9. Artificial intelligence for good? Challenges and possibilities of AI in higher education from a data justice perspective
© 2023 Ekaterina Pechenkina, CC BY-NC 4.0 https://doi.org/10.11647/OBP.0363.09
Artificial intelligence technologies and methods have long been gaining traction in higher education, with accelerated growth in uptake and spread since the COVID-19 pandemic, including the 2023 rise of generative AI. However, even before the rapid evolution currently unfolding, AI-powered bots have already been widely used by universities, fielding student inquiries and delivering automated feedback in teaching and learning contexts. While this chapter acknowledges the groundbreaking changes currently wrought by generative AI technologies in HE, in particular in relation to assessment, it is primarily concerned with overarching principles and frameworks rather than with capturing the current rapidly-changing state of the tech industry. Among specific interests of this chapter is the use of AI tools by universities to predict students’ academic outcomes based on demographics, performance, and other data. The chapter explores whether and how AI brings benefits in the areas of student support and learning, and whether and how AI, as a symptom of HE’s massification, further complicates justice and equity issues. Drawing on scholarship dedicated to data justice and ethics of care, the chapter seeks to answer urgent questions associated with the proliferation of AI in HE: (a) how can AI be used in HE for good, (b) how can this rapidly growing industry be regulated, and (c) what would a conceptual framework for data justice and fair usage of AI in HE look like?
Introduction
My first experience with artificial intelligence (AI) in higher education (HE) dates to 2008 when I was employed on a short contract to work at a university administration. In my first week, my new manager came back excited and inspired from an overseas conference. There was one particular presentation that excited her the most: the one discussing how learning analytics and similar “automated” tools can identify students “at-risk” as early as the first day of the semester based on data students provide at enrolment, such as their postcode, whether they are first in the family to attend a university, and whatever other demographic data they are required to give throughout the application process (e.g. ethnicity, place of birth, whether they come from a refugee background or self-identify as Aboriginal and/or Torres Strait Islander — a collective term used by the Australian government for Australia’s First Nations peoples). Hearing this, I blurted out: “so, we will be racially profiling students?”. The manager did not react well to the remark. Long story short, my contract was not renewed. Naturally, the incident stayed with me over the years.
When over a decade later I responded to an invitation to contribute a chapter to the #HE4Good book, AI immediately came to mind. As a scholarship of teaching and learning (SoTL) researcher interested in the various ways technologies impact on and change teaching and learning practice and student experience, I am continually concerned with equity, justice, integrity, digital surveillance, and other salient issues associated with the proliferation of AI across the spheres of life.
A useful UNESCO publication offers an extensive list of possible applications of AI in HE, with a specific focus on generative AI. Among these possibilities are using AI as a guide, collaborative coach, motivator, assessor, and co-designer (Sabzalieva & Valentini, 2023). The same report also identifies a number of challenges such as academic integrity, accessibility, cognitive bias, and lack of regulation. None of these challenges are new. It is the latter issue in particular that this chapter is concerned with as it asks how we — educators, administrators, university leaders — can ensure that the inevitable propagation of AI technologies in our classrooms and wider HE spaces is indeed “for good”.
Drawing on scholarship around data justice (Dencik et al., 2019; Hoffmann, 2019; Taylor, 2017), ethics of care (Prinsloo, 2017; Prinsloo & Slade, 2017) and other relevant works, I analyse the phenomenon of AI in universities through the lens of social justice and in the wider context of datafication and massification of HE. I then make an argument for a data justice framework and principles that universities can — and should — use to guide their AI efforts to ensure that AI is indeed used for good. This chapter focuses on the superset of AI systems and tools on the conceptual level, rather than specifically on LLM (such as ChatGPT) as the matters of regulation and governance apply to a variety of AI applications.
Part critical review, part reflective piece, this chapter proposes an evidence-based roadmap for the future of AI governance in HE. It reiterates the urgent need for regulation and data justice in this field and proposes specific ways to enable AI practices that maximise the good for students, educators, universities, and communities.
Artificial intelligence is here to stay
Defined as “computer systems that undertake tasks usually thought to require human cognitive processes and decision-making capabilities” (Riedel et al., 2017, p. 1), AI technologies and methods entered HE’s lexicon about 30 years ago (Zawacki-Richter et al., 2019), yet AI is still positioned as an “emerging” field in HE. A recent Horizon report (Pelletier et al., 2021) identified AI among the six top technologies and practices expected to have a significant impact on the future of teaching and learning in tertiary education. The 2022 report (Pelletier et al., 2022) made a similar prediction. None of these predictions, however, truly accounted for the evolutionary leap that AI technologies took in 2023, with the rise of generative AI and large language models (LLMs) such as ChatGPT (What’s the next work in large language models?, 2023).
While AI technologies have much in common with the field of learner data measurement, collection and analysis collectively known as learning analytics (LA), AI in HE is swiftly evolving into a field of its own, encompassing a variety of methods and approaches, from machine learning to neural networks (Zawacki-Richter et al., 2019). Universities already use AI systems and methods in a variety of ways, such as administrative support provision (Sandu & Gide, 2019). Despite concerns around chatbots’ limited capacity to solve complex issues, combined with issues around privacy and exposure of personal information, the main selling point of chatbots to universities is that these technologies promise improved productivity and streamlined communications (Sandu & Gide, 2019).
There are other implied promises of “good” associated with AI integrations in HE. When seen through the lens of techno-optimism, defined as a consistent belief that science and technology can solve the various issues faced by our society (Alexander & Rutherford, 2019), such promises are typical of the edtech sector. And so, AI systems come to HE bearing “gifts”: from automating repetitive tasks that may not require human intervention, such as certain types of marking and assessing, providing feedback, responding to student queries (Zawacki-Richter et al., 2019), to such alleged benefits to teaching and learning as enhanced interactivity and personalised experiences for students in asynchronous online environments (Tanveer et al., 2020). This “automation is good” discourse is not new. In a 2021 book chronicling the history of so-called “teaching machines”, programmed devices designed to offer students personalised learning in the 1950s in accordance with B. F. Skinner’s controversial behaviourist theory, Audrey Watters outlines how Skinner’s (and Pressey’s before him) “innovations” in teaching and learning did not quite live up to their hype.
Other alleged benefits are associated with LA-centric applications of AI, which are tasked with helping educators and administrators understand how student online behaviour may be indicative of their academic outcomes (Herodotou et al., 2019). However, using AI for predicting human behaviour comes with loaded, and well-founded, concerns around equity and ethics (Kantayya, 2020; Lee, 2018), as well as data privacy and exploitation (Ouyang & Jiao, 2021; Schiff, 2021).
