3. Modelling as Semiotic Process82
© 2023 Arianna Ciula et al., CC BY-NC 4.0 https://doi.org/10.11647/OBP.0369.03
3.1 Introducing a Semiotic Framework
Digital Humanities (DH) is a field of research engaged in exploring how humanities scholarship is transformed and extended by the digital and vice versa. This mutual transformation and extension concerns tools (technology) as well as epistemologies (how we come to know). One of the core practices of DH research is modelling (McCarty 2005, pp. 20-72; Buzzetti 2002; Flanders and Jannidis 2015; 2018), which implies the translation of complex systems of knowledge into models to be manipulated (processed) computationally.83
In this chapter we contextualise DH practices within a semiotic conceptualisation of modelling adapted from Kralemann and Lattmann (2013) and complemented by intermedia theories on iconicity (Elleström 2013). Despite being neglected or relatively unexplored in DH, a semiotic framework allows us to see modelling primarily as a strategy to make sense (signification) via practical thinking (creating and manipulating models). From a semiotic perspective, modelling is a process of signification enacting a triadic cooperation between object, representamen (form of a sign) and interpreter (significate outcome of a sign or the thing that is signified). A semiotic framework is therefore instrumental in stressing that modelling and models are dynamic by nature and to understand modelling as an open process in renewed terms. Referring to Peirce’s classification of hypoicons,84 in this chapter we will reflect on some DH examples of modelling in the form of images, diagrams and metaphors. We claim that a semiotic understanding of modelling could ultimately allow us to further investigate the duality of object vs. model (as well as sign vs. context). This is central to the overall argument of the book, as outlined in the previous chapter where we proposed to consider modelling as a creative and pragmatic process of thinking and reasoning in which metaphors assume a central role and meaning is negotiated through the creation and manipulation of external representations combined with an imaginative and faceted use of formal and informal languages.
This chapter has two parts: in the first part (Section 3.2), some core concepts around the ontology and epistemology of modelling are introduced to explain the argument which follows; in the second part (Section 3.3), DH and semiotics are put in dialogue with one another to discuss what a semiotic model of modelling entails in relation to two other central concepts, namely iconicity and reasoning.
3.2 Modelling as a Process of Signification
As discussed in Chapter 1 and in Ciula et al. (2018), the complexity of the concept of modelling is not only the result of decades of theory and centuries of practices in modelling, but is deeply embedded in the roots and history of the term itself. Therefore, it is not surprising that there are multiple, often discipline-dependent definitions of modelling.
In the last two decades there has been a significant development of theory (with due connections to interdisciplinary research e.g. in McCarty 2005; 2009) that complements the practice-based tradition of DH as a field. The practice of modelling in DH is mainly theorised around understandings of modelling adopted in the techno-sciences and computer science in particular (Flanders and Jannidis 2018; Mahr 2009). This theory builds especially on an analytical understanding of modelling inherited from computer science (e.g., the practice of data modelling instrumental to system development), but also more widely on the consideration that modelling is an heuristic strategy of coming to know, which spans multiple scientific cultures and epistemic traditions. Recently, model-making was theorised within a semiotic framework, whereby modelling is presented as a process of signification (semiotic process of meaning-making; see Kralemann and Lattmann 2013; Ciula and Marras 2016; Ciula and Eide 2014; 2017).
Taking stock of these approaches and their intersections by modelling, we mean mainly two things:
- A creative process of thinking and reasoning, where meaning is made and negotiated through the creation and manipulation of external representations;
- A research strategy intended as a process by which researchers make and manipulate external representations (“imaginary concreta”, Godfrey-Smith 2009) to make sense of objects and phenomena (Ciula and Eide 2017, p. i33).
In line with Nersessian’s (2008) continuum hypothesis adopted in her cognitive-historical account of modelling, (2) (modelling in research and science) is a specification of (1) (modelling in life), in the sense that it is constrained and enhanced by the idiosyncratic contexts and purposes of research endeavours in science and scholarship at large. Figures 3.1 and 3.2 are examples of historical models developed to study molecular structures.
