.01.004.
Margalida, A., Martínez, J.M., Gómez de Segura, A., et al. 2017. Supplementary feeding and young extraction from the wild are not a sensible alternative to captive breeding for reintroducing bearded vultures Gypaetus barbatus. Journal of Applied Ecology 54: 334–40, https://doi.org/10.1111/1365-2664.12541.
Martin, T.G., Burgman, M.A., Fidler, F., et al. 2012. Eliciting expert knowledge in conservation science. Conservation Biology 26: 29–38, https://doi.org/10.1111/j.1523-1739.2011.01806.x.
McBride, M., Garnett, S.T., Szabo, J.K., et al. 2012. Structured elicitation of expert judgments for threatened species assessment: A case study on a continental scale using email. Methods in Ecology and Evolution 3: 906–20, https://doi.org/10.1111/j.2041-210X.2012.00221.x.
McKenzie, C., Liersch, M. and Yaniv, I. 2008. Overconfidence in interval estimates: What does expertise buy you? Organizational Behavior and Human Decision Processes 107: 179–91, https://doi.org/10.1016/j.obhdp.2008.02.007.
Mellers, B., Stone, E., Murray, T., et al. 2015. Identifying and cultivating superforecasters as a method of improving probabilistic predictions. Perspectives On Psychological Science: A Journal Of The Association For Psychological Science 10: 267–81, https://doi.org/10.1177/1745691615577794.
Mellers, B., Ungar, L., Baron, J., et al. 2014. Psychological strategies for winning a geopolitical forecasting tournament. Psychological Science 25: 1106–15, https://doi.org/10.1177/0956797614524255.
Mercier, H. and Claidière, N. 2022. Does discussion make crowds any wiser? Cognition 222: 104912, https://doi.org/10.1016/j.cognition.2021.104912.
Mercier, H. and Sperber, D. 2011. Why do humans reason? Arguments for an argumentative theory. Behavioral and Brain Sciences 34: 57–74, https://doi.org/10.1017/S0140525X10000968.
Mohanani, R., Salman, I., Turhan, B., et al. 2018. Cognitive biases in software engineering: A systematic mapping study. IEEE Transactions on Software Engineering 46: 1318–39, https://doi.org/10.1109/TSE.2018.2877759.
Mojzisch, A. and Schulz-Hardt, S. 2010. Knowing others’ preferences degrades the quality of group decisions. Journal of Personality and Social Psychology 98: 794–808, https://doi.org/10.1037/a0017627.
Moore, D.A. and Healy, P.J. 2008. The trouble with overconfidence. Psychological Review 115: 502–17, https://doi.org/10.1037/0033-295x.115.2.502.
Morgan, M.G. 2014. Use (and abuse) of expert elicitation in support of decision making for public policy. Proceedings of the National Academy of Sciences 111: 7176–84, https://doi.org/10.1073/pnas.1319946111.
Mukherjee, N., Hugé, J., Sutherland, W.J., et al. 2015. The Delphi technique in ecology and biological conservation: Applications and guidelines. Methods in Ecology and Evolution 6: 1097–109, https://doi.org/10.1111/2041-210x.12387.
Mukherjee, N., Zabala, A., Hugé, J., et al. 2018. Comparison of techniques for eliciting views and judgements in decision‐making. Methods in Ecology and Evolution 9: 54–63, https://doi.org/10.1111/2041-210X.12940.
Murphy, A.H. and Winkler, R.L. 1977. Can weather forecasters formulate reliable probability forecasts of precipitation and temperature? National Weather Digest 2: 2–9, http://nwafiles.nwas.org/digest/papers/1977/Vol02No2/1977v002no02-MurphyWinkler.pdf.
Navajas, J., Niella, T., Garbulsky, G., et al. 2017. Aggregated knowledge from a small number of debates outperforms the wisdom of large crowds. Nature Human Behaviour 2: 126–32, https://doi.org/10.1038/s41562-017-0273-4.
