17. Artificial Canaries: Early Warning Signs for Anticipatory and Democratic Governance of AI
© 2024 Carla Zoe Cremer & Jess Whittlestone, CC BY-NC 4.0 https://doi.org/10.11647/OBP.0360.17
Highlights:
- This chapter proposes a method for identifying early warning signs of transformative progress in Artificial Intelligence (AI), and discusses how these can support the anticipatory and democratic governance of AI. These early warning signs are called “canaries”, based on the use of canaries to provide early warnings of unsafe air pollution in coal mines.
- The author’s method combines expert elicitation and collaborative causal graphs to identify key milestones and the relationships between them. They present two illustrations of how this method could be used: to identify early warnings of harmful impacts of language models on political systems; and of progress towards high-level machine intelligence.
- Identifying early warning signs of transformative applications can support more efficient monitoring and timely regulation of progress in AI: as AI advances, its impacts on society may be too great to be governed retrospectively.
- It is essential that those impacted by AI have a say in how it is governed. Early warnings can give the public time and focus to influence emerging technologies using democratic, participatory processes.
This chapter was originally published in 2021 the International Journal of Interactive Multimedia & Artificial Intelligence. Like other contributions to this volume, it proposes the use of expert elicitation and the collaborative development of knowledge and understanding, in this case through the use of causal graphs. Methodological comparisons can be explored by reviewing Chapters 7, 8 or 16, whilst the core arguments concerning representation and democracy are also examined in different ways in Chapter 2 and 22.
I. Introduction
Progress in Artificial Intelligence (AI) research has accelerated in recent years. Applications are already changing society1 and some researchers warn that continued progress could precipitate transformative impacts.2 We use the term “transformative AI” to describe a range of possible advances with potential to impact society in significant and hard-to-reverse ways.3 For example, future machine learning systems could be used to optimise management of safety-critical infrastructure.4 Advanced language models could be used in ways that corrupt our online information ecosystem5 and future advances in AI systems could trigger widespread labour automation.6
There is an urgent need to develop anticipatory governance approaches to AI development and deployment. As AI advances, its impacts on society will become more profound, and some harms may be too great to rely on purely “reactive” or retrospective governance.
Anticipating future impacts is a challenging task. Experts show substantial disagreement about when different advances in AI capabilities should be expected.7 Policy-makers face challenges in keeping pace with technological progress: it is difficult to foresee impacts before a technology is deployed, but after deployment it may already be too late to shape impacts, and some harm may already have been done.8 Ideally, we would focus preventative, anticipatory efforts on applications which are close enough to deployment to be meaningfully influenced today, but whose impacts we are not already seeing. Finding “early warning signs” of transformative AI applications can help us to do this.
Early warning signs can also help democratise AI development and governance. They can provide time and direction for much-needed public discourse about what we want and do not want from AI. It is not enough for anticipatory governance to look out for supposedly “inevitable” future impacts. We are not mere bystanders in this AI revolution: the futures we occupy will be futures of our own making, driven by the actions of technology developers, policy-makers, civil society and the public. In order to prevent foreseeable harms towards those people who bear the effects of AI deployments, we must find ways for AI developers to be held accountable to the society which they are embedded in. If we want AI to benefit society broadly, we must urgently find ways to give democratic control to those who will be impacted. Our aim with identifying early warning signs is to develop anticipatory methods which can prompt a focussed civic discourse around significant developments and provide a wider range of people with the information they need to contribute to conversations about the future of AI.
We present a methodology for identifying early warning signs of potentially transformative impacts of AI and discuss how these can feed into more anticipatory and democratic governance processes. We call these early warning signs “canaries” based on the practice of using canaries to provide early warnings of unsafe air pollution in coal mines in the industrial revolution. Others before us have used this term in the context of AI to stress the importance of early warning signs9 but this is the first attempt to outline in detail how such “artificial canaries“ might be identified and used.
Our methodology is a prototype but we believe it provides an important first step towards assessing and then trialling the feasibility of identifying canaries. We first present the approach and then illustrate it on two high-level examples, in which we identify preliminary warning signs of AI applications that could undermine democracy, and warning signs of progress towards High-Level Machine Intelligence (HLMI). We explain why early warning signs are needed by drawing on the literature of Participatory Technology Assessments, and we discuss the advantages and practical challenges of this method in the hope of preparing future research that might attempt to put this method into practise. Our theoretical exploration of a method to identify early warning signs of transformative applications provides a foundation towards more anticipatory, accountable and democratic governance of AI in practice.
2. Related Work
We rely on two main bodies of work. Our methodology for identifying canaries relies on the literature on forecasting and monitoring AI. Our suggestions for how canaries might be used once identified build on work on Participatory Technology Assessments, which stresses a more inclusive approach to technology governance. While substantial research exists in both these areas, we believe this is the first piece of work that shows how they could feed into each other.
A. AI forecasting and monitoring
Over the past decade, an increasing number of studies have attempted to forecast AI progress. They commonly use expert elicitations to generate probabilistic estimates for when different AI advances and milestones will be achieved.10 For example, Baum et al. ask experts about when specific milestones in AI will be achieved, including passing the Turing Test or passing third grade.11 Both Müller and Bostrom12 and Grace et al.13 ask experts to predict the arrival of high-level machine intelligence (HLMI), which the latter define as when “unaided machines can accomplish every task better and more cheaply than human workers”.
However, we should be cautious about giving results from these surveys too much weight. These studies have several limitations, including the fact that the questions asked are often ambiguous, that expertise is narrowly defined, and that respondents do not receive training in quantitative forecasting.14 Experts disagree substantially about when crucial capabilities will be achieved,15 but these surveys cannot tell us who (if anyone) is more accurate in their predictions.
Issues of accuracy and reliability aside, forecasts focused solely on timelines for specific events are limited in how much they can inform our decisions about AI today. While it is interesting to know how much experts disagree on AI progress via these probabilistic estimates, they cannot tell us why experts disagree or what would change their minds. Surveys tell us little about what early warning signs to look out for or where we should place our focus today to shape the future development and impact of AI.
At the same time, several projects have begun to track and measure progress in AI.16 These projects focus on a range of indicators relevant to AI progress, but do not make any systematic attempt to identify which markers of progress are more important than others for the preparation of transformative applications. Time and attention for tracking progress is limited and it would be helpful if we were able to prioritise and monitor those research areas that are most relevant to mitigating risks.