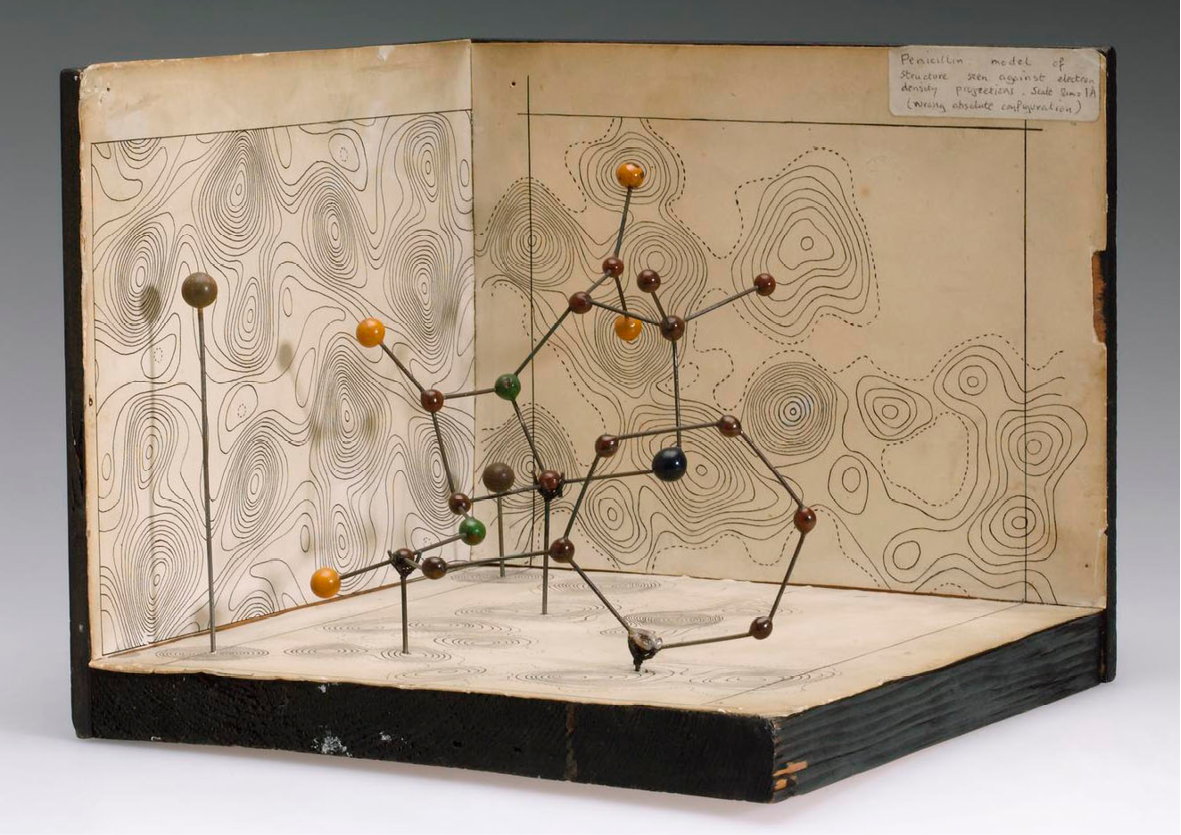
Fig. 3.1 Molecular model of Penicillin by Dorothy Hodgkin, c.1945, who used large punch-card operated tabulators to help analyse the patterns cast by reflected X-rays. Science Museum Group Collection © The Board of Trustees of the Science Museum.
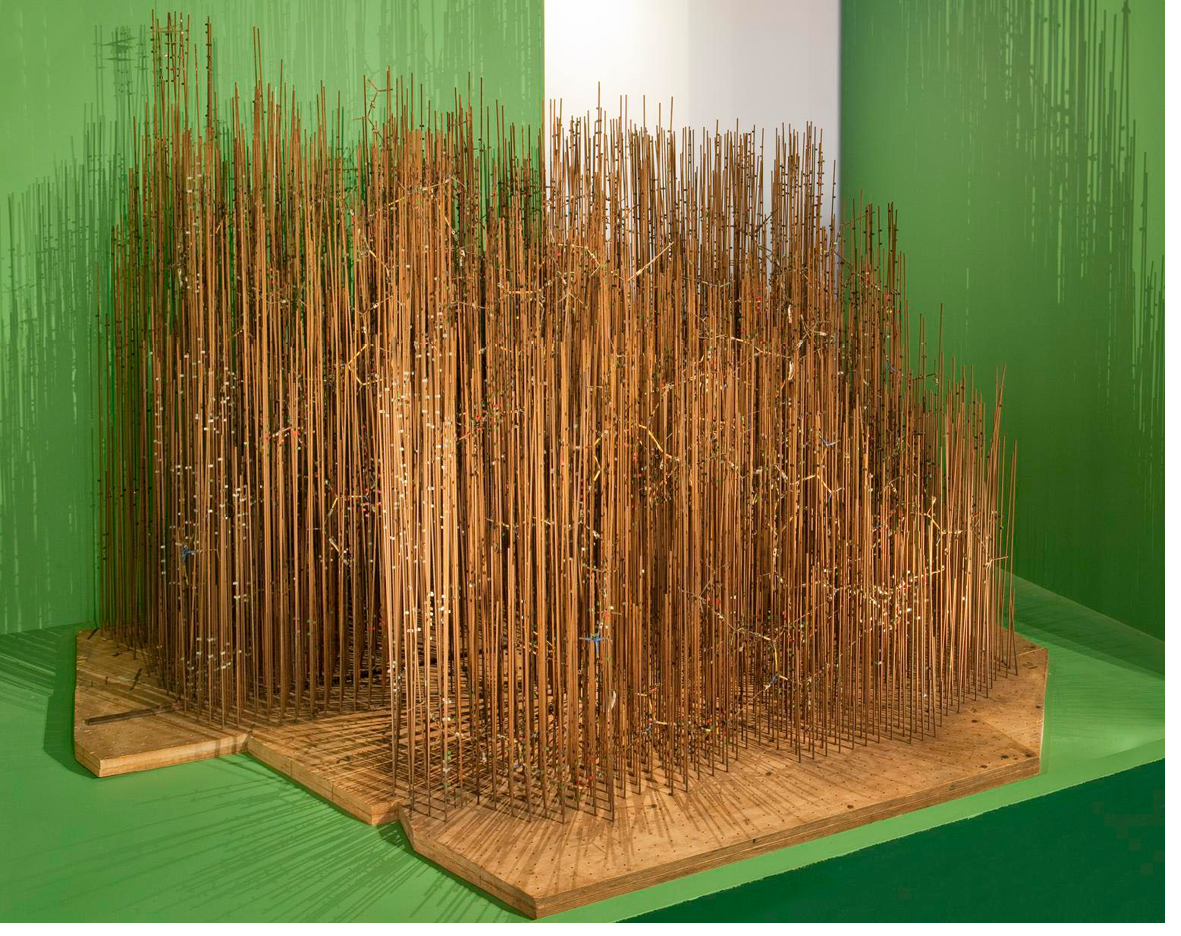
Fig. 3.2 Model of myoglobin (“forest of rods”) constructed in 1960 with mecano clips to represent the molecular structure of a compound that stores oxygen in muscles. Science Museum Group Collection © The Board of Trustees of the Science Museum.