Newell, B.R., Weston, N.J., Tunney, R.J., et al. 2009. The effectiveness of feedback in multiple-cue probability learning. Quarterly Journal Of Experimental Psychology 62: 890–908, https://doi.org/10.1080/17470210802351411.
Nickerson, R.S. 1998. Confirmation bias: A ubiquitous phenomenon in many guises. Review of General Psychology 2: 175–220, https://doi.org/10.1037/1089-2680.2.2.175.
O’Hagan, A. 2019. Expert knowledge elicitation: Subjective but scientific. The American Statistician 79: 69–81, https://doi.org/10.1080/00031305.2018.1518265.
O’Reilly, C. and Chatman, J. 1986. Organizational commitment and psychological attachment: the effects of compliance, identification, and internalization on pro-social behavior. Journal of Applied Psychology 71: 492–99, https://doi.org/10.1037/0021-9010.71.3.492.
Oskamp, S. 1965. Overconfidence in case-study judgments. Journal of Consulting Psychology 29: 261–65, https://doi.org/10.1037/h0022125.
Page, S. 2007. The Difference: How the Power of Diversity Creates Better Groups, Firms, Schools, and Societies, New Edition (Princeton, NJ: Princeton University Press), https://doi.org/10.2307/j.ctt7sp9c.
Palan, S., Huber, J., and Senninger, L. 2020. Aggregation mechanisms for crowd predictions. Experimental Economics 23: 788–814, https://doi.org/10.1007/s10683-019-09631-0.
Perneger, T.V. and Agoritsas, T. 2011. Doctors and patients’ susceptibility to framing bias: A randomized trial. Journal of General Internal Medicine 26: 1411–17, https://doi.org/10.1007/s11606-011-1810-x.
Postmes, T., Spears, R. and Cihangir, S. 2001. Quality of decision making and group norms. Journal of Personality and Social Psychology 80: 918–30, https://doi.org/10.1037//0022-3514.80.6.918.
Puncochar, J.M. and Fox, P.W. 2004. Confidence in individual and group decision making: When ‘two heads’ are worse than one. Journal of Educational Psychology 96: 582–91, https://doi.org/10.1037/0022-0663.96.3.582.
Regan, H., Colyvan, M. and Burgman, M. 2002. A taxonomy and treatment of uncertainty for ecology and conservation biology. Ecological Applications 12: 618–28, https://doi.org/10.1890/1051-0761(2002)012[0618:ATATOU]2.0.CO;2.
Rothschild, D. and Wolfers, J. 2011. Forecasting elections: Voter intentions versus expectations. SSRN Electronic Journal, https://doi.org/10.2139/ssrn.1884644.
Rowe, G. and Wright, G. 1999. The Delphi technique as a forecasting tool: Issues and analysis. International Journal of Forecasting 15: 353–75, https://doi.org/10.1016/S0169-2070(99)00018-7.
Saposnik, G., Redelmeier, D., Ruff, C.C., et al. 2016. Cognitive biases associated with medical decisions: A systematic review. BMC Medical Informatics and Decision Making 16: Article 138, https://doi.org/10.1186/s12911-016-0377-1.
Satopää, V.A., Baron, J., Foster, D.P., et al. 2014. Combining multiple probability predictions using a simple logit model. International Journal of Forecasting 30: 344–56, https://doi.org/10.1016/j.ijforecast.2013.09.009.
Scholten, L., van Knippenberg, D., Nijstad, B.A., et al. 2007. Motivated information processing and group decision-making: Effects of process accountability on information processing and decision quality. Journal of Experimental Social Psychology 43: 539–52,http://dx.doi.org/10.1016/j.jesp.2006.05.010.
Schultze, T., Mojzisch, A. and Schulz-Hardt, S. 2012. Why groups perform better than individuals at quantitative judgment tasks: Group-to-individual transfer as an alternative to differential weighting. Organizational Behavior and Human Decision Processes 118: 24–36, https://doi.org/10.1016/j.obhdp.2011.12.006.