While the promise of automation and prediction may be appealing, such as for educators tasked with teaching large cohorts, faced with high student attrition or dealing with significant volumes of administrative work, the possibility of outsourcing such vital tasks as marking or feedback-giving to machines/algorithms may not sit well. Perhaps, some types of marking (e.g. multiple choice quiz or a highly structured essay) can indeed be automated, but as much as I would love to delegate my overflowing emails and student queries to an AI assistant, there remains a deep-seated sense of dread. sava saheli singh (2021) rightly points out that so-called “smart” technologies in education (where “smart” refers to the ability of a technology or a device to “make the decision so the person doesn’t have to think”) can “tak[e] away the ability of a teacher to connect meaningfully with their students” (p. 262–63). Even with its offer of a rapid response, can AI ever provide the same level of care to a student that a human educator would? And what about the various possibilities of misuse of these technologies, such as a recent case surrounding chatbot ChatGPT, with its use detected in one-fifth of student assessments (Cassidy, 2023b) or an even more recent example of an educator using AI software incorrectly to detect cheating, resulting in false accusation and withholding of grades (Klee, 2023)?
Despite mounting concerns, AI presence in universities is becoming ubiquitous, affecting multiple aspects of experience for students and staff. While some universities decided to ban the use of certain types of AI altogether, many others looked for smooth integration and effective usage while slowly revising their policies and governance frameworks. But as universities compete for students and resources, especially in the augmented post pandemic terrain characterised by shrinking budgets and austerity measures, it is not surprising if they turn increasingly to AI solutions. However, will this happen at the expense of student and staff privacy and digital rights? Chris Gilliard and other scholars working in this field issue legitimate warnings, including in relation to digital redlining — a digital equivalent of “historical form of societal division… that enforce class boundaries and discriminate against specific groups” (Gilliard, 2017, p. 64). The 2023 UNESCO report (Sabzalieva & Valentini, 2023) cites privacy concerns, commercialisation and, again, governance, as central challenges to overcome.
Issues around data ownership concerned with liability and accountability require extensive investigation, as many universities around the world use US-based educational technologies (such as learning management systems, or LMS). Similarly, the ongoing investment into AI technologies is also in the hands of ‘big tech’, which is dominated by US firms. This means student and staff data are likely to be stored on overseas servers, creating a legislative conundrum in cases of leaks and breaches, as well as issues associated with power relations.1 Further, there are related matters of accessibility, commercialisation and equity, given AI as an industry is profit-driven, and while its products may be free of cost at first they will likely end up behind paywalls eventually. Although non-for-profit alternatives to the likes of ChatGPT do exist, the lack of overarching governance policy and regulations creates many risks for universities, potentially allowing questionable practices to proliferate. However, such regulations are on the rise. Despite several countries banning or blocking ChatGPT (Sabzalieva & Valentini, 2023) and the CEO of OpenAI himself testifying in favour of regulation (Bhuiyan, 2023), these are far from widespread and many issues of uneven protections and access remain unaddressed.
In light of the rapid changes outlined, there is an urgent need for critical research, with practitioners and scholars coming together to provide evidence and inform regulation and governance design. In-depth understandings of AI’s impact on students and staff are essential. Maximum impact will be gained if theorists and practitioners work together to continue building this body of knowledge.
How AI is used in higher education
In their systematic review of research over the period 2007–2018, Zawacki-Richter et al. (2019) identify four main types of applications of AI in HE:
- Profiling/prediction,
- Assessment/evaluation,
- Adaptive systems/personalisation, and
- Intelligent tutoring systems.
The review outlines an assortment of possibilities afforded by AI, from using machine learning to predicting the likelihood of a student dropping out to providing just-in-time feedback to detecting plagiarism (Bahadir 2016; Luckin et al., 2016; Zawacki-Richter et al., 2019). A more recent review by Crompton and Burke (2023) offers a similar list of types of AI applications in HE, with the only new category added being AI assistants. “Self-supervised learning” which draws on the ability of AI systems to “learn from raw or non-labeled data” is touted as one of the most important relevant advances of AI relevant to HE (Mondelli, 2021, p. 13; Zhang et al., 2021). In a related conceptual discussion, Prinsloo (2017) proposes categorising the ways universities collect, analyse, and use student data as a matrix of seven dimensions: “automation; visibility; directionality; assemblage; temporality; sorting; and structuring” (p. 138).
AI systems can also be categorised based on tasks they perform across HE domains such as proctoring, office productivity, and admissions, and can be integrated into institutional learning management and student information systems and mobile apps used by students and staff (Pelletier et al., 2021). There are many specific examples of AI systems in action. A predictive algorithmic model tested by Delen (2011), for instance, demonstrated an accuracy of 81.19% when determining a student’s likelihood of dropping out, with factors such as previous academic achievement and the presence of financial support being key to determining their chances of success. It is not clear what happens next, but presumably with this information at their disposal, universities can “intervene” early and offer “at risk” students the help they need to stay enrolled. Whether students would accept such help and how effective it would be is less certain. My concern about using LA for racial profiling that in 2008 essentially cost me my job becomes salient again; my PhD research into the drivers of academic success of Indigenous Australian students revealed that “support” from the university was perceived very differently by Indigenous students depending on the way it was offered. When students felt singled out for “support” because of their Indigeneity, they rejected such offerings, finding them tokenistic and even stigmatising (Pechenkina, 2014, 2015).
In another predictive application of AI in HE, “sentiment analysis” using AI algorithmic capabilities can determine negative and positive attitudes in student social media posts about a particular course and based on that, make judgements about student experiences (Pham et al., 2020). Perhaps a less controversial example, AI systems and capabilities can also be used to understand how students self-regulate learning — their metacognition skills — and ways to scaffold and facilitate those in personalised ways (Pelletier et al., 2021).
AI technologies can be used to evaluate the content of student assignments (automated marking), identifying topics covered in essays, engaging students in a dialogue about their learning progression, and offering support and resources to help them achieve their learning goals. Pelletier et al. (2021) provide several examples of the latter, including various chatbots that can enable student language practice with a virtual avatar which delivers “natural responses” to students.
Other examples of utilising AI in HE have to do with latent semantic analysis or semantic web technologies which can “inform personalised learning pathways for students” by evaluating and verifying recognition of prior learning (RPL) and converting credits and credentials obtained by students elsewhere to count toward their degree (Zawacki-Richter et al. 2019, p. 17). It is argued that in addition to saving time and money, these affordances of AI may increase students’ employability by helping them match their skills and competencies with requirements of the workplace. Similar to intelligent tutoring systems, AI tools used as digital assistants can support student learning by posing diagnostic questions and guiding students toward accessing resources relevant to their needs (Crompton & Burke, 2023).
At the time of writing, the media discourse surrounding AI tools in HE, specifically generative AI, has been both alarming and alarmist, with a torrent of articles outlining the documented or alleged misuse of bots like ChatGPT by students. Commonly used by copywriters, lawyers, and other professionals to generate website content, legal briefs and so on, bot-generated text has been detected in university students’ written assignments (Cassidy, 2023b). However, the discourse quickly shifted to discussing practical steps forward, such as assessment redesign and re-thinking the matter of governance around academic integrity and the use of AI.