At a rather abstract level and in line with sense (1) mentioned above, a model provides a shareable language through which to talk about and understand (hence communicate, as we will see in Chapter 4) existing or possible realities. Historical analysis of scientific practices backs up this understanding and accounts for model-based reasoning (in sense (2) above) as a social problem-solving strategy grounded in everyday signification (sense (1) above). At a more concrete level, cognitive sciences and the philosophy of scientific modelling contribute to the understanding that cognition is distributed and shared through external mediations—of which formal and informal models are but instantiations (see Chapter 4)—in modelling acts of everyday life (1) as well as in scientific contexts (2).
Whether generic as in sense (1) above or more specific as in sense (2), the process of signification that unfolds in modelling activities implies translation and negotiation of meaning. Within a scientific or scholarly context, these translations and negotiations occur both in modelling processes engaged with abstraction of complex phenomena into rule-based procedures — what Gooding (2003, p. 280) calls “reduction” — and in modelling directed at the re-integration of the results of that abstraction or reduction into interpretative frameworks such as explanatory diagrams and data visualisations — what Gooding (2003, p. 278) calls “expansion”.
As a process of translation (see Chapter 2 on this concept) and negotiation of meaning, whether in its reductive or expansive role, modelling has therefore both terminological and semiotic implications. In addition, modelling is strictly related to foundational ontological and epistemological issues concerning the nature of the objects being modelled (when we encounter the model of an existing object, for example) or ‘created’ (when we encounter a model for an object to be)85 and the relations between modeller, modelling and model.
If we assume that in a humanities research setting, the “Modelling process is part of what is being modelled” (Flanders 2012), it becomes crucial to ask what model of modelling can be ‘adequate’ to DH. So, for example, if a text (which as an external object can only occur in the form of a document or an acoustic stream) becomes the object ‘text’ only when we entertain the idea or a representation of a model of it, how that model comes to be is of paramount importance to guide any further analysis or computational processing. It is precisely to address the question of ‘what model of modelling can be ‘adequate’ to DH’ that we reflect below on three constitutive elements of humanities research: objects of study (Section 3.2.1) and practices of modelling (Section 3.2.2), followed by a semiotic perspective on modelling acts operating as languages of signification, reasoning, and DH scholarship (Section 3.3).
3.2.1 Factuality and Fictionality of Objects
Rooted in the history of science and philosophy, the discussion concerning the nature of an object of experience has been led by crucial epistemological and philosophical questions such as: How can objects pertaining to the physical world (causally determined) have an effect on the human mind (generally considered as a free entity)? Under which conditions can the existence of external objects be processed?
Within the history of science, the problem is framed as a struggle to reach an accurate representation of nature and to find an adequate language able to provide the link between external objects, belief, and knowledge (Daston 2000). Encompassing discussions spanning from the language of God to the ‘language of nature’ (Galileo Galilei), natural philosophers have traditionally addressed the problem by questioning the truth and falsity (in senso lato) of the objects of scientific knowledge and of the mathematical entities explaining them. Especially in the early modern era in the Western world, the making and the use of microscopes, telescopes and lenses changed the perspective, the distances, and the accessibility to the smallest entities as well as to those objects farthest away. A process of approximation based on analogy, directed at identifying relational abstractions, at mapping the structure of the object, at defining, analysing and measuring, rather than at ‘directly’ observing objects, was introduced. Consequently, the perception of the nature of the object changed radically from what could be directly measured and touched to something that is somehow ‘created’ via mediated observations. In early modern times, the object of experience assumed a complex profile and was often conceived as the result of a cultural process and activity86 (Daston 2000).
A still common and affirmed image of science today is that of an activity based on the experimental gathering of facts, a mathematical modelling of results deduced from facts, and the framing of hypotheses and theories.87 This process of development and construction of scientific objects is dependent on the intrinsic interweaving of perception, representation and consciousness in a multilayered socio-technical environment; it has its counterpart also in scholarly debates in the humanities, ranging from questions around the nature of consciousness (Palmieri 2012) and the nature of cognition, to the definition of the ethnographic object, just to mention a few.
Semiotics has contributed to this discussion with its theory of signs whereby a semiotic object is generally intended as what a sign represents (or encodes) within a generative interpretative process. Historical, anthropological, sociological and ethnographic disciplines within and outside the humanities consider cultural artefacts - spanning, for example, from weapons to inscriptions, from folk dances to theatre productions—as their objects of analysis. In the last century, material culture approaches have evolved across those disciplines to inform object-based analyses aiming at unpacking artefacts, writing their biographies, their stories, shaped by use (“thick descriptions”, Geertz 1973). Within this perspective, artefacts are considered intentional, cultural releasers “animated by their passage through the lives of people” (Graves-Brown 2000). While the total meaning of an artefact might be unattainable, the claim is that there are clues to the ways of thinking and living of those who made it. Similarly, in the realm of textual scholarship, theories connected to material culture approaches, such as the social theory of texts, recognise texts as open objects to be understood in the dynamic condition of their creation, formulation, and media production (see Chapter 4) on the one hand and their use, reception, and interpretation/understanding on the other.88
DH research89 has tended to prefer texts as objects of recurrent modelling activities. In line with a social theory approach, by texts we mean dynamic cultural objects (material documents as well as conceptual objects) contingent on the contexts of their production and reading or fruition, expressed in a wide range of manifestations from linear to discontinuous narrative, from manuscripts to printed editions, from analogue codes to digital re-coding, encompassing hybrid modalities.