Recognising some of the limitations of existing work, Gruetzemacher aims for a more holistic approach to AI forecasting.17 This framework emphasises the use of the Delphi technique18 to aggregate different perspectives of a group of experts, and cognitive mapping methods to study how different milestones relate to one another, rather than to simply forecast milestones in isolation. We agree that such methods might address some limitations of previous work in both AI forecasting and monitoring. AI forecasting has focused on timelines for particularly extreme events, but these timelines are subject to enormous uncertainty and do not indicate near-term warning signs. AI measurement initiatives have the opposite limitation: they focus on near-term progress, but with little systematic reflection on which avenues of progress are, from a governance perspective, more important to monitor than others. What is needed are attempts to identify areas of progress today that may be particularly important to pay attention to, given concerns about the kinds of transformative AI systems that may be possible in future.
B. Participatory Technology Assessments
Presently, the impacts of AI are largely shaped by a small group of powerful people with a narrow perspective which can be at odds with public interest.19 Only a few powerful actors, such as governments, defence agencies, and firms the size of Google or Amazon, have the resources to conduct ambitious research projects. Democratic control over these research projects is limited. Governments retain discretion over what gets regulated, large technology firms can distort and avoid policies via intensive lobbying20 and defence agencies may classify ongoing research.
Recognising these problems, a number of initiatives over the past few years have emphasised the need for wider participation in the development and governance of AI.21 In considering how best to achieve this, it is helpful to look to the field of science and technology studies (STS) which has long considered the value of democratising research progress.22 Several publications refer to the “participatory turn” in STS23 and an increasing interest in the role of the non-expert in technology development and assessment.24 More recently, in the spirit of “democratic experimentation”,25 various methods for civic participation have been developed and trialled, including deliberative polls, citizen juries and scenario exercises.26
With a widening conception of expertise, a large body of research on “participatory technology assessment” (PTA) has emerged, aiming to examine how we might increase civic participation in how technology is developed, assessed and rolled out. We cannot summarise this wide-ranging and complex body of work fully here. But we point towards some relevant pieces for interested readers to begin with. Biegelbauer and Loeber27 and Rowe and Frewer28 present a typology of the methods and goals of participating, which now come in many forms. This means that assessments of the success of PTAs are challenging29 and ongoing because different studies evaluate different PTA processes against different goals.30 Yet while scholars recognise remaining limitations of PTAs,31 several arguments for their advantages have been brought forward, ranging from citizen agency to consensus identification and justice. There are good reasons to believe that non-experts possess relevant end-user expertise. They often quickly develop the relevant subject-matter understanding to contribute meaningfully, leading to better epistemic outcomes due to a greater diversity of views which result in a cancellation of errors.32 To assess the performance of PTAs, scholars draw from case studies and identify best practices.33
There is an important difference between truly participatory, democratically minded, technology assessments, and consultations that use the public to help legitimise a preconceived technology.34 The question of how to make PTAs count in established representational democracies is an ongoing challenge to the field.35 But Hsaio et al., who present a recent example of collective technology policy-making, show that success and impact with PTAs is possible.36 Rask et al. draw from 38 international case studies to extract best practices,37 building on Joss and Bellucci,38 who showcase great diversity of possible ways in which to draw on the public. Comparing different approaches is difficult, but has been done.39 Burgess and Chilvers present a conceptual framework with which to design and assess PTAs,40 Ertiö et al. compare online versus offline methodologies41 and in Rowe and Frewer we find a typology of various design choices for public engagement mechanisms.42 See also, Rask for a helpful discussion on how to determine the diversity of participants;43 Mauksch et al. on what counts as expertise in foresight;44 and Lengwiler,45 Chilvers,46 and Saldivar et al.47 for challenges to be aware of in implementing PTAs.
Many before us have noted that we need wider participation in the development and governance of AI, including by calling for the use of PTAs in designing algorithms.48 We see a need to go beyond greater participation in addressing existing problems with algorithms and propose that wider participation should also be considered in conversations about future AI impacts.
Experts and citizens each have a role to play in ensuring that AI governance is informed by and inclusive of a wide range of knowledge, concerns and perspectives. However, the question of how best to marry expert foresight and citizen engagement is a challenging one. While a full answer to this question is beyond the scope of this chapter, what we do offer is a first step: a proposal for how expert elicitation can be used to identify important warnings which can later be used to facilitate timely democratic debate. For such debates to be useful, we first need an idea of which developments on the horizon can be meaningfully assessed and influenced, for which it makes sense to draw on public expertise and limited attention. This is precisely what our method aims to provide.
3. Identifying Early Warning Signs
We believe that identifying canaries for transformative AI is a tractable problem and worth investing research effort in today. Engineering and cognitive development present a proof of principle: capabilities are achieved sequentially, meaning that there are often key underlying capabilities which, if attained, unlock progress in many other areas. For example, musical protolanguage is thought to have enabled grammatical competence in the development of language in homo sapiens.49 AI progress so far has also seen such amplifiers: the use of multi-layered non-linear learning or stochastic gradient descent arguably laid the foundation for unexpectedly fast progress on image recognition, translation and speech recognition.50 By mapping out the dependencies between different capabilities needed to reach some notion of transformative AI, therefore, we should be able to identify milestones which are particularly important for enabling many others — these are our canaries.
The proposed methodology is intended to be highly adaptable and can be used to identify canaries for a number of important potentially transformative events, such as foundational research breakthroughs or the automation of tasks that affect a wide range of jobs. Many types of indicators could be of interest and classed as canaries, including algorithmic innovation that supports key cognitive faculties (e.g. natural language understanding); overcoming known technical challenges (such as improving the data efficiency of deep learning algorithms); or improved applicability of AI to economically-relevant tasks (e.g. text summarisation).
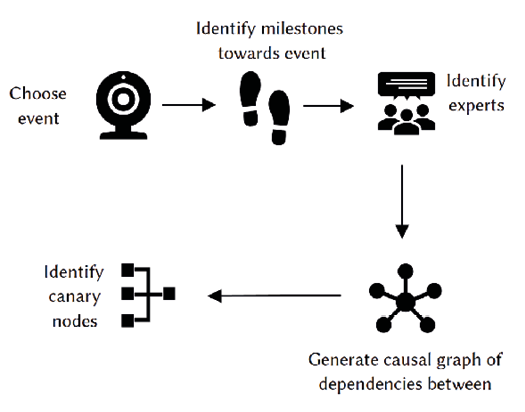
Fig. 1: Illustration of methodological steps to identify canaries of AI progress.
Given an event for which we wish to identify canaries, our methodology has three essential steps: (1) identifying key milestones towards the event; (2) identifying dependency relations between these milestones; and (3) identifying milestones which underpin many others as canaries. See Fig. 1 for an illustration. We here deliberately refrain from describing the method with too much specificity, because we want to stress the flexibility of our approach, and recognise that there is currently no one-size-fits-all approach to forecasting. The method will require adaptation to the particular transformative event in question, but each step of this method is suited for such specifications. We outline example adaptations of the method to particular cases.