Concerns around assessment are not new, with Pelletier et al. (2021) arguing for the need to re-think assessment to “better serve ‘generation AI’” (p. 13). Assessment could be redesigned to reduce opportunities for students to use text-generating bots and to rely on their critical thinking and reflection skills instead, while the way examinations are run would also need change, with some universities already reverting to “pen and paper” exams (Cassidy, 2023a). Considering this, it is troubling that of the sample analysed by Zawacki-Richter et al. (2019), only two out of 146 articles (1.4% of the sample) engaged critically with issues relating to ethics and risks that come with AI applications in HE. This apparent scarcity of critical perspectives in practitioners’ research suggests a prevalence of techno-centric, uncritical implementations of AI technologies in HE, which can produce more harm than good.
In addition to the issues outlined above, there are many more serious risks associated with AI in HE, ranging from those posed to students and educators due to unconscious bias affecting the fairness of algorithmic decisions and the misuse of private data, to potential loss of academic and administrative/professional support jobs. Further risks include harm to workers as well as climate effects. Lack of algorithm transparency constitutes an ongoing challenge, likely to disproportionately affect those who may already be vulnerable and disempowered (Kantayya, 2020; Lee, 2018), as Gilliard’s (2017) work on surveillance and digital redlining highlights. Further, Buolamwini and Gebru (2018) note the potential of machine learning algorithms to discriminate based on race and gender among other classes, offering ways to alleviate these biases.
Pedagogically-led implementation of AI in teaching and learning remains an underexplored area in peer reviewed literature (Zawacki-Richter et al., 2019), indicative of a divide that persists between practitioners’ drive for technological innovation and the pedagogical rationale behind it. These and other challenges associated with AI in HE are discussed in the next section, which brings to light important criticisms before offering a way forward.
Challenges and risks of artificial intelligence in higher education
Ethics, privacy and other issues associated with AI practices in HE were rarely foregrounded in the studies reviewed by Zawacki-Richter et al. (2019), with rare exceptions (Li, 2007; Welham, 2008). Li (2007), acknowledging that when using automated systems to deliver support or teaching, students might be worried about possibilities of discrimination when their personal data was accessed, while Welham (2008) was primarily concerned with the cost and affordability of AI applications for publicly funded universities which may not be able to compete with their wealthier counterparts.
However, there is a promising rise of diverse, critical voices that challenge the techno-centric and techno-optimistic accounts which exalt the technical affordances and possibilities of AI (and edtech more generally) while brushing over (or wilfully ignoring) the deeper concerns over privacy, equity, profiling and other serious risks and challenges. For example, contrasting the earlier promises of increased productivity and freeing up of educators’ time via automating “routine” tasks, Mirbabaie et al. (2022) highlight how the integration of AI systems into day-to-day university life may also have legal implications for workloads and enterprise bargaining agreements which are designed to protect staff and jobs. Further, when it comes to students, the central narrative maintained by edtech companies that sell surveillance and other AI-powered products to universities is that cheating is on the rise and students cannot be trusted (Swauger, 2020). While the evidence behind such trends is not so clear-cut (Newton, 2018), it is suggested that the increase in student cheating observed over the decades “may be due to an overall increase in self-reported cheating generally, rather than contract cheating specifically” (p. 1). What is more concerning, however, is how bodies and behaviours of students are categorised by the AI surveillance systems. As Swauger (2020) observes, “cisgender, able-bodied, neurotypical, [male, and] white” bodies are “generally categorized… as normal and safe” by these technologies, hence there is little risk of jeopardising such bodies’ academic or professional standings. Bodies that do not share these characteristics, however, may not fare so well.
Analysing the dilemmas surrounding AI, surveillance and algorithmic decision-making in education, Prinsloo (2017) warns that ethical considerations must be prioritised and negotiated in this complicated terrain where human and nonhuman actors interact. Other scholars also employ critical perspectives to argue that antiracist, equity, and privacy principles must be embedded into any policies concerned with using AI systems in HE to reduce harm and not to disenfranchise and disempower students and/or educators (Ouyang & Jiao, 2021; Schiff, 2021). Discussing what it means for AI to truly empower human actors, Ouyang and Jiao (2021) theorise empowerment as a conceptual movement from the dominant paradigm in which learners are recipients of AI-directed teaching and support, toward a paradigm which sees learners as leaders directing AI action within complex educational terrains. The importance of ethical considerations in the latter scenario is implied.
Among the most significant challenges associated with the use of AI in HE are those related to teaching and learning. Analysing AI applications in so-called intelligent tutoring services, Zawacki-Richter et al. (2019) located four main types of their use:
- Teaching/delivering content,
- Diagnosing strengths/gaps in students’ knowledge; providing automated feedback
- Curating resources and materials based on student need, and
- Facilitating collaboration.
An alarming finding of this review indicates a scarcity of research that mindfully applies educational theories and pedagogical foundations to inform AI decisions in teaching and learning. Only a handful of studies were identified where educational theory and pedagogical thinking were apparent in AI designs. Among these were the two Barker (2010, 2011) studies, which drew on Bloom’s taxonomy and cognitive levels when designing automated feedback systems for adoptive testing modelling. Other examples discussed developing AI solutions to enable learning progression support with intelligent tutoring systems. Arguably, these and similar practices can benefit immensely from robust theorising, for example, by bringing Vygotskian ideas about learning and development into online and hybrid spaces (Hall, 2007). Ouyang and Jiao’s 2021 review reinforces this need, highlighting that many of the above-mentioned issues persist and pedagogical theories underpinning AI-based learning and instruction are still rare to find in AI-focused HE studies.
A specific set of challenges associated with AI in HE relates to the use of chatbots and similar mechanisms to resolve student inquiries, provide feedback, assess students’ work, and perform other types of automated or semi-automated tasks. While a deeper understanding of costs and “return on investment” is needed, there is perhaps a potential for bots to save universities time and money, for example, by using bot-enabled apps to understand student experiences (and challenges) and use this knowledge to reduce attrition (Nietzel, 2020). However, the increasing use of bots may also be indicative of massification and commercialisation of HE, where students are “customers” or “users” rather than learners. This is troubling as, I would argue, it further increases the distance between students and learning and between students and educators, potentially isolating and disenfranchising some students and further marginalising those who might already be disadvantaged. Peer reviewed research into bot-assisted support and teaching, especially from a student perspective, remains scarce, while challenges associated with using AI bots in student support require serious exploration, with quality (Pérez et al., 2020) and security (Hasal et al., 2021) being particularly salient issues.
While scholars of AI and educational technologies more generally (Facer & Selwyn, 2021; Ferguson, 2019; Selwyn & Gašević, 2020) argue in favour of prioritising ethical and pedagogically-sound approaches to designing and deploying AI tools in HE, prior to the rise of generative AI, university leadership appeared overly preoccupied with using AI for surveillance and student outcome prediction, focusing on early identification of “students-at-risk”. While these goals are still very much present, the current discourse has shifted to deal with regulating the use of generative AI by students and staff. Relevant discussions can be found in scholarship dedicated specifically to LA, with Guzmán-Valenzuela et al. (2021) and other authors warning of a divide that persists between practice-based and management-oriented applications of LA in HE. With AI’s proliferation across HE, challenges and risks associated with ethics, privacy and related issues deserve a deeper exploration — and with the possibilities of generative AI, these concerns are more important than ever.