Taking into consideration the evolution of the understanding of the nature of objects of experience outlined above, the objects that take part in an act of modelling—whether they are the texts (or other objects) being studied or the models being created—feature both an element of factuality (an experienced substance) and one of fictionality (they are construals that presuppose some rules of artifice). This implies that in a DH modelling activity, making explicit both components and their interaction is paramount; this means making explicit the perspectives of study towards the analysis of an object both in interpretative and technical terms. Making explicit the facts of modelling—the contexts in which models are created, how they are used and what forms they take—as well as its fictionality—the constraints of the discipline or knowledge domain that determine the creation and use of those models and the rules of the technical systems according to which they are created and used—go hand in hand. For example, unpacking various theories of text (as exemplified using visual and descriptive languages in Chapter 5) is a way of making explicit the fictionality of a modelling act as important as algorithm criticism. Similarly, describing the material realisation of a model (its size, production process, language of expressions, materials, modalities, context of use) is useful for the observation of its factuality and practical ‘affordances’ (in the sense used by Norman (1988) as fundamental properties that determine how a model could be used).
3.2.2 Modelling Practices
If acts of modelling are used to make sense of our cultural objects, they are meaning-making practices and hence in themselves objects of study for the humanities.90 Therefore the modelling process can also be posited as something to be modelled, as outlined above.
Modelling is considered to be one of the core research methodologies in DH, particularly in its earlier incarnation as humanities computing (McCarty 2005).91 In DH-specific research and teaching, modelling has mainly been embedded within a techno-scientific approach with connections to multifaceted conceptualisations of modelling as used and understood by other disciplines and practices.
In very sketchy and simplified terms: as modellers, we behold a certain understanding of a cultural phenomenon of some sort. By way of (often informal) external models of aspects of such understanding (e.g., sketchy graphical representations such as ad hoc diagrams not compliant with formal languages or conventions), formal92 and computable models of components of that understanding are generated and manipulated in an iterative cycle, which progressively—often via repeated and adjusted trials and errors—make us gain a more nuanced analysis of reduced portions of the phenomenon from which we originally departed. Sometimes the analysis we develop is in conflict with our original takes on that phenomenon. These iterative experimental cycles have been extensively theorised within design and development practices engaged with making things and building methods.93 In connection with digital modelling in literary criticism, Moretti (2013) talks about the well-known process of ‘operationalisation’, whereby concepts of humanistic inquiry (e.g., Hegelian pathos in Greek tragedies) are literally operationalised, made measurable, hence creating empirical objects of study which bear theoretical consequences for the discipline of literary study as a whole. Bode (2020, p. 100) enriches the discussion further by stating that in quantitative literary studies “modeling is the means by which literary concepts and artefacts are both made computable and computed”.
The epistemic value of modelling in research is ascribed in particular to the operationalisation aspects of modelling. The reconceptualisation of a theory used in a specific field can occur via the process of making humanistic objects or phenomena of computable enquiry; in parallel, during modelling interactions, the computational methods themselves are subject to critique (e.g., in the form of algorithmic criticisms), to phases of refining and re-building, as part of one or more modelling cycles. A pragmatic approach to modelling practices accounts for different levels of analysis and for the recognition of gaps and bias (Bode 2020) embedded in the operationalisation (inevitably a reduction) of a theory or a conceptual device, but also in the construction of datasets (inevitably partial) and of the models used to compute them.