A. Identifying milestones via expert elicitation
The first step of our methodology involves using traditional approaches in expert elicitation to identify milestones that may be relevant to the transformative event in question. Which experts are selected is crucial to the outcome and reliability of studies in AI forecasting. There are unavoidable limitations of using any form of subjective judgement in forecasting, but these limitations can be minimised by carefully thinking through the group selection. Both the direct expertise of individuals, and how they contribute to the diversity of the overall group, must be considered. See Mauksch et al. for a discussion of who counts as an expert in forecasting.51
Researchers should decide in advance what kinds of expertise are most relevant and must be combined to study the milestones that relate to the transformative event. Milestones might include technical limitations of current methods (e.g. adversarial attacks) and informed speculation about future capabilities (e.g. common sense) that may be important prerequisites to the transformative event. Consulting across a wide range of academic disciplines to order such diverse milestones is important. For example, a cohort of experts identifying and ordering milestones towards HLMI should include not only experts in machine learning and computer science but also cognitive scientists, philosophers, developmental psychologists, evolutionary biologists, or animal cognition experts. Such a group combines expertise on current capabilities in AI, with expertise on key pillars of cognitive development and the order in which cognitive faculties develop in animals. Groups which are diverse (on multiple dimensions) are expected to produce better epistemic outcomes.52
We encourage the careful design and phrasing of questions to enable participants to make use of their expertise, but refrain from demanding answers that lie outside their area of expertise. For example, asking machine learning researchers directly for milestones towards HLMI does not draw on their expertise. But asking machine learning researchers about the limitations of the methods they use every day — or asking psychologists what human capacities they see lacking in machines today — draws directly on their day-to-day experience.
Perceived limitations can then be transformed into milestones.
There are several different methods available for expert elicitation including surveys, interviews, workshops and focus groups, each with advantages and disadvantages. Interviews provide greater opportunity to tailor questions to the specific expert, but can be time-intensive compared to surveys and reduce the sample size of experts. If possible, some combination of the two may be ideal: using carefully selected semi-structured interviews to elicit initial milestones, followed-up with surveys with a much broader group to validate which milestones are widely accepted as being key.
B. Mapping causal relations between milestones
The second step of our methodology involves convening experts to identify causal relations between identified milestones: that is, how milestones may underpin, depend on, or affect progress towards other milestones. Experts should be guided in generating directed causal graphs, a type of cognitive map that elicits a person’s perceived causal relations between components. Causal graphs use arrows to represent perceived causal relations between nodes, which in this case are milestones.53
This process primarily focuses on finding out whether or not a relationship exists at all; how precisely this relationship is specified can be adapted to the goals of the study. An arrow from A to B at minimum indicates that progress on A will allow for further progress on B. But this relationship can also be made more precise: in some cases indicating that progress on AI is necessary for progress on B, for example. The relationship between nodes may be either linear or nonlinear; again, this can be specified more precisely if needed or known.
Constructing and debating causal graphs can “help groups to convert tacit knowledge into explicit knowledge”.54 Causal graphs are used as decision support for individuals or groups, and are often used to solve problems in policy and management involving complex relationships between components in a system by tapping into experts’ mental models and intuitions. We therefore suggest that causal graphs are particularly well-suited to eliciting experts’ models and assumptions about the relationship between different milestones in AI development.
As a method, causal graphs are highly flexible and can be adapted to the preferred level of detail for a given study: they can be varied in complexity and can be analysed both quantitatively and qualitatively.55 We neither exclude nor favour quantitative approaches here, due to the complexity and uncertainty of the questions around transformative events. Particularly for very high-level questions, quantitative approaches might not offer much advantage and might communicate a false sense of certainty. In narrower domains where there is more existing evidence, however, quantitative approaches may help to represent differences in the strength of relationships between milestones.
Eden notes that there are no ready-made designs that will fit all studies: design and analysis of causal mapping procedures must be matched to a clear theoretical context and the goal of the study.56 We highlight a number of different design choices which can be used to adapt the process. As more studies use causal graphs in expert elicitations about AI developments, we can learn from the success of different design choices over time and identify best practices.
Scavarda et al. stress that interviews or collective brainstorming are the most accepted method for generating the data upon which to analyse causal relations.57 Ackerman, Bryson, and Eden list heuristics on how to manage the procedure of combining graphs by different participants,58 or see Montibeller and Belton for a discussion on evaluating different options presented by experts.59 Scavarda et al. suggests visual, interactive tools to aid the process.60 Eden61 and Eden et al. 62 discuss approaches to analysing graphs and extracting the emergent properties, significant “core” nodes as well as hierarchical clusters. Core or “potent” nodes are those that relate to many clusters in the graphs and thus have implications for connected nodes. In our proposed methodology, such potent nodes play a central role in pointing to canary milestones. For more detail on the many options on how to generate, analyse and use causal graphs we refer the reader to the volume of Ackerman, Bryson, and Eden,63 or reviews such as Scavardia et al. (2004 and 2006).64 See Eden and Ackerman for an example of applying cognitive mapping to expert views on UK public policies,65 and Ackerman and Eden for group problem-solving with causal graphs.66
We propose that identified experts be given instruction in generating either an individual causal graph, after which a mediated discussion between experts generates a shared graph; or that the groups of experts as a whole generate the causal graph via argumentation, visualisations and voting procedures if necessary. As Eden emphasises, any group of experts will have both shared and conflicting assumptions, which causal graphs aim to integrate in a way that approaches greater accuracy than that contained in any single expert viewpoint.67 The researchers are free to add as much detail to the final maps as required or desired. Each node can be broken into subcomponents or justified with extensive literature reviews.
C. Identifying canaries
Finally, the resulting causal graphs can be used to identify nodes of particular relevance for progress towards the transformative event in question. This can be a node with a high number of outgoing arrows, i.e. milestones which unlock many others that are prerequisites for the event in question. It can also be a node which functions as a bottleneck — a single dependency node that restricts access to a subsequent highly significant milestone. See Fig. 2 for an illustration. Progress on these milestones can thus represent a “canary”, indicating that further advances in subsequent milestones will become possible and more likely. These canaries can act as early warning signs for potentially rapid and discontinuous progress, or may signal that applications are becoming ready for deployment. Experts identify nodes which unlock or provide a bottleneck for a significant number of other nodes (some amount of discretion from the experts/conveners will be needed to determine what counts as “significant”).
Of course, in some cases generating these causal graphs and using them to identify canaries may be as complicated as a full scientific research project. The difficulty of estimating causal relationships between future technological advances must not be underestimated. However, we believe it to be the case that each individual researcher already does this to some extent, when they chose to prioritise a research project, idea or method over another within a research paradigm. Scientists also debate the most fruitful and promising research avenues and arguably place bets on implicit maps of milestones as they pick a research agenda. The idea is not to generate maps that provide a perfectly accurate indication of warning signs, but to use the wisdom of crowds to make implicit assumptions explicit, creating the best possible estimate of which milestones may provide important indications of future transformative progress.