Ethics, privacy, and data justice
Data justice discourses highlight important privacy and digital surveillance concerns, such as the potential misuse of data and the quality of services and teaching provided to students. These issues become particularly problematic when juxtaposed with the idea of HE as a “public good” (Marginson, 2011) along with its stated noble goals, such as students’ personal development, reducing inequality, and tackling other societal challenges (Bowen & Fincher, 1996).
Specific risks to student privacy are associated with the use of AI-enabled surveillance in examination and proctoring practices (Pelletier et al., 2021). Chin (2020) and Clark (2021) chronicle one such case of digital proctoring, where a university staff member faced litigation after publicly raising concerns about the practice and the software. At the heart of the case is the evidence-based concern that using an AI software to proctor online examinations caused students emotional harm by tracking their private spaces using built-in cameras, deploying abnormal eye movement function as well as other invasive technologies to determine when students were not looking at their test. Such student behaviours were labelled problematic, indicating signs of cheating. However, the software did not account for neurodivergent students and those with physical and learning disabilities, raising the concerns of discrimination. While teachers could choose not to use the software, it was not clear what alternative methods of remote proctoring were available to them. It was also not clear whether students could opt out from this practice without harming their standing in the university. It comes as little surprise that students are speaking up against automated proctoring, online tracking, and other types of surveillance (Feathers & Rose, 2020), calling it out as ableist, discriminatory, and intrusive (Chin, 2020; Gullo, 2022).
Text-matching platforms used by universities to detect plagiarism and other misconduct offer another example of automated surveillance that has become ubiquitous in HE. Mphahlele and McKenna (2019) decode several myths surrounding one such platform widely used by universities (at the time of the study’s publication, it was being used by 15,000 HE institutions in over 140 countries). The most common myth alarmingly has to do with the software’s perceived core function: while it is a misconception that it detects plagiarism, this myth continues to popularise this text-matching product among universities and beyond. Software like this is used primarily to police student behaviour rather than for educational or developmental purposes. I argue that such uncritical, routine use of surveillance software on students feeds into the overall culture of surveillance that has become normalised at universities and other workplaces.2
While specific university policies guide institutional efforts relating to academic integrity and so-called contract cheating, as Stoesz et al. (2019) point out, these policies often lack “specific and direct language”, their principles are not clearly defined, and overall, such policies are often underdeveloped. Whether or not universities mandate the use of such platforms, the choice of usage is often left with individual teaching academics. Once activated, one widely used text-matching platform automatically produces a colour-coded “similarity score” students can preview. Academics can view a “similarity report” once the assignment is submitted, indicating where text in the student assignment matches text published elsewhere. All submissions processed in this way are digitally stored in a repository owned by the company that owns the software.3 It is ironic that tools meant to uphold academic integrity in turn collect students’ work and sell it for profit (Morris & Stommel, 2017). While students and academics can request that individual papers be removed, this process can take time. At the same time, academics can request to see relevant assignments submitted elsewhere to analyse a piece under investigation for cheating. Depending on a university’s academic misconduct policy, students can face penalties, such as suspension or exclusion.4 While similarity checks may be beneficial to students, helping them develop a stronger sense of integrity and become better writers, they come with risks and punishments in stock. Student consent is implied here but it is not fully informed — throughout their studies students remain largely unaware of what data generated by their actions is gathered, how it is used, or how they can opt out.
Considering the threat of lawsuits and persecution of whistle-blowers and critics (Chin, 2020), clear university-level frameworks to govern the use of AI are necessary if universities are serious about their promises in relation to students’ and faculty’s wellbeing. Moreso, such frameworks must go hand in hand with protection offered to staff and students who speak up about their experiences and offer critiques, holding those in power accountable.
Professional development and upskilling of staff, as well as students, is another critical challenge to tackle alongside ethical AI integrations into the HE. In such a task, principles of data justice informed by empathy, antiracist philosophy, ethics of care, and trauma-informed teaching must take centre stage to ensure AI technologies do no harm.
A data justice framework for artificial intelligence in higher education
Data justice is an important dimension of the debate surrounding the ethics of using AI in HE. Explored by Dencik et al. (2019), Prinsloo and Slade (2017), Taylor (2017) and others, data justice can be understood as a dimension of the broader social justice discourse, concerned specifically with datafication and digital rights and freedoms in the context of datafied society. Data justice presents a useful framework “for engaging with… challenges [associated with datafication] in a way that privileges an explicit concern for social justice” (Dencik & Sanchez-Monedero, 2022, p. 2) as data-driven discrimination can take place whenever data is collected (Kantayya, 2020). A “fairness in the way people are made visible, represented and treated as a result of their production of digital data” Taylor (2017, p. 1), as explained earlier in the chapter, data justice is urgently needed in HE the same way it is needed in all other domains of datafied society.
Student and staff anxieties around intrusive surveillance and data-based profiling should be centred when designing fair and equitable AI solutions. This is particularly important in online and hybrid environments which attract large and diverse cohorts and where personalised student experiences are not always possible without technological interventions.
The establishment of specialised institutes and advisory groups tasked with producing ethical frameworks and policies for governance of AI in HE, like the UK’s now-defunct Institute for Ethical AI in Education,5 Germany’s state-funded project AI Campus,6 Australia’s Data61, Hong-Kong-based Asia-Pacific Artificial Intelligence Association,7 and other similar formations, indicates a concerted move toward a unifying approach in this field, at least at national levels.
The framework and principles presented below (see Figure 9.1) are a synthesis of recommendations developed by other scholars and practitioners. It is proposed that universities use these principles when developing institutional policies for AI, to ensure that all implementations of AI are fair, transparent, and just.