Schulz-Hardt, S., Brodbeck, F.C., Mojzisch, A., et al. 2006. Group decision making in hidden profile situations: Dissent as a facilitator for decision quality. Journal of Personality and Social Psychology 91: 1080–93, https://doi.org/10.1037/0022-3514.91.6.1080.
Schwenk, C.R. 1990. Effects of devil’s advocacy and dialectical inquiry on decision-making: A meta-analysis. Organizational Behavior and Human Decision Processes 47: 161–76, https://doi.org/10.1016/0749-5978(90)90051-a.
Shanteau, J. 1992. Competence in experts: The role of task characteristics. Organizational Behavior and Human Decision Processes 53: 252–66, https://doi.org/10.1016/0749-5978(92)90064-E.
Shanteau, J., Weiss, D.J., Thomas, R.P., et al. 2003. How can you tell if someone is an expert? Performance-based assessment of expertise. In: Emerging Perspectives on Judgment and Decision Research, ed. by S.L. Schneider and J. Shanteau (Cambridge: Cambridge University Press), pp.620–42, https://doi.org/10.1017/CBO9780511609978.021.
Siegel-Jacobs, K. and Yates, J.F. 1996. Effects of procedural and outcome accountability on judgment quality. Organizational Behavior and Human Decision Processes 65: 1–17, https://doi.org/10.1006/obhd.1996.0001.
Silver, I., Mellers, B.A. and Tetlock, P.E. 2021. Wise teamwork: Collective confidence calibration predicts the effectiveness of group discussion. Journal of Experimental Social Psychology 96: 104157, https://doi.org/10.1016/j.jesp.2021.104157.
Simon, H.A. 1977. The logic of heuristic decision making. In: Models of Discovery, Boston Studies in the Philosophy of Science, Vol LIV (Dordrecht: Springer), pp.154–75, https://doi.org/10.1007/978-94-010-9521-1_10.
Sniezek, J.A. 1992. Groups under uncertainty: An examination of confidence in group decision making. Organizational Behavior and Human Decision Processes 52: 124–55, https://doi.org/10.1016/0749-5978(92)90048-C.
Sniezek, J.A., Paese, P.W. and Switzer, F.S., III. 1990. The effect of choosing on confidence in choice. Organizational Behavior and Human Decision Processes 46: 264–82, https://doi.org/10.1016/0749-5978(90)90032-5.
Soll, J.B. and Klayman, J. 2004. Overconfidence in interval estimates. Journal of Experimental Psychology: Learning Memory and Cognition 30: 299–314, https://doi.org/10.1037/0278-7393.30.2.299.
Soll, J.B. and Larrick, R.P. 2009. Strategies for revising judgment: How (and how well) people use others’ opinions. Journal of Experimental Psychology: Learning, Memory, and Cognition 35: 780–805, https://doi.org/10.1037/a0015145.
Speirs-Bridge, A., Fidler, F., McBride, M., et al. 2010. Reducing overconfidence in the interval judgments of experts. Risk Analysis: An International Journal 30: 512–23, https://doi.org/10.1111/j.1539-6924.2009.01337.x.
Stasser, G. and Stewart, D. 1992. Discovery of hidden profiles by decision-making groups — Solving a problem versus making a judgment. Journal of Personality and Social Psychology 63: 426–34, https://doi.org/10.1037/0022-3514.63.3.426.
Stasser, G., Taylor, L.A. and Hanna, C. 1989. Information sampling in structured and unstructured discussions of 3-person and 6-person groups. Journal of Personality and Social Psychology 57: 67–78, https://doi.org/10.1037//0022-3514.57.1.67.