4. Using Early Warning Signs
Once identified, canary milestones can immediately help to focus existing efforts in forecasting and anticipatory governance. Given limited resources, early warning signs can direct governance attention to areas of AI progress which are soon likely to impact society and which can be influenced now. For example, if progress in a specific area of NLP (e.g. sentiment analysis) serves as a warning sign for the deployment of more engaging social bots to manipulate voters, policy-makers and regulators can monitor or regulate access and research on this research area within NLP.
We can also establish research and policy initiatives to monitor and forecast progress towards canaries. Initiatives might automate the collection, tracking and flagging of new publications relevant to canary capabilities, and build a database of relevant publications. They might use prediction platforms to enable collective forecasting of progress towards canary capabilities. Foundational research can try to validate hypothesised relationships between milestones or illuminate the societal implications of different milestones.
These forecasting and tracking initiatives can be used to improve policy prioritisation more broadly. For example, if we begin to see substantial progress in an area of AI likely to impact jobs in a particular domain, policy-makers can begin preparing for potential unemployment in that sector with greater urgency.
However, we believe the value of early warning signs can go further and support us in democratising the development and deployment of AI. Providing opportunities for participation and control over policy is a fundamental part of living in a democratic society. It may be especially important in the case of AI, since its deployment might indeed transform society across many sectors. If AI applications are to bring benefits across such wide-ranging contexts, AI deployment strategies must consider and be directed by the diverse interests found across those sectors. Interests which are underrepresented at technology firms are otherwise likely to bear the negative impacts.
There is currently an information asymmetry between those developing AI and those impacted by it. Citizens need better information about specific developments and impacts which might affect them. Public attention and funding for deliberation processes is not unlimited, so we need to think carefully about which technologies to direct public attention and funding towards. Identifying early warning signs can help address this issue, by focusing the attention of public debate and directing funding towards deliberation practises that centre around technological advancements on the horizon.
We believe early warning signs may be particularly well-suited to feed into Participatory Technology Assessments (PTAs), as introduced earlier. Early warning signs can provide a concrete focal point for citizens and domain experts to collectively discuss concerns. Having identified a specific warning sign, various PTA formats could be suited to consult citizens who are especially likely to be impacted. PTAs come in many forms and a full analysis of which design is best suited to assessing particular AI applications is beyond the scope of this article. But the options are plenty and PTAs show much potential (see Section 2). For example, Taiwan has had remarkable success and engagement with an open consultation of citizens on complex technology policy questions.68 An impact assessment of PTA is not a simple task, but we hypothesise that carefully designed, inclusive PTAs would present a great improvement over how AI is currently developed, deployed and governed. Our suggestion is not limited to governmental bodies. PTAs or other deliberative processes can be run by research groups and private institutions such as AI labs, technology companies and think tanks who are concerned with ensuring AI benefits all of humanity.
5. Method Illustrations
We outline two examples of how this methodology could be adapted and implemented: one focused on identifying warning signs of a particular societal impact, the other on warning signs of progress towards particular technical capabilities. Both these examples pertain to high-level, complex questions about the future development and impacts of AI, meaning our discussion can only begin to illustrate what the process of identifying canaries would look like, and what questions such a process might raise. Since the results are only the suggestions of the authors of this chapter, we do not show a full implementation of the method whose value lies in letting a group of experts deliberate. As mentioned previously, the work of generating these causal maps will often be a research project of its own, and we will return later to the question of what level of detail and certainty is needed to make the resulting graphs useful.
A. First illustration: AI applications in voter manipulation
We show how our method could identify warning signs of the kind of algorithmic progress which could improve the effectiveness of, or reduce the cost of, algorithmic election manipulation. The use of algorithms in attempts to manipulate election results incur great risk for the epistemic resilience of democratic countries.69
Manipulations of public opinion by national and commercial actors are not a new phenomenon. We detail the history of how newly emerging technologies are often used for this purpose. 70 But recent advances in deep learning techniques, as well as the widespread use of social media, have introduced easy and more effective mechanisms for influencing opinions and behaviour. Several studies detail the various ways in which political and commercial actors incur harm to the information ecosystem via the use of algorithms.71 Manipulators profile voters to identify susceptible targets on social media, distribute micro-targeted advertising, spread misinformation about policies of the opposing candidate and try to convince unwanted voters not to vote. Automation plays a large role in influencing online public discourse. Like et al.72 and Ferrara73 also note that manipulators use both human-run accounts and bots74 or a combination of the two.75 Misinformation76 and targeted messaging77 can have transformative implications for the resilience of democracies and the very possibility of collective action.78
Despite attempts by national and sub-national actors to apply algorithms to influence elections, their impact so far has been contested.79 Yet foreign actors and national political campaigns will continue to have incentives and substantial resources to invest in such campaigns, suggesting their efforts are unlikely to wane in future. We may thus inquire what kinds of technological progress would increase the risk that elections can be successfully manipulated. We can begin this inquiry by identifying what technological barriers currently prevent full-scale election manipulation.
We would identify those technological limitations by drawing on the expertise of actors who are directly affected by these bottlenecks. Those might be managers of online political campaigns and foreign consulting firms (as described in Howard),80 who specialise in influencing public opinion via social media, or governmental organisations across the world who comment on posts, target individual influencers and operate fake accounts to uphold and spread particular beliefs. People who run such political cyber campaigns have knowledge of what technological bottlenecks still constrain their influence on voter decisions. We recommend running a series of interviews to collect a list of limitations.
This list might include, for example, that the natural language functionality of social bots is a major bottleneck for effective online influence (for the plausibility of this being an important technical factor, see Howard).81 Targeted users often disengage from a chat conversation after detecting that they are exchanging messages with social bots. Low retention time is presumably a bottleneck for further manipulation, which suggests that improvements in Natural Language Processing (NLP) would significantly reduce the cost of manipulation as social bots become more effective.
We will assume, for the purpose of this illustration that NLP were to be identified as a key bottleneck. We would then seek to gather experts (e.g. in a workshop) who can identify and map milestones (or current limitations) in NLP likely to be relevant to improving the functionality of social bots. This will include machine learning experts who specialise in NLP and understand the technical barriers to developing more convincing social bots, as well as experts in developmental linguistics and evolutionary biology, who can determine suitable benchmarks and the required skills, and who understand the order in which linguistic skills are usually developed in animals.