Figure 9.1
Conceptual framework for principles for AI governance in HE8
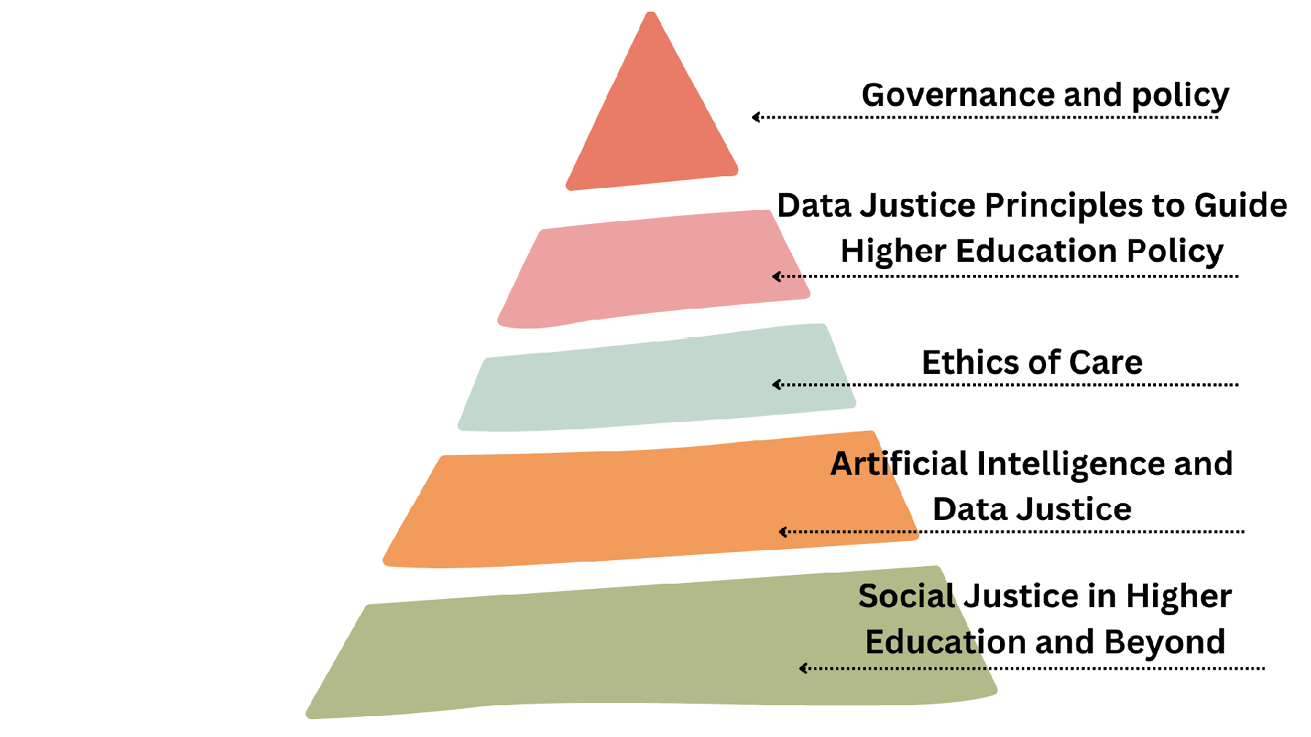
Data justice-based principles for AI governance in HE:
- Transparency: to offer upfront information to students and staff about what data is collected and how it will be used.
- Clarity: to spell out rationale (pedagogical and/or otherwise) for all AI solutions affecting students and staff and explain in plain language why this data is collected.
- No harm: to embed into AI designs measures against harmful profiling, e.g. data about students’ ethnicity, for example, could be hidden/not made available to algorithms unless there is a strong rationale for its inclusion.
- Agency: to allow students and staff to actively exercise their right to opt out and withdraw their data without prejudice.
- Active governance: to set up a meaningful institutional entity to handle complaints and other issues of relevance to AI. A dedicated ethics committee could be set up and populated by members who are up to date on these issues. Any such committee must include student representatives and social justice advocates.
- Accountability: to consider AI’s expected benefits against estimated risks, with mitigation strategies put in place as well as reporting processes embedded to ensure accountability and transparency to the public.
Ethical principles currently found in peer reviewed research are primarily concerned with LA and using data for prediction of outcomes, such as principles developed by Corrin et al. (2019), which include privacy, data ownership, transparency, consent, anonymity, non-maleficence, security, and access. An excellent example of university-level framework for the ethical use of student data comes from Athabasca University, highlighting such principles as Supporting and Developing Learner Agency, Duty of Care, Transparency and Accuracy, and others.9 However, most of these, like the OECD principles,10 are non-binding recommendations, which limits their reach and impact. Importantly, with some exceptions (Jones et al., 2020), meaningful staff and student voices tend to be missing from these important discussions altogether.
Among the conceptual works informing the proposed framework is Prinsloo’s (2017) matrix explaining four main AI-performed processes in education and which focuses on the shifting responsibility between algorithmic and human actors. The matrix is presented as a spectrum of possibilities based on the presence of human agency, starting from tasks performed solely by humans and ending with tasks performed fully by algorithms without human oversight and intervention. Two in-between possibilities included tasks shared between humans and machines and tasks performed by algorithms with human supervision.
Commissioned by the Australian Government, Dawson et al.’s (2019) discussion paper is also relevant to the above framework and principles. It identifies trust as a key principle when integrating AI solutions and systems, regardless of industry. The paper solicited feedback regarding AI ethics, receiving 130 submissions from government, business, academia, and the non-government sector and from individuals. As a result, the following eight principles emerged as important:
- Wellbeing
- Human-centred values
- Fairness
- Privacy protection and security
- Reliability and safety
- Transparency and explain-ability
- Contestability
- Accountability
These principles are voluntary, offered as guidance for businesses and other stakeholders wishing to exercise high ethical standards in their work with AI. The main consequence of this is that it is left to the discretion of organisations whether to follow these guidelines or not, which makes it difficult to assign responsibility and accountability. Among the case studies submitted in response to Dawson et al. (2019), none came from HE or the wider education sector. Among the recommendations produced were formation of advisory groups and review panels tasked with guiding the organisation’s leadership in responsible AI use, reviewing sensitive cases and complaints, and championing ethical use across smaller teams. The overall need for training and useful exemplars was also identified as essential (Dawson et al., 2019).
Among the case studies in Dawson et al. (2019) was one by Microsoft,11 which focused on the ethical and safe use of chatbots. Key practices of operationalising the above-mentioned ethical principles included clearly defining chatbots’ purpose, informing customers/clients about the bot’s non-human status, designing the bot and interactions to redirect customers to a human representative when needed, emphasising respect for individual preferences, and seeking views on bot usage and experiences from customers. The principle of transparency of data collection and usage emerged as the most important to make explicit. This principle was implemented in the chatbot design by including a “an easy-to-find ‘Show me all you know about me’ button, or a profile page for users to manage privacy settings” (Australian Government, n.d.), including an option to opt out, where possible.
Another useful consideration comes from the 2022 concept note developed by Research ICT Africa, which critiques existing Global-North-centred governance frameworks and proposes an approach informed by a positive regulation model rather than a more typical negative regulatory perspective. The authors argue that the governance approach needs to actively redress inequality and injustice and to follow such principles of rights-based AI as “(in)visibility [or representation]; (dis)engagement with technology; and (anti) discrimination” (Research ICT Africa, 2022, p. 3).
Principles such as those discussed above do not imply a one-size-fits-all approach, but rather customisation and tailoring to fit specific HE contexts. Further, having principles as guidance-only would not put the necessary pressure of accountability on universities. A real commitment is needed from university leaders, for example, by embedding these principles in HE policy and procedures. Further, HE-specific AI solutions would need to be guided by a set of industry-relevant standards, inclusive of built-in pedagogical rationale for AI technologies used in teaching and learning scenarios.
Conclusion
Data without context, stripped of in-depth understanding of human experience, is close to meaningless. With cases of AI algorithmic discrimination based on race (Kantayya, 2020), gender (Buolamwini & Gebru, 2018), and religious clothing (Chin, 2020), and with Google notoriously firing AI ethics researchers (Schiffer, 2021; Vincent, 2021), it is urgent that questions and critiques around AI ethics be taken seriously. The focus of any AI endeavour in HE must be on human experience and actual human needs, rather than on predictive technologies, student surveillance or detection of cheating.