A domain often used to explain modelling practices in DH is digital textual editing. In this context, one might adopt the Ordered Hierarchy of Content Objects (OHCO) model combined with a Text Encoding Initiative (TEI) document abstract model and schema (if unfamiliar with this, see “The OCHO Model” in Chapter 5). During the modelling process itself, the OHCO model as well as the TEI categorisations might be questioned deeply, for example, because of their inadequateness to represent the modeller’s theory of the historical documents at hand or because of the constraints the models force on the specific objects of interest:
[The modeller] will most likely go back and forth in her modelling efforts to match – based on her knowledge and scholarly language – what she would like to elicit in the document (e.g. the organisation of the diplomatic formulas, the occurrence of names of witnesses in certain locations in the document, the abbreviations occurring in the date clauses) with the formal hierarchical structure proposed by one or more of the TEI guidelines chapters on the encoding of primary sources. Once this mapping reaches a certain stability and she is able to actually process that model, her interpretative activity will likely have to zoom in and out of these manifestations (e.g. between single cases and emerging patterns). She will often puzzle over exceptions that will allow her to iteratively refine the original mapping or require a total rethinking of categories and of the OHCO model being adopted. (Ciula and Marras 2016)
We shall add that even in empirical, technical, or highly implementation-focused settings, formal (e.g. computationally rule-bound) and informal (e.g. narrative, story-based) models coexist and interact to give sense to our modelling efforts.94 For example, while parts of the documentation to accompany code for a computational implementation has the main purpose of making the source code understandable in a narrow sense, best practices in documentation aim also at making the system understandable in the context of specific use cases, in the relevant research setting and activities. This sort of documentation is expressed mainly in informal language. Ciula et al. (2023) illustrated how modelling can occur at every phase of the Software Development Lifecycle in a Research Software Engineering DH laboratory and across its operational methods of design (or designing), building, maintaining and monitoring (Smithies and Ciula 2020). They showcase how in that setting, one of the core functions of modelling practices is “to support the translations of cycles of analysis and design”. Building on the idea in Génova et al. (2009) of modelling activities as trajectories in the multidimensional space along the axes of purpose, reality and abstraction, the examples of modelling practices they bring to the fore are “all but linear”, evolve organically and intersubjectively with the agency of multiple actors (team members and project partners) and the mediation of a diversity of languages of expression. The resulting models are “artefacts of different kinds including but not limited to computational models” expressed via “verbal descriptions, graphical representations following more or less standardised conventions, code”:
Each modelling cycle produces one or more models which can contribute to bridging building phases and increments (analysts ← models → developers ← models → designers). This process is far from linear and unidirectional. Its epistemological value can be limited to one role (for example an analyst sketches a model of a domain of knowledge for her own understanding and to inform requirement elicitation at a later stage), to more than one role within the engineering team or indeed to the overall research team including partners outside KDL. It facilitates communication, shared understanding and ultimately the building of a final product charged with meaning sedimented in more or less ephemeral intermediary products (widely defined here as models). More often than not, models are also shared outside the research team of a project with other users and researchers (e.g. in focus groups and workshops as part of user research and testing or dissemination activities). (Ciula et al. 2023, p. 271)
Modelling is a pragmatic activity framed within the complex cognitive, social, and cultural functioning of DH practices affected by cross-linguistic and interdisciplinary dimensions. As introduced in Chapters 1, 2 and further discussed in Section. 3.3 and elsewhere (Ciula and Marras 2016), a pragmatic stance highlights how the relational and dynamic aspects of modelling operate. Pragmatic modelling is anchored to theory and language, while at the same time claiming indeterminacy and some level of independence from both.
3.3 Semiotics of Modelling in DH
In this section we would like to draw attention to some key intersections between DH and semiotics and to how, in some cases, semiotic conceptual tools applied and used in a DH context acquire a specific meaning. In addition to modelling itself, at least two key cross concepts emerge as particularly relevant, namely iconicity and reasoning.
Recently, Kralemann and Lattmann (2013) proposed a semiotic model of modelling which they claim pertains to modelling in the sciences as well as in life more generally (semiosis). We maintain that DH practices of modelling can also be contextualised within this semiotic conceptualisation of modelling, albeit complemented by additional semiotic and intermedia theories on iconicity (see Chapter 4) as well as a pragmatic understanding of the importance of a creative use of language in modelling. As discussed in Chapter 1, a pragmatic understanding of modelling, as practised in DH research, can facilitate the recognition that modelling operates within relational and dynamic cycles which are elicited via negotiations over the use of modelling languages (e.g., by renaming categories of analysis or adopting neologisms).
Considering modelling as a process of signification and reasoning in action does not mean that we leave aside its role in human-machine communication, or its implementation-oriented purpose of creating working digital artefacts in software-intensive DH research.95 Contextualising modelling within a semiotic framework is an attempt to provide a wider framework of analysis to account for the multiple facets that modelling takes in a DH context, from the translation of concepts into formulas (see for example “Argamon: Burrows’ Delta Formula” in Chapter 5), to the use of metaphors in constructing a project language; from the integration of design workflows into evolutionary development of a technical solution, to the construction of a narrative used to make sense of a clustering graph. A view of modelling as a meaning-making process sees very different yet integrated workflows included in the remit of modelling acts and, consequently, diverse populations of models ranging from conceptual schemes describing and depicting a theory (as amply exemplified in Chapter 5) to artefacts resulting from data modelling and interface design activities.
The challenge is therefore to see whether modelling intended as a sense-making strategy (signification) via practical thinking (creating and manipulating models) is an adequate lens through which to study modelling as research and teaching strategies in DH and to complement other perspectives, for example in the philosophy of computing or software engineering.96
3.3.1 Models as Signs and Grades of Iconicity
Modelling as a general strategy of creative reasoning and as a scientific methodology has been fruitfully related to the semiotic concept of iconicity as a form of extended similarity (Kralemann and Lattmann 2013; Ljungberg 2018; Lattmann 2018). The semiotic theory proposed by Peirce identifies three types of signs based on the relationship between the object and the sign:97 symbols (e.g. conventional names in lexicons of languages used to denote objects via arbitrary association), icons (e.g. onomatopoeic words such as ‘splash’), and indexes (signs used to point directly to their meaning, such as ‘there’). The general semiotic meaning of iconicity is resemblance or analogy between the form of a sign (representamen, source) and its object (target):
Representation based on resemblance generally falls under the heading of ‘iconicity’. When something is understood to be a sign of something else because of shared, similar qualities, it is referred to as an iconic sign. (Elleström 2013, p. 95)
The discussion on iconicity and modes of reasoning is one of the leading themes in semiotics since its beginnings in Peirce’s theory and is still the subject of ongoing interdisciplinary inquiry (Giardino and Greenberg 2015): “it is by icons only that we really reason” (Peirce 1933, CP 4.127 [1893]). Kralemann and Lattmann (2013, pp. 3399–3400) claim that models are icons, because the dominant relationship with the objects they represent is one of similarity, as shown in Fig. 3.3. In Peircean theory, such an iconic relationship of similarity is what makes icons signify. Icons act as signs based on how the relation of similarity is enacted: via simple qualities of their own in the case of images; via analogous relations between parts and whole and between parts in the case of diagrams; via parallelism of qualities with something else in the case of metaphors (Olteanu 2015, pp. 77 and 193).