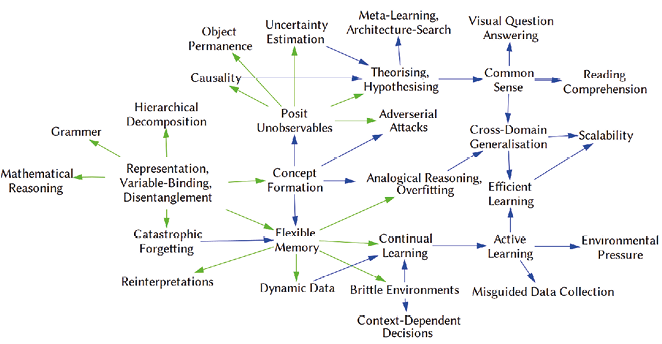
Fig. 2: Cognitive map of dependencies between milestones collected in expert elicitations. Arrows coloured in green signify those milestones that have most outgoing arrows. See appendix for description of each milestone and dependency relations between one “canary” node and subsequent nodes.
From these expert elicitation processes we would acquire a list of milestones in NLP which, if achieved, would likely lower the cost and increase the effectiveness of online manipulation. Experts would then order milestones into a causal graph of dependencies. Given the interdisciplinary nature of the question at hand, we suggest in this case that the graph should be directly developed by the whole group. A mediated discussion in a workshop context can help to draw out different connections between milestones and the reasoning behind them, ensuring participants do not make judgements outside their range of expertise. A voting procedure such as majority voting should be used if no consensus can be reached. In a final step, experts can highlight milestone nodes in the final graph which are either marked by many outgoing nodes or are bottlenecks for a series of subsequent nodes that are not accessed by an alternative pathway. These (e.g. sentiment analysis) are our canaries: areas of progress which serve as a warning sign of NLP being applied more effectively in voter manipulation.
Having looked at how this methodology can be used to identify warning signs of a specific societal impact, we next illustrate a different application of the method in which we aim to identify warning signs of a research breakthrough.
B. Second illustration: High-level Machine intelligence
We use this second example to illustrate in more detail what the process of developing a causal map might look like once initial milestones have been identified, and how canary capabilities can be identified from the map.
We define High-Level Machine Intelligence (HLMI) as an AI system (or collection of AI systems) that performs at the level of an average human adult on key cognitive measures required for economically relevant tasks. We choose to focus on HLMI since it is a milestone which has been the focus of previous forecasting studies82 and which, despite the ambiguity and uncertain nature of the concepts, is interesting to attempt to examine, because it is likely to precipitate widely transformative societal impacts.
To trial this method, we used interview results from Cremer (2021).83 25 experts from a diverse set of disciplines (including computer science, cognitive science and neuroscience) were interviewed and asked what they believed to be the main limitations preventing current machine learning methods from achieving the capabilities of HLMI. These limitations can be translated into “milestones”: capabilities experts believe machine learning methods need to achieve on the path to HLMI, i.e. the output of Step 1 of our methodology.
Having identified key milestones, Step 2 of our methodology involves exploring dependencies between them using causal graphs. We use the software VenSim to illustrate hypothesised relationships between milestones (see Fig. 2). For example, we hypothesise that the ability to formulate, comprehend and manipulate abstract concepts may be an important prerequisite to the ability to account for unobservable phenomena, which is in turn important for reasoning about causality. This map of causal relations and dependencies was constructed by the authors alone, and is therefore far from definitive, but provides a useful illustration of the kind of output this methodology can produce.
Based on this causal map, we can identify three candidates for canary capabilities:
- Representations that allow variable-binding and disentanglement: the ability to construct abstract, discrete and disentangled representations of inputs, to allow for efficiency and variable-binding. We hypothesise that this capability underpins several others, including grammar, mathematical reasoning, concept formation, and flexible memory.
- Flexible memory: the ability to store, recognise, and re-use memory and knowledge representations. We hypothesise that this ability would unlock many others, including the ability to learn from dynamic data, to learn in a continual fashion, and to update old interpretations of data as new information is acquired.
- Positing unobservables: the ability to recognise and use unobservable concepts that are not represented in the visual features of a scene, including numerosity or intentionality.
We might tentatively suggest that these are important capabilities to track progress on from the perspective of anticipating HLMI.
6. Discussion and Future Directions
As the two illustrative examples show, there are many complexities and challenges involved in putting this method into practice. One particular challenge is that there is likely to be substantial uncertainty in the causal graphs developed. This uncertainty can come in many forms.
Milestones that are not well understood are likely to be composed of several sub-milestones. As more research is produced, the graph will be in need of revision. Some such revisions may include the addition of connections between milestones that were previously not foreseen, which in turn might alter the number of outgoing connections from nodes and turn them into potent nodes, i.e. “canaries”.
The process of involving a diversity of experts in a multi-stage, collaborative process is designed to reduce this uncertainty by allowing for the identification of nodes and relationships that are widely agreed upon and so more likely to be robust. However, considerable uncertainty will inevitably remain due to the nature of forecasting. The higher the level of abstraction and ambiguity in the events studied (like events such as HLMI, which we use for our illustration) the greater the uncertainty inherent in the map and the less reliable the forecasts will likely be. It will be important to find ways to acknowledge and represent this uncertainty in the maps developed and conclusions drawn from them. This might include marking uncertainties in the graph and taking this into account when identifying and communicating “canary” nodes.
Given the uncertainty inherent in forecasting, we must consider what kinds of inevitable misjudgements are most important to try to avoid. A precautionary perspective would suggest it is better to slightly overspend resources on monitoring canaries that turn out to be false positives, rather than to miss an opportunity to anticipate significant technological impacts. This suggests we may want to set a low threshold for what should be considered a “canary” in the final stage of the method.
The uncertainty raises an important question: will it on average be better to have an imperfect, uncertain mapping of milestones rather than none at all? There is some chance that incorrect estimates of “canaries” could be harmful. An incorrect mapping could focus undue attention on some avenue of AI progress, waste resources or distract from more important issues.
Our view is that it is nonetheless preferable to attempt a prioritisation. The realistic alternative is that anticipatory governance is not attempted or informed by scholars’ individual estimates in an ad-hoc manner, which we should expect to be incorrect more often than our collective and structured expert elicitation. How accurate our method is can only be studied by trialling it and tracking its predictions as AI research progresses to confirm or refute the forecasts.
Future studies are likely to face several trade-offs in managing the uncertainty. For example, a large and cognitively diverse expert group may be better placed to develop robust maps eventually, but this may be a much more challenging process than doing it with a smaller, less diverse group — making the latter a tempting choice (see Rask for a discussion of this trade-off).84 The study of broad and high-level questions (such as when we might attain HLMI or automate a large percentage of jobs) may be more societally relevant or intellectually motivating, but narrower studies focused on nearer-term, well-defined applications or impacts may be easier to reach certainty on.