While issues around the ethics of AI usage, such as those concerned with privacy and data capture may be similar in other sectors, the specific nature of HE requires context-specific principles to be devised and implemented. Considering how quickly AI systems develop and mature, policies and regulations governing AI must go beyond “catch-up” mode, pre-emptive regulation is required. The development of governance policy and related frameworks should be a cyclical process that considers the fast-evolving nature of AI technologies, allowing for amendments and clarification of “grey areas” as new information emerges. Agile advisory bodies need to provide clarifications and interpretations, hence keeping policy relevant and responsive. Ideally, resultant AI policies would acknowledge existing biases and implement ways to minimise those, recognising the complexity of factors influencing student academic success. A positive regulation model must drive such efforts.
National (and even international) regulation, arrived at via negotiations between industry and sectoral bodies, researchers, and governments, could govern the use of AI systems in HE. While scholars increasingly engage with this topic, important questions around data ownership, privacy, transparency, and ethics are far from resolved. Principles in existence are largely proposed as recommendations, and with rare exceptions, staff and student voices are missing from these processes and recommendations. Although there are several social groups that lobby for the ethical use of technology in wider society, there is an obvious absence of a united HE-focused voice that starts at the universities’ level and is powered by evidence-based research to help advocate for meaningful adoption of ethical principles.
Despite ongoing ‘breakthroughs’ concerned with visual art and writing produced by AI bots that regurgitate content, amid concerns with plagiarism and IP theft, “robots” are not going to take over HE jobs just yet. However, trust and transparency where AI decisions are concerned are still missing. Students and staff are rarely privy to important developments around AI that may directly affect their work and study. The use of AI needs to be rigorously supervised and written into enterprise bargaining agreements, with possible implications for workload and day-to-day functions of professional and academic staff considered. Likewise, AI algorithms used for identifying “students-at-risk” should be critically interrogated and re/designed in a way that does not harm. Clear options must be provided for staff and students to opt out, or at the very least make informed decisions about their involvement and usage. Lastly, the governance of AI, in particular generative AI like ChatGPT, must be solidified in relevant university policies concerned with academic misconduct, plagiarism and so on. Relevant staff require training, tools, and resources, including examples of redesigning assignments to maximise students’ critical thinking, problem solving, and collaboration. One such example was proposed by a peer reviewer of this chapter who suggested the following approach: using a tool like an AI essay generator or text-matching software in class together with students. An auto-generated essay draft could be critiqued, individually or in groups, with students invited to identify issues and gaps and offer improvements. Such an exercise could help demystify these tools and processes as well as help students to critically reflect on the assumptions such tools are making about writing and referencing. Similarly, students could be guided in using tools like ChatGPT in generating responses to essay questions and then critiquing together the limitations. Again, assessment would need to be redesigned in ways that encourage students to use critical thinking and produce unique responses to scenarios. I welcome readers to propose other approaches that make use of AI tools in scenarios that do no harm.
If universities are truly serious about their mission statements centring student experiences, then a data justice framework for AI in HE is non-negotiable. Currently, the use of AI in HE is not always “for good”. Vigilance is essential and it is important to call out risks and problems. At the same time, the extraordinary power of AI can also be harnessed for good. Such opportunities deserve equal attention and resourcing so that AI can serve the ends of social justice in education.
References
Alexander, B., Ashford-Rowe, K., Barajas-Murph, N., Dobbin, G., Knott, J., McCormack, M., Pomerantz, J., Seilhamer, R., & Weber, N. (2019). EDUCAUSE horizon report. EDUCAUSE.
https://library.educause.edu/-/media/files/library/2019/4/2019horizonreport.pdf
Alexander, S., & Rutherford, J. (2019). A critique of techno-optimism: Efficiency without sufficiency is lost. In A. Kalfagianni, D. Fuchs, & A. Hayden (Eds), Routledge handbook of global sustainability governance (pp. 231–41). Routledge.
Australian Government. (n.d.). AI ethics case study: Microsoft. Australia Government Department of Industry, Science and Resources.
Bahadır, E. (2016). Using neural network and logistic regression analysis to predict prospective mathematics teachers’ academic success upon entering graduate education. Kuram ve Uygulamada Egitim Bilimleri, 16(3), 943–64.
https://doi.org/10.12738/estp.2016.3.0214.
Barker, T. (2010). An automated feedback system based on adaptive testing: Extending the model. International Journal of Emerging Technologies in Learning, 5(2), 11–14.
https://doi.org/10.3991/ijet.v5i2.1235.
Barker, T. (2011). An automated individual feedback and marking system: An empirical study. Electronic Journal of E-Learning, 9(1), 1–14.
https://www.learntechlib.org/p/52053/.
Bhattacharya, A. (2021, September 3). How Byju’s became the world’s biggest ed-tech company during the Covid-19 pandemic. Scroll.in.
Bhuiyan, J. (2023, 17 May). OpenAI CEO calls for laws to mitigate ‘risks of increasingly powerful’ AI. The Guardian.
https://www.theguardian.com/technology/2023/may/16/ceo-openai-chatgpt-ai-tech-regulations
Bowen, H. R., & Fincher, C. (1996). Investment in learning: The individual and social value of American higher education. Routledge.
Buolamwini, J., & Gebru, T. (2018). Gender Shades: Intersectional Accuracy Disparities in Commercial Gender Classification Proceedings of the 1st Conference on Fairness, Accountability and Transparency, Proceedings of Machine Learning Research, 81, 77–91.
http://proceedings.mlr.press/v81/buolamwini18a.html?mod=article_inline
Cassidy, C. (2023a, January 10). Australian universities to return to pen and paper exams after students caught using AI to write essays. The Guardian.
Cassidy, C. (2023b, January 17). Lecturer detects bot use in one-fifth of assessments as concerns mount over AI in exams. The Guardian.
Chin, M. (2020, October 22). An ed-tech specialist spoke out about remote testing software – and now he’s being sued. The Verge.
Clark, M. (2021, April 9). Students of color are getting flagged to their teachers because testing software can’t see them. The Verge.
Crompton, H., & Burke, D. (2023). Artificial intelligence in higher education: the state of the field. International Journal of Educational Technology in Higher Education, 20(1), 1–22.
https://doi.org/10.1186/s41239-023-00392-8
Corrin, L., Kennedy, G., French, S., Buckingham Shum, S., Kitto, K., Pardo, A., West, D., Mirriahi, N., & Colvin, C. (2019). The ethics of learning analytics in Australian higher education. www.melbourne-cshe.unimelb.edu.au/__data/assets/pdf_file/0004/3035047/LA_Ethics_Discussion_Paper.pdf
Dawson, D., Schleiger, E., Horton, J., McLaughlin, J., Robinson, C., Quezada, G., Scowcroft, J., & Hajkowicz, S. (2019). Artificial intelligence: Australia’s ethics framework (a discussion paper). Commonwealth Scientific and Industrial Research Organisation, Australia.
https://www.csiro.au/en/research/technology-space/ai/ai-ethics-framework
Delen, D. (2011). Predicting student attrition with data mining methods. Journal of College Student Retention: Research, Theory and Practice, 13(1), 17–35.
https://doi.org/10.2190/CS.13.1.b.