Different grades of iconic similarity between sign and object as theorised by Peirce correspond to three kinds of models in Kralemann and Lattmann:98
- image-like models, for example, real-life sketches where single qualities such as forms and shapes enable them to act as signs of the original objects they represent in given circumstances;
- relational or structural models, for example, diagrams such as the relation exhibited in the graph of a mathematical equation, where the “interdependence between the structure of the sign and the structure of the object” (Kralemann and Lattmann 2013, p. 3408) enables the modeller to make inferences about the original by manipulating its model;
- metaphor-like models which represent attributes of the original by a non-standard kind of parallelism with something else which generates further models (in this generative sense metaphors are considered meta-models; (Kralemann and Lattmann 2013, p. 3409).
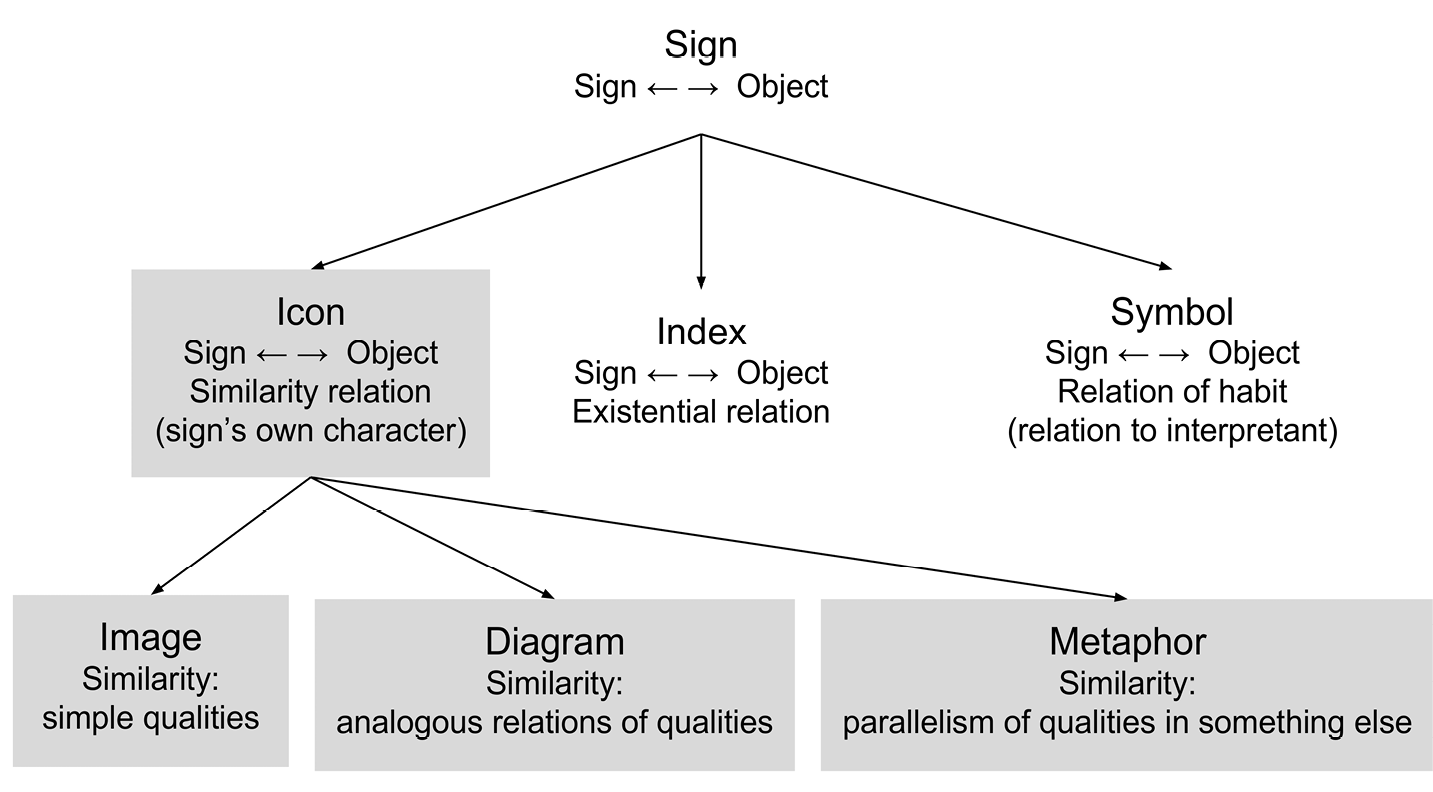
Fig. 3.3 This is a reproduction of Ciula and Eide (2017, Fig. 2), based on Kralemann and Lattmann (2013, Fig. 2). Highlighted in grey is the Peircean sign type of icon associated with models by Kralemann and Lattmann. Based on how the respective similarity relations signify, pure icons or hypoicons are classified further by Peirce into images, diagrams and metaphors.99
In Figure 3.4 below the object is the apple and the models (icons) are the three different apple icons exemplifying the grades of iconicity:
The representamen of an image is perceptually close to its object, which means that the object may be sensuously perceived in much the same way as the representamen (this is a conception that is close to Peirce’s own few remarks on the image). The representamen of a metaphor is at a greater distance from its object, which means that the interpretation of a metaphor includes one or several cognitive leaps that make the similarity between representamen and object apparent (Elleström 2013, p. 104).