Stasser, G. and Titus, W. 1985. Pooling of unshared information in group decision-making: Biased information sampling during discussion. Journal of Personality and Social Psychology 48: 1467–78, https://doi.org/10.1037/0022-3514.48.6.1467.
Stasser, G. and Titus, W. 2003. Hidden profiles: A brief history. Psychological Inquiry 14: 304–13,https://doi.org/10.1207/s15327965pli1403&4_21.
Steiner, I.D. 1972. Group Process and Productivity. Social Psychology (New York: Academic Press).
Stewart, D.D., Billings, R.S. and Stasser, G. 1998. Accountability and the discussion of unshared, critical information in decision making groups. Group Dynamics: Theory, Research and Practice 2: 18–23, https://doi.org/10.1037//1089-2699.2.1.18.
Stewart, T.R., Roebber, P.J. and Bosart, L.F. 1997. The importance of the task in analyzing expert judgment. Organizational Behavior and Human Decision Processes 69: 205–19, https://doi.org/10.1006/obhd.1997.2682.
Surowiecki, J. 2004. The Wisdom of Crowds: Why the Many Are Smarter than the Few (London: Abacus).
Sutherland, W.J. and Burgman, M. 2015. Policy advice: Use experts wisely. Nature 526: 317–18, https://doi.org/10.1038/526317a.
Taber, C.S. and Lodge, M. 2006. Motivated skepticism in the evaluation of political beliefs. American Journal of Political Science 50: 755–69, https://doi.org/10.1111/j.1540-5907.2006.00214.x.
Teigen, K.H. and Jørgensen, M. 2005. When 90% confidence intervals are 50% certain: On the credibility of credible intervals. Applied Cognitive Psychology 19: 455–75, https://doi.org/10.1002/acp.1085.
Tetlock, P.E. 2005. Expert Political Judgment (Princeton, NJ: Princeton University Press).
Tetlock, P.E. and Gardner, D. 2016. Superforecasting: The Art and Science of Prediction (New York: Random House).
Thomas, E.J. and Fink, C.F. 1961. Models of group problem solving. Journal of Abnormal and Social Psychology 63: 53–63, https://doi.org/10.1037/h0040512.
Thorndike, E.L. 1920. A constant error in psychological ratings. Journal of Applied Psychology 4: 25–29, https://doi.org/10.1037/h0071663.
Trumbo, D., Adams, C., Milner, M., et al. 1962. Reliability and accuracy in the inspection of hard red winter wheat. Cereal Science Today 1: 62–71.
Tversky, A. and Kahneman, D. 1971. Belief in the law of small numbers. Psychological Bulletin 76: 105–10, https://doi.org/10.1037/h0031322.
Tversky, A. and Kahneman, D. 1973. Availability: A heuristic for judging frequency and probability. Cognitive Psychology 5: 207–32, https://doi.org/10.1016/0010-0285(73)90033-9.
Tversky, A. and Kahneman, D. 1974. Judgment under uncertainty: Heuristics and biases: Biases in judgments reveal some heuristics of thinking under uncertainty. Science 185: 1124–31, https://doi.org/10.1126/science.185.4157.1124.
Tversky, A. and Kahneman, D. 1983. Extensional versus intuitive reasoning: The conjunction fallacy in probability judgment. Psychological Review 90: 293–315, https://doi.org/10.1037/0033-295X.90.4.293.
Tversky, A. and Kahneman, D. 1985. The framing of decisions and the psychology of choice. In: Behavioral Decision Making (Boston, MA: Springer), pp.25–41, https://doi.org/10.1007/978-1-4613-2391-4_2.
Valacich, J.S., Jessup, L.M., Dennis, A.R., et al. 1992. A conceptual framework of anonymity in group support systems. Group Decision and Negotiation 1: 219–41, https://doi.org/10.1007/BF00126264.
Van de Ven, A. and Delbecq, A.L. 1971. Nominal versus interacting group processes for committee decision making effectiveness. Academy of Management Journal 14: 203–12, https://doi.org/10.5465/255307.