Dencik, L., & Sanchez-Monedero, J. (2022). Data justice. Internet Policy Review, 11(1), 1–16.
https://doi.org/10.14763/2022.1.1615
Dencik, L., Hintz, A., Redden, J., & Treré, E. (2019). Exploring data justice: Conceptions, applications and directions. Information, Communication & Society, 22(7), 873–81.
https://www.tandfonline.com/doi/full/10.1080/1369118X.2019.1606268
Editorial Board. (2023, April). What’s the next word in large language models? Nature Machine Intelligence, 5, 331–32.
https://doi.org/10.1038/s42256-023-00655-z
Facer, K., & Selwyn, N. (2021). Digital technology and the futures of education: Towards non-stupid’ optimism. UNESCO.
https://unesdoc.unesco.org/ark:/48223/pf0000377071
Feathers, T., & Rose, J. (2020). Students are rebelling against eye-tracking exam surveillance tools. Vice.
Ferguson, R. (2019). Ethical challenges for learning analytics. Journal of Learning Analytics, 6(3), 25–30.
http://dx.doi.org/10.18608/jla.2019.63.5
Gilliard, C. (2017). Pedagogy and the logic of platforms. Educause Review, 52(4), 64–65.
https://er.educause.edu/-/media/files/articles/2017/7/erm174111.pdf
Global Market Insights (2022). Artificial intelligence (AI) in education market.
https://www.gminsights.com/industry-analysis/artificial-intelligence-ai-in-education-market
Gullo, K. (2022, March 25). EFF client Erik Johnson and Proctorio settle lawsuit over bogus DMCA claims. Electronic Frontier Foundation.
Guzmán-Valenzuela, C., Gómez-González, C., Rojas-Murphy Tagle, A., & Lorca-Vyhmeister, A. (2021). Learning analytics in higher education: A preponderance of analytics but very little learning? International Journal of Educational Technology in Higher Education, 18(1), 1–19.
https://doi.org/10.1186/s41239-021-00258-x
Hall, A. (2007, August). Vygotsky goes online: Learning design from a socio-cultural perspective [Workshop paper]. Learning and socio-cultural Theory: Exploring modern Vygotskian perspectives international workshop 2007, Australia.
https://ro.uow.edu.au/llrg/vol1/iss1/6
Hasal, M., Nowaková, J., Ahmed Saghair, K., Abdulla, H., Snášel, V., & Ogiela, L. (2021). Chatbots: Security, privacy, data protection, and social aspects. Concurrency and Computation: Practice and Experience, 33(19), 1–13.
https://doi.org/10.1002/cpe.6426
Herodotou, C., Hlosta, M., Boroowa, A., Rienties, B., Zdrahal, Z., & Mangafa, C. (2019). Empowering online teachers through predictive learning analytics. British Journal of Educational Technology, 50(6), 3064–79.
https://doi.org/10.1111/bjet.12853
Hoffmann, A. L. (2019). Where fairness fails: Data, algorithms, and the limits of antidiscrimination discourse. Information, Communication & Society, 22(7), 900–15.
https://doi.org/10.1080/1369118X.2019.1573912
Jones, K. M., Asher, A., Goben, A., Perry, M. R., Salo, D., Briney, K. A., & Robertshaw, M. B. (2020). “We’re being tracked at all times”: Student perspectives of their privacy in relation to learning analytics in higher education. Journal of the Association for Information Science and Technology, 71(9), 1044–59.
https://doi.org/10.1002/asi.24358
Kantayya, S. (Director). (2020). Coded bias. [Documentary]. 7th Empire Media.
Klee, M. (2023, 17 May) Professor flunks all his students after ChatGPT falsely claims it wrote their papers. Rolling Stone.
Lee, N. T. (2018). Detecting racial bias in algorithms and machine learning. Journal of Information, Communication and Ethics in Society, 16(3), 252–60.
https://doi.org/10.1108/JICES-06-2018-0056
Li, X. (2007). Intelligent agent-supported online education. Decision Sciences Journal of Innovative Education, 5(2), 311–31.
https://doi.org/10.1111/j.1540-4609.2007.00143.x.
Luckin, R., Holmes, W., Griffiths, M., & Forcier, L. B. (2016). Intelligence unleashed: An argument for AI in education.
http://discovery.ucl.ac.uk/1475756/
Marginson, S. (2011). Higher education and public good. Higher Education Quarterly, 65(4), 411–33.
https://doi.org/10.1111/j.1468-2273.2011.00496.x
Mascarenhas, N. (2022, March 10). Course Hero scoops up Scribbr for subject-specific study help. Join TechCrunch+.
https://techcrunch.com/2022/03/10/course-hero-scribbr/
Matthews, C., Twaddle, J., Cashion, G., & Wu, S. (n.d.). Redefining the role of EdTech: A threat to our education institutions, or a strategic catalyst for growth? PwC.
Mirbabaie, M., Brünker, F., Möllmann Frick, N. R., & Stieglitz, S. (2022). The rise of artificial intelligence: Understanding the AI identity threat at the workplace. Electronic Markets, 32(1), 73–99.
https://doi.org/10.1007/s12525-021-00496-x
Morris, S. M., & Stommel, J. (2017, June 15). A guide for resisting edtech: The case against Turnitin. Hybrid Pedagogy.
https://hybridpedagogy.org/resisting-edtech/
Mphahlele, A., & McKenna, S. (2019). The use of Turnitin in the higher education sector: Decoding the myth. Assessment & Evaluation in Higher Education, 44(7), 1079–89.
https://doi.org/10.1080/02602938.2019.1573971
Newton, P. M. (2018). How common is commercial contract cheating in higher education and is it increasing? A systematic review. Frontiers in Education, 3(67), 1–18. https://doi.org/10.3389/feduc.2018.00067
Nietzel, M. T. (2020, March 12). How colleges are using chatbots to improve student retention. Forbes.
Ouyang, F., & Jiao, P. (2021). Artificial intelligence in education: The three paradigms. Computers and Education: Artificial Intelligence, 2, 1–6.
https://doi.org/10.1016/j.caeai.2021.100020
Pechenkina, E. (2014). Being successful. Becoming successful: An ethnography of Indigenous students at an Australian university [Doctoral dissertation, University of Melbourne, Faculty of Arts].
https://minerva-access.unimelb.edu.au/items/1856f614-cf78-5700-a1 a4-64c8f6360455/full
Pechenkina, E. (2015). Who needs support? Perceptions of institutional support by Indigenous Australian students at an Australian University. UNESCO Observatory Multi-Disciplinary Journal in the Arts, 4(1), 1–17.
https://www.unescoejournal.com/volume-4-issue-1/
Pelletier, K., Brown, M., Brooks, D. C., McCormack, M., Reeves, J., Arbino, N., Bozkurt, A., Crawford, S., Czerniewicz, L., Gibson, R., Linder, K., Mason, J., & Mondelli, V. (2021). EDUCAUSE horizon report. EDUCAUSE.
Pelletier, K., McCormack, M., Reeves, J., Robert, J., Arbino, N., Dickson-Deane, C., Guevara, C., Koster, L., Sánchez-Mendiola, M., Bessette, L. S., & Stine, J. (2022). EDUCAUSE Horizon Report. EDUCAUSE.