A semiotic understanding of modelling shows clearly how the analytical dichotomy of objects vs. models is useful, but also misleading. Indeed, the semantics of an object changes when the model changes; the meaning of the apple in the metaphorical example is different from the apple in the diagrammatic example. The context of the interpretation changes the sign, but the sign also changes the context of interpretation. In modelling, ontology (of objects and models) and epistemology (how we know objects via models and modelling) are entangled.
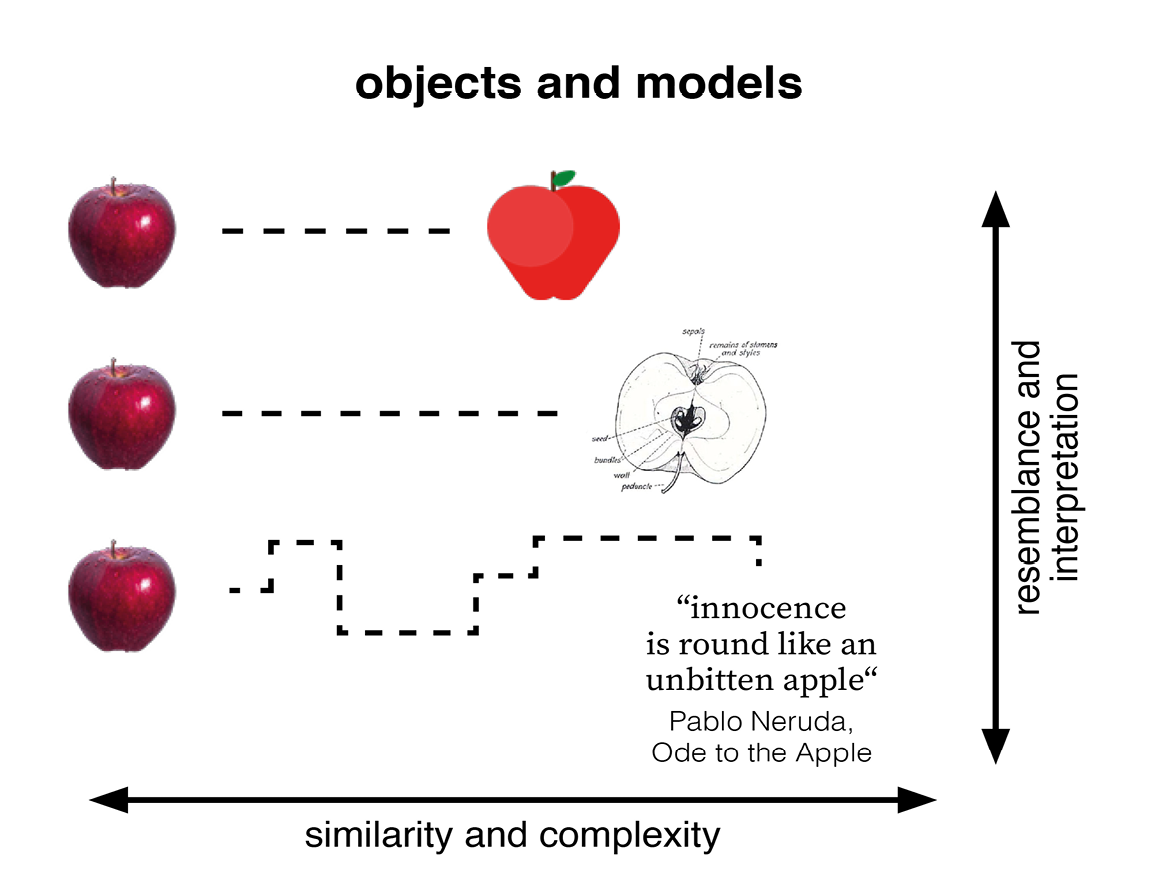
Fig. 3.4 Three grades of iconicity: apple sign in a grocery shop (image), schematic visualisation of the apple in a botanical handbook (diagram), apple as a sign of innocence in a poem (metaphor). We are well aware of the fact that ‘the object’ (as a concrete exemplary object) on the left of the diagram can itself not be represented in any other way than through ‘some’ mode of representation
(here: photographic).
Elleström claims that Peircean iconicity in the form of images, diagrams and metaphors expresses a continuum of interpretative engagements from the immediacy of resemblance to highly creative cognitive leaps (Haley 1988, p. 34). If we accept this view, it follows that grades of iconicity can function as additional operative semiotic components.