A further risk is that this method, intended to identify warning signs so as to give time to debate transformative applications, may inadvertently speed up progress towards AI capabilities and applications. By fostering expert deliberation and mapping milestones, it is likely that important research projects and goals are highlighted and the field’s research roadmap is improved. This means our method must be used with caution.
However, we do not believe this is a reason to abandon the approach, since these concerns must be balanced against the benefits of being able to deliberate upon and shape the impacts of AI in advance. In particular, we believe that the process of distilling information from experts in a way that can be communicated to wider society, including those currently underrepresented in debates about the future of AI, is likely to have many more benefits than costs.
The idea that we can identify “warning signs” for progress assumes that there will be some time lag between progress on milestones, during which anticipatory governance work can take place. Of course, the extent to which this is possible will vary, and in some cases, unlocking a “canary” capability could lead to very rapid progress on subsequent milestones. Future work could consider how to incorporate assessment of timescales into the causal graphs developed, so that it is easier to identify canaries which warn of future progress while allowing time to prepare.
Future work should also critically consider what constitutes relevant “expertise” for the task of identifying canaries, and further explore ways to effectively integrate expert knowledge with the values and perspectives of diverse publics. Our method finds a role for the expert situated in a larger democratic process of anticipating and regulating emerging technologies. Expert judgement can thereby be beneficial to wider participation. However, processes that allow more interaction between experts and citizens could be even more effective. One limitation of the method presented in this chapter is that it requires one to have already identified a particular transformative event of concern, but does not provide guidance on how to identify and prioritise between events. It may be valuable to consider how citizens that are impacted by technology can play a role in identifying initial areas of concern, which can then feed into this process of expert elicitation to address the concerns.
7. Conclusion
We have presented a flexible method for identifying early warning signs, or “canaries” in AI progress. Once identified, these canaries can provide focal points for anticipatory governance efforts, and can form the basis for meaningful participatory processes enabling citizens to steer AI developments and their impacts. Future work must now test this method by putting it into practice, which will more clearly reveal both benefits and limitations. Our artificial canaries offer a chance for forward-looking, democratic assessments of transformative technologies.
Acknowledgements
We thank reviewers for their particularly detailed comments and engagement with this chapter, the scholars at the Leverhulme Centre for the Future of Intelligence for fruitful discussions after our presentation, as well as the attendees of the workshop “Evaluating Progress in AI” at the European Conference on AI (August 2020) for recognising the potential of this work. We particularly thank Carolyn Ashurst and Luke Kemp for their efforts and commentary on our drafts.
Appendix available online at https://doi.org/10.11647/OBP.0360#resources
1 Crawford, K. et al. ‘AI Now Report 2019’, AI 2019 Report (2019), p. 100.
2 Russell, S. Human Compatible. Viking Press (2019); Cath, C., S. Wachter, B. Mittelstadt, M. Taddeo and L. Floridi. ‘Artificial Intelligence and the “good society”: The US, EU, and UK approach’, Sci. Eng. Ethics, 24(2) (April 2018): 505–28. https://doi.org/10.1007/s11948017-9901-7. Whittlestone, J., R. Nyrup, A. Alexandrova, K. Dihal and S. Cave. Ethical and Societal Implications of Algorithms, Data, and Artificial Intelligence: A Roadmap for Research (2019), p. 59. Dwivedi, Y. K. et al. ‘Artificial Intelligence (AI): Multidisciplinary perspectives on emerging challenges, opportunities, and agenda for research, practice and policy’, Int. J. Inf. Manag. (August 2019), p. 101994. https://doi.org/10.1016/j.ijinfomgt.2019.08.002
3 Gruetzemacher, R. and J. Whittlestone. ‘The transformative potential of Artificial Intelligence’, ArXiv191200747 Cs (September 2020). http://arxiv.org/abs/1912.00747
4 Brundage, M. et al., ‘The malicious use of Artificial Intelligence: Forecasting, prevention, and mitigation’, ArXiv180207228 Cs (February 2018). http://arxiv.org/ abs/1802.07228
5 Howard, P. Lie Machines, How to Save Democracy From Troll Armies, Deceitful Robots, Junk News Operations, and Political Operatives. Yale University Press (2020).
6 Frey, C. B. and M. A. Osborne. ‘The future of employment: How susceptible are jobs to computerisation?’, Technol. Forecast. Soc. Change, 114 (January 2017): 254–80. https://doi.org/10.1016/j.techfore.2016.08.019
7 Grace, J. K., J. Salvatier, A. Dafoe, B. Zhang and O. Evans. ‘Viewpoint: When will AI exceed human performance? Evidence from AI experts’, Artif. Intell. Res., 62 (July 2018): 729–54. https://doi.org/10.1613/jair.1.11222; Cremer, C. Z. ‘Deep limitations? Examining expert disagreement over deep learning’, Prog. Artif. Intell. Springer (to be published 2021).
8 Collingridge, D. The Social Control of Technology. Frances Pinter (1980).
9 Etzioni, O. ‘How to know if Artificial Intelligence is about to destroy civilization’, MIT Technology Review. https://www.technologyreview.com/s/615264/artificial-intelligence-destroy-civilization-canaries-robotoverlords-take-over-world-ai/; Dafoe, A. ‘The academics preparing for the possibility that AI will destabilise global politics’, 80,000 Hours (2018). https://80000hours.org/ podcast/episodes/allan-dafoe-politics-of-ai/
10 Grace et al. (2018); Müller, V. C. and N. Bostrom. ‘Future progress in Artificial Intelligence: A survey of expert opinion’, in Fundamental Issues of Artificial Intelligence, ed. V. C. Müller. Springer (2016), pp. 555–72; Baum, S. D., B. Goertzel and T. G. Goertzel. ‘How long until human-level AI? Results from an expert assessment’, Technol. Forecast. Soc. Change, 78(1) (January 2011): 185–95. https://doi.org/10.1016/j.techfore.2010.09.006; Beard, S., T. Rowe and J. Fox, ‘An analysis and evaluation of methods currently used to quantify the likelihood of existential hazards’, Futures, 115 (January 2020), p. 102469. https://doi.org/10.1016/j.futures.2019.102469
11 Baum et al. (2011).
12 Müller and Bostrom (2016).
13 Grace et al. (2018).
14 Cremer (2021); Tetlock, P. E. and D. Gardner. Superforecasting: The Art and Science of Prediction (1st edition). Crown Publishers (2015).
15 Grace et al. (2018).
16 E.g. Benaich, N. and I. Hogarth. State of AI Report 2020 (2020). https://www. stateof.ai/; Eckersley, P. and Y. Nasser. ‘AI progress measurement’, Electronic Frontier Foundation (12 June 2017). https://www.eff.org/ai/metrics; ‘Papers with code’. https://paperswithcode.com; Perrault, R. et al. ‘The AI Index 2019 annual report’, AI Index Steer. Comm. Hum.-Centered AI Inst. Stanf. Univ. Stanf. (2019).