Pérez, J. Q., Daradoumis, T., & Puig, J. M. M. (2020). Rediscovering the use of chatbots in education: A systematic literature review. Computer Applications in Engineering Education, 28(6), 1549–65.
https://doi.org/10.1002/cae.22326
Pham, T. D., Vo, D., Li, F., Baker, K., Han, B., Lindsay, L., Pashna, M., & Rowley, R. (2020). Natural language processing for analysis of student online sentiment in a postgraduate program. Pacific Journal of Technology Enhanced Learning, 2(2), 15–30.
https://doi.org/10.24135/pjtel.v2i2.4
Prinsloo, P. (2017). Fleeing from Frankenstein’s monster and meeting Kafka on the way: Algorithmic decision-making in higher education. E-Learning and Digital Media, 14(3), 138–63.
https://doi.org/10.1177/2042753017731355.
Prinsloo, P., Slade, S. (2017). Big data, higher education and learning analytics: Beyond justice, towards an ethics of care. In B. K. Daniel (Ed.), Big data and learning analytics in higher education (pp. 109–24). Springer, Cham.
Research ICT Africa (2022). Concept Note, From Data Protection to Data Justice: Redressing the uneven distribution of opportunities and harms in AI.
Riedel, A., Essa, A., & Bowen, K. (2017, April 12). 7 Things you should know about artificial intelligence in teaching and learning. EDUCAUSE.
Sabzalieva, E., & Valentini, A. (2023). ChatGPT and artificial intelligence in higher education: quick start guide. United Nations Educational, Scientific and Cultural Organization Digital Library.
https://unesdoc.unesco.org/ark:/48223/pf0000385146
Sandu, N., & Gide, E. (2019). Adoption of AI-Chatbots to enhance student learning experience in higher education in India. Proceedings of the 18th international conference on information technology based higher education and training (pp. 1–5). IEEE.
https://doi.org/10.1109/ITHET46829.2019
Schiff, D. (2021). Out of the laboratory and into the classroom: The future of artificial intelligence in education. AI & Society, 36(1), 331–48.
https://doi.org/10.1007/s00146-020-01033-8
Schiffer, Z. (2021, February 20). Google fires second AI ethics researcher following an internal investigation. The Verge.
https://www.theverge.com/2021/2/19/22292011/google-second-ethical -ai-researcher-fired
Selwyn, N., & Gašević, D. (2020). The datafication of higher education: Discussing the promises and problems. Teaching in Higher Education, 25(4), 527–40.
https://doi.org/10.1080/13562517.2019.1689388
singh, s. s., Davis, J. E., & Gilliard, C. (2021). Smart educational technology: A conversation between sava saheli singh, Jade E. Davis, and Chris Gilliard. Surveillance & Society, 19(2), 262–71.
https://doi.org/10.24908/ss.v19i2.14812
Stoesz, B. M., Eaton, S. E., Miron, J., & Thacker, E. J. (2019). Academic integrity and contract cheating policy analysis of colleges in Ontario, Canada. International Journal for Educational Integrity, 15(1), 1–18.
https://doi.org/10.1007/s40979-019-0042-4
Swauger, S. (2020, April 2). Our bodies encoded: Algorithmic test proctoring in higher education. Hybrid Pedagogy.
https://hybridpedagogy.org/our-bodies-encoded-algorithmic-test-proctoring-in-higher-education/
Swinburne University of Technology. (2012). Student academic misconduct regulations 2012.
https://www.swinburne.edu.au/about/policies-regulations/student-academic-misconduct/#academic_misconduct_regulations_4
Tanveer, M., Hassan, S., & Bhaumik, A. (2020). Academic policy regarding sustainability and artificial intelligence (AI). Sustainability, 12(22), 1–13.
https://doi.org/10.3390/su12229435
Taylor, L. (2017). What is data justice? The case for connecting digital rights and freedoms globally. Big Data & Society, 4(2), 1–14.
https://journals.sagepub.com/doi/full/10.1177/2053951717736335
Vincent, J. (2021, April 13). Google is poisoning its reputation with AI researchers. The Verge.
Watters, A. (2021). Teaching machines: The history of personalized learning. MIT Press.
Welham, D. (2008). AI in training (1980–2000): Foundation for the future or misplaced optimism? British Journal of Educational Technology, 39(2), 287–303.
https://doi.org/10.1111/j.1467-8535.2008.00818.x.
Zawacki-Richter, O., Marín, V. I., Bond, M., & Gouverneur, F. (2019). Systematic review of research on artificial intelligence applications in higher education – where are the educators? International Journal of Educational Technology in Higher Education, 16(1), 1–27.
https://doi.org/10.1186/s41239-019-0171-0
Zhang, D., Mishra, S., Brynjolfsson, E., Etchemendy, J., Ganguli, D., Grosz, B., Lyons, T., Manyika, J., Niebles, J. C., Sellitto, M., Shoham, Y., Clark, J., & Perrault, R. (2021). The AI index 2021 annual report. Stanford University.
https://aiindex.stanford.edu/ai-index-report-2021/arXivpreprint arXiv:2103.0631
1 See the Amiel & Deniz chapter “Advancing ‘openness’ as a strategy against platformization in education” for a detailed discussion of these issues.
2 See, for example, this article in review: Bowell P., Smith G., Pechenkina E., & Scifleet, P. ‘You’re walking on eggshells’: Exploring subjective experiences of workplace tracking. Culture and Organization, 29(6), 1-20.
3 Notably, the leading text-matching company was recently sold in some of the biggest edtech acquisitions in the history of the industry (see https://www.edsurge.com/news/2019-03-06-turnitin-to-be-acquired-by-advance-publications-for-1-75b)
4 See, for instance, Swinburne University of Technology’s student academic misconduct regulations 2012: https://www.swinburne.edu.au/about/policies-regulations/student-academic-misconduct/#academic_misconduct_regulations_4
5 The Institute is no longer operating; www.buckingham.ac.uk/research-the-institute-for-ethical-ai-in-education/
6 According to its website, AI campus is “the AI Campus is a not-for-profit space where research, start-ups and corporates come together and collaborate on Artificial Intelligence.”; www.aicampus.berlin/
7 According to its website, AAIA is “an academic, non-profit and non-governmental organization voluntarily formed 1074 academicians worldwide”; https://www.aaia-ai.org/
8 This image was inspired by Emeritus Associate Professor Cheryl Hodgkinson-Williams’s peer review.
9 Principles for Ethical Use of Personalized Student Data are available here: https://www.athabascau.ca/university-secretariat/_documents/policy/principles-for-ethical-use-personalized-student-data.pdf
10 G20/OECD Principles of Corporate Governance; www.oecd.org/corporate/principles-corporate-governance/
11 See Australia’s Artificial Intelligence Ethics Framework for further information: www.industry.gov.au/data-and-publications/australias-artificial-intelligence-ethics-framework/testing-the-ai-ethics-principles/ai-ethics-case-study-microsoft