Vul, E. and Pashler, H. 2008. Measuring the crowd within: Probabilistic representations within individuals. Psychological Science 19: 645–47, https://doi.org/10.1111/j.1467-9280.2008.02136.x.
Wattanacharoensil, W. and La-ornual, D. 2019. A systematic review of cognitive biases in tourist decisions. Tourism Management 75: 353–69, https://doi.org/10.1016/j.tourman.2019.06.006.
Williams, J.J. and Mandel, D.R. 2007. Do evaluation frames improve the quality of conditional probability judgment? In: Proceedings of the 29th Annual Meeting of the Cognitive Science Society, ed. by D.S. McNamara and J.G. Trafton (Mahwah, NJ: Erlbaum), pp.1653–58, https://escholarship.org/uc/item/8vc9s8sm.
Winkler, R.L. 1967. Assessment of prior distributions in Bayesian analysis. Journal of the American Statistical Association 62: 776–800, https://doi.org/10.2307/2283671.
Winkler, R.L. and Murphy, A.H. 1968. ‘Good’ probability assessors. Journal of Applied Meteorology 7: 751–58, https://doi.org/10.1175/1520-0450(1968)007<0751:PA>2.0.CO;2.
Winman, A., Hansson, P. and Juslin, P. 2004. Subjective Probability intervals: How to reduce overconfidence by interval evaluation. Journal of Experimental Psychology-Learning Memory and Cognition 30: 1167–75, https://doi.org/10.1037/0278-7393.30.6.1167.
Wintle, B.C., Fidler, F., Vesk, P., et al. 2013. Improving visual estimation through active feedback. Methods in Ecology and Evolution 4: 53–62, https://doi.org/10.1111/j.2041-210x.2012.00254.x.
Wintle, B.C., Fraser, H., Wills, B.C., et al. 2019. Verbal probabilities: Very likely to be somewhat more confusing than numbers. PloS ONE 14: e0213522, https://doi.org/10.1371/journal.pone.0213522.
Wintle, B., Mody, F., Smith, E., et al. 2021. Predicting and reasoning about replicability using structured groups. MetaArXiv, 4 May 2021, https://doi.org/10.31222/osf.io/vtpmb.
Wolfers, J. and Zitzewitz, E. 2004. Prediction markets. Journal of Economic Perspectives 18: 107–26, https://doi.org/10.1257/0895330041371321.
Worchel, S., Wood, W. and Simpson, J.A. 1992. Group Process and Productivity (Newbury Park, CA: Sage Publications).
Yang, Y.-T.C. 2008. A catalyst for teaching critical thinking in a large university class in Taiwan: Asynchronous online discussions with the facilitation of teaching assistants. Educational Technology Research and Development 56: 241–64, https://doi.org/10.1007/s11423-007-9054-5.
Yaniv, I. 2011. Group diversity and decision quality: Amplification and attenuation of the framing effect. International Journal of Forecasting 27: 41–49, https://doi.org/10.1016/j.ijforecast.2010.05.009.
Yaniv, I. and Choshen-Hillel, S. 2012a. When guessing what another person would say is better than giving your own opinion: Using perspective-taking to improve advice-taking. Journal of Experimental Social Psychology 48: 1022–28, https://doi.org/10.1016/j.jesp.2012.03.016.
Yaniv, I. and Choshen-Hillel, S. 2012b. Exploiting the wisdom of others to make better decisions: Suspending judgement reduces egocentrism and increases accuracy. Journal of Behavioral Decision Making 25: 427–34, https://doi.org/10.1002/bdm.740.
Yaniv, I, Choshen-Hillel, S. and Milyavsky, M. 2009. Spurious consensus and opinion revision: Why might people be more confident in their less accurate judgments? Journal of Experimental Psychology-Learning Memory and Cognition 35: 558–63, https://doi.org/10.1037/a0014589.