17 Gruetzemacher. ‘A holistic framework for forecasting transformative AI’, Big Data Cogn. Comput., 3(3) (June 2019): 35. https://doi.org/10.3390/bdcc3030035
18 Linstone, H. A. and M. Turoff. The Delphi Method. Addison-Wesley Reading (1975).
19 West, S. M., M. Whittaker and K. Crawford. ‘Discriminating systems: Gender, race and power in AI’, AI Now Institute (2019). https://ainowinstitute.org/discriminatingsystems.html
20 Nemitz, P. and M. Pfeffer. Prinzip Mensch — Macht, Freiheit und Demokratie im Zeitalter der Künstlichen Intelligenz. Verlag J.H.W. Dietz Nachf. (2020).
21 Ipsos, M. ‘Public views of Machine Learning: Findings from public research and engagement conducted on behalf of the Royal Society’, The Royal Society (2017). https://royalsociety.org/-/ media/policy/projects/machine-learning/publications/public-views-ofmachine-learning-ipsos-mori.pdf; The RSA. ‘Artificial Intelligence: Real public engagement’, Royal Society for the Encouragement of Arts, Manufactures and Commerce (2018); Cohen, T., J. Stilgoe and C. Cavoli. ‘Reframing the governance of automotive automation: insights from UK stakeholder workshops’, J. Responsible Innov., 5(3) (September 2018): 257–79. https://doi.org/10.1080/23299460.2018.1495030
22 Lengwiler, M. ‘Participatory approaches in science and technology: Historical origins and current practices in critical perspective’, Sci. Technol. Hum. Values, 33(2) (March 2008): 186–200. https://doi.org/10.1177/0162243907311262; Rask, M. ‘The tragedy of citizen deliberation — Two cases of participatory technology assessment’, Technol. Anal. Strateg. Manag., 25(1) (January 2013): 39–55. https://doi.org/10.1080/09537325.2012.751012
23 Chilvers, J. ‘Deliberating competence: Theoretical and practitioner perspectives on effective participatory appraisal practice’, Sci. Technol. Hum. Values, 33(2) (March 2008): 155–85. https://doi.org/10.1177/0162243907307594
24 Ipsos (2017).
25 Abels, G. ‘Participatory technology assessment and the “institutional void”: Investigating democratic theory and representative politics’, in Democratic Transgressions of Law (vol. 112). Brill (2010), pp. 237–68.
26 Abels (2010).
27 Biegelbauer, P. and A. Loeber. ‘The challenge of citizen participation to democracy’, Inst. Für Höhere Stud. — Inst. Adv. Stud. IHS (2010), p. 46.
28 Rowe, G. and L. J. Frewer. ‘A typology of public engagement mechanisms’, Sci. Technol. Hum. Values, 30(2) (April 2005): 251–90. https://doi.org/10.1177/0162243904271724
29 Abels (2010).
30 Biegelbauer and Loeber (2010).
31 Rask (2013).
32 Hong, L. and S. E. Page. ‘Groups of diverse problem solvers can outperform groups of high-ability problem solvers’, Proc. Natl. Acad. Sci., 101(46) (November 2004): 16385–89. https://doi.org/10.1073/pnas.0403723101; Landemore, H. Democratic Reason. Princeton University Press (2017).
33 Joss, S. and S. Bellucci. Participatory Technology Assessment: European Perspectives. Center for the Study of Democracy (2002); Zhao, Y., C. Fautz, L. Hennen, K. R. Srinivas and Q. Li. ‘Public engagement in the governance of science and technology’, in Science and Technology Governance and Ethics: A Global Perspective From Europe, India and China, ed. M. Ladikas, S. Chaturvedi, Y. Zhao and D. Stemerding. Springer International Publishing (2015), pp. 39–51; Rask, M. T. et al. Public Participation, Science and Society: Tools for Dynamic and Responsible Governance of Research and Innovation. Routledge — Taylor & Francis Group (2018).
34 Burgess, J. and J. Chilvers. ‘Upping the ante: a conceptual framework for designing and evaluating participatory technology assessments’, Sci. Public Policy, 33(10) (December 2006): 713–28. https://doi.org/10.3152/147154306781778551
35 Rask (2013); Abels (2010).
36 Hsiao, Y. T., S.-Y. Lin, A. Tang, D. Narayanan and C. Sarahe. ‘vTaiwan: An empirical study of open consultation process in Taiwan’, SocArXiv (July 2018). https://doi.org/10.31235/osf.io/xyhft
37 Rask et al. (2018).
38 Joss and Bellucci (2002).
39 Zhao et al. (2015); Hansen, J. ‘Operationalising the public in participatory technology assessment: A framework for comparison applied to three cases’, Sci. Public Policy, 33(8) (October 2006): 571–84. https://doi.org/10.3152/147154306781778678
40 Burgess and Chilvers (2006).
41 Ertiö, T.-P., P. Tuominen and M. Rask. ‘Turning ideas into proposals: A case for blended participation during the participatory budgeting trial in Helsinki’, in Electronic Participation: ePart 2019 (Jul. 2019), pp. 15–25. https://doi.org/10.1007/978-3-030-27397-2_2
42 Rowe Frewer (2005).
43 Rask, M. ‘Foresight — Balancing between increasing variety and productive convergence’, Technol. Forecast. Soc. Change — TECHNOL FORECAST SOC CHANGE, 75 (October 2008): 1157–75. https://doi.org/10.1016/j.techfore.2007.12.002
44 Mauksch, S., H. A. von der Gracht and T. J. Gordon. ‘Who is an expert for foresight? A review of identification methods’, Technol. Forecast. Soc. Change, 154 (May 2020), p. 119982. https://doi.org/10.1016/j.techfore.2020.119982
45 Lengwiler (2008).
46 Chilvers (2008).
47 Saldivar, J., C. Parra, M. Alcaraz, R. Arteta and L. Cernuzzi. ‘Civic technology for social innovation: A systematic literature review’, Comput. Support. Coop. Work CSCW, 28(1–2) (April 2019): 169–207. https://doi.org/10.1007/s10606-018-9311-7
48 Kariotis, T. and J. Darakhshan. ‘Fighting back algocracy: The need for new participatory approaches to technology assessment’, in Proceedings of the 16th Participatory Design Conference 2020 — Participation(s) Otherwise — Volume 2. Manizales Colombia (June 2020), pp. 148–53. https://doi.org/10.1145/3384772.3385151; Whitman, M., C. Hsiang and K. Roark. ‘Potential for participatory big data ethics and algorithm design: A scoping mapping review’, Proceedings of the 15th Participatory Design Conference: Short Papers, Situated Actions, Workshops and Tutoriall — Volume 2 (August 2018), pp. 1–6. https://doi.org/10.1145/3210604.3210644
49 Buckner, C. and K. Yang. ‘Mating dances and the evolution of language: What’s the next step?’, Biol. Philos., 32 (2017). https://doi.org/10.1007/s10539017-9605-z
50 LeCun, Y., Y. Bengio and G. Hinton. ‘Deep learning’, Nature, 521(7553) (May 2015): 436–44. https://doi.org/10.1038/nature14539
51 Mauksch et al. (2020).
52 Landemore (2017); Page, S. E. The Difference: How the Power of Diversity Creates Better Groups, Firms, Schools and Societies. Princeton University Press (2008).
53 Scavarda, A. J., T. Bouzdine-Chameeva, S. M. Goldstein, J. M. Hays and A. V. Hill. ‘A review of the causal mapping practice and research literature’, in Abstract Number: 002-0256 (2004), p. 21.
54 Scavarda et al. (2004).
55 Markíczy, L. and J. Goldberg. ‘A method for eliciting and comparing causal maps’, J. Manag., 21(2) (January 1995): 305–33. https://doi.org/10.1016/0149-2063(95)90060-8; Eden, C. and F. Ackermann. ‘Cognitive mapping expert views for policy analysis in the public sector’, Eur. J. Oper. Res., 152(3) (February 2004): 615–30. https://doi.org/10.1016/S0377-2217(03)00061-4
56 Eden, C. On the Nature of Cognitive Maps (1992). https://doi.org/10.1111/j.1467-6486.1992.tb00664.x
57 Scavarda et al. (2004).
58 Ackerman, F., J. Bryson and C. Eden. Visible Thinking, Unlocking Causal Mapping for Practical Business Results. John Wiley & Sons (2004).
59 Montibeller, G. and V. Belton. ‘Causal maps and the evaluation of decision options — A review’, J. Oper. Res. Soc., 57(7) (July 2006): 779–91. https://doi.org/10.1057/palgrave.jors.2602214
60 Scavarda, A. J., T. Bouzdine-Chameeva, S. M. Goldstein, J. M. Hays and A. V. Hill. ‘A methodology for constructing collective causal maps*’, Decis. Sci., 37(2) (May 2006): 263–83. https://doi.org/10.1111/j.15405915.2006.00124.x
61 Eden (1992).
62 Eden, C., F. Ackermann and S. Cropper. ‘The analysis of cause maps’, J. Manag. Stud., 29(3) (1992): 309–24. https://doi.org/10.1111/j.1467-6486.1992.tb00667.x
63 Ackerman, Bryson and Eden (2004).
64 Scavardia et al. (2004); Scavardia et al. (2006).
65 Eden and Ackermann (2004).
66 Ackermann, F.and C. Eden. ‘Using causal mapping with group support systems to elicit an understanding of failure in complex projects: Some implications for organizational research’, Group Decis. Negot., 14(5) (September 2005): 355–76. https://doi.org/10.1007/s10726-005-8917-6
67 Eden, C. F. Ackermann, J. Bryson, G. Richardson, D. Andersen and C. Finn. Integrating Modes of Policy Analysis and Strategic Management Practice: Requisite Elements and Dilemmas (2009), p. 13.
68 Hsiao et al. (2018).
69 Neudert, L.-M. and P. Howard. ‘Ready to vote: Elections, technology and political campaigning in the United Kingdom’, Oxford Technology and Elections Commission (October 2019). https://apo.org.au/node/263976; Bolsover, G. and P. Howard. ‘Computational propaganda and political big data: moving toward a more critical research agenda’, Big Data, 5(4) (December 2017): 273–76. https://doi.org/czz; Mazarr, M. J., R. Bauer, A. Casey, S. Heintz and L. J. Matthews. The Emerging Risk of Virtual Societal Warfare: Social Manipulation in a Changing Information Environment (October 2019). https://www.rand.org/pubs/research_reports/ RR2714.html
70 Wu, T. The Attention Merchants: From the Daily Newspaper to Social Media, How Our Time and Attention is Harvested and Sold. Atlantic Books (2017).
71 Howard (2020); Starbird, K. ‘Disinformation’s spread: bots, trolls and all of us’, Nature, 571(7766) (July 2019): 449–50.
72 Like R. Gorwa and D. Guilbeault. ‘Unpacking the social media bot: A typology to guide research and policy’, Policy Internet, 12(2) (June 2020): 225–48. https://doi.org/10.1002/poi3.184
73 Ferrara, E. ‘Disinformation and social bot operations in the run up to the 2017 French Presidential Election’, Social Science Research Network (June 2017). https://doi.org/10.2139/ ssrn.2995809
74 Shao, C., G. L. Ciampaglia, O. Varol, K.-C. Yang, A. Flammini and F. Menczer. ‘The spread of low-credibility content by social bots’, Nat. Commun., 9(1) (November 2018). https://doi.org/10.1038/s41467-01806930-7
75 Howard, P. N., S. Woolley and R. Calo. ‘Algorithms, bots, and political communication in the US 2016 election: The challenge of automated political communication for election law and administration’, J. Inf. Technol. Polit., 15(2) (April 2018): 81–93. https://doi.org/10.1080/19331681.2018.1448735
76 Chessen, M. ‘The MADCOM future: How Artificial Intelligence will enhance computational propaganda, reprogram human culture, and threaten democracy… and what can be done about it’, Artificial Intelligence Safety and Security. Chapman and Hall/CRC Press (2018), pp. 127–44.
77 Kertysova, K. Artificial Intelligence and Disinformation: How AI Changes the Way Disinformation is Produced, Disseminated, and Can Be Countered (2018). https://doi.org/10.1163/18750230-02901005
78 Brainard, J. and P. R. Hunter. ‘Misinformation making a disease outbreak worse: Outcomes compared for influenza, monkeypox, and norovirus’, SIMULATION, 96(4) (April 2020): 365–74. https://doi.org/10.1177/0037549719885021; Seger, E., S. Avin, G. Pearson, M. Briers, S. O Heigeartaigh and H. Bacon. Tackling Threats to Informed Decision-Making in Democratic Societies: Promoting Epistemic Security in a Technologically Advanced World. Allan Turing Institute, CSER (2020). https://www.turing.ac.uk/sites/default/files/2020-10/epistemic-security-report_final.pdf
79 Jamieson, K. H. Cyberwar: How Russian Hackers and Trolls Helped Elect a President: What We Don’t, Can’t, and Do Know. Oxford University Press (2020).
80 Howard (2020).
81 Howard (2020).
82 Grace et al. (2018); Müller and Bostrom (2016).
83 Cremer (2021).
84 Rask (2008).