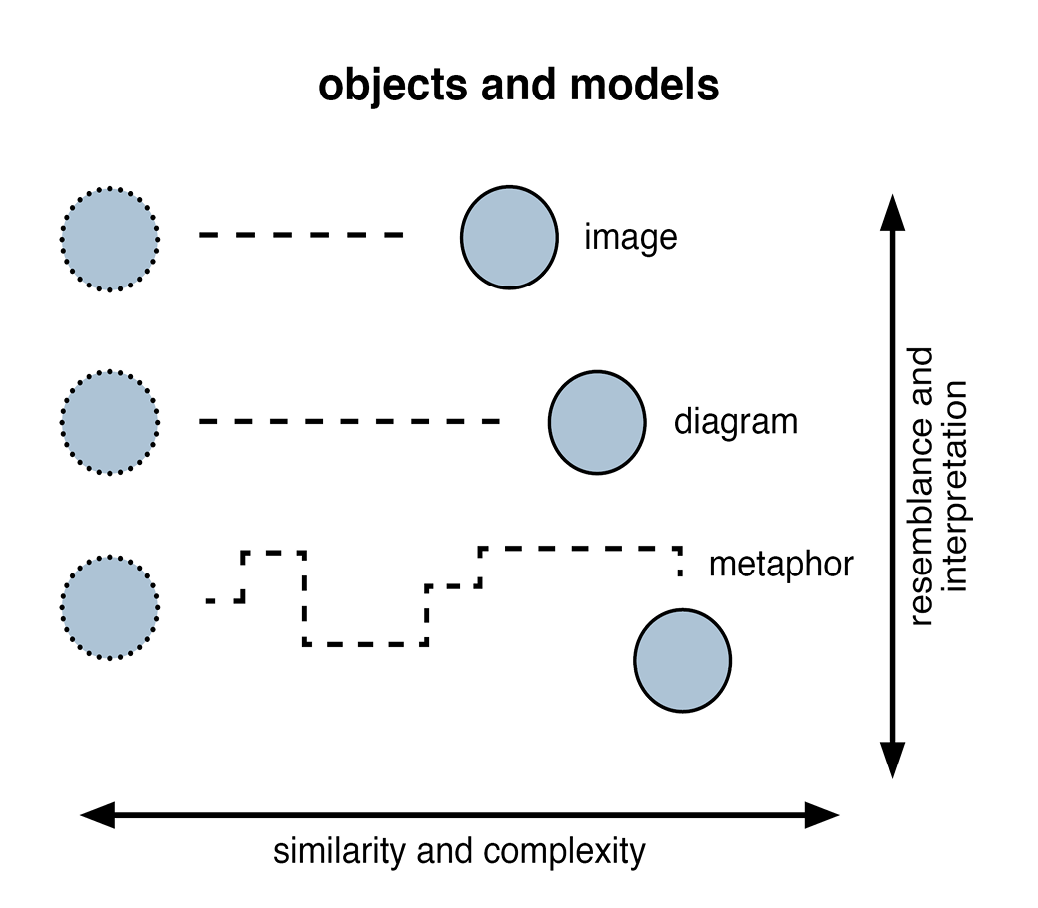
Fig. 3.5 Three grades of iconicity: icons form a scale with varying degrees of conceptual complexity. Along the scale of similarity, metaphors feature the greatest ‘distance’ from their objects compared to the structural similarity of diagrams and the immediate resemblance of images (cf. Ciula and Eide 2017, Fig. 3).
In Peirce’s original theory and in Kralemann and Lattmann’s theory, signs and models as signs do not act as signs in virtue of themselves. What establishes the model as a sign is the interpretative act of a subject, whether in the role of creator, reader, or user. Each act of modelling connects a model to its interpretation, that is, to its specific semantic content in a given social and institutional context (Kralemann and Lattmann 2013, pp. 3402–3403). The modeller’s judgement depends on his or her presuppositions connected to ‘theory, language or cultural practice’ (Kralemann and Lattmann 2013, p. 3417). Models are contingent. The iconic relationship between the model and the objects or processes being modelled (often referred to as the target of modelling) is partly externally determined (it relies on the similarity between the model and the objects or processes) and partly internally determined (it depends on theory, languages, conventions, scholarly tradition, etc.). Based on this duality, they stress, on the one hand, the subjectively determined dependency of models on prior knowledge and theory (what we above called ‘the fictionality’ of models) and, on the other, their independence from these in light of the specific conditions of what is being modelled and of what is produced in the act of modelling (which we describe as ‘the factuality’ of models and objects).
3.3.2 DH Context and Examples
As outlined above, models as signs relate to the interpretation of the objects they represent in different ways, from the immediacy of visual similarity on the image end of the iconic continuum to the conceptual similarity on the metaphorical end. In order to understand the inferential, epistemic and heuristic roles of models as sign-relations, one needs to look at both how they come to be—their context, including how we make our prior knowledge explicit and often formalised—and how the similarity with the object is used to create meaning and new knowledge. Of special interest in the discussion around modelling in DH is how iconicity can be used to unpack the nature of the relation established between sign (model) and object (often also referred to as target). As further discussed below, it can be characterised as a sort of ‘mirroring relation’ based on similarity intended broadly to encompass analogy and metaphorical thinking. When contextualised within modelling practices in DH, iconicity and its associated wide spectrum of graded approaches to reasoning seems particularly productive. Indeed, the notion of iconicity is not only about how models (as signs) appear with respect to similarity to their objects. It also encompasses the possibility (affordances) of reasoning (see Key term Model/s 2) with models while making and manipulating them.
If modelling in DH implies the translation of complex systems of knowledge into models to be processed computationally, it follows that every DH model is a diagram in that it embeds a structure, a formalism of logical and mostly mathematical nature. A digital image or a 3D model of, for instance, a historical monument can act as a surrogate of the monument or a substitute for a physical reconstruction of the real object. A diagrammatic version of the same model could be the mathematical equations underlying the graphical 3D model (Ciula and Eide 2017, p. i39). Furthermore, any operationalisation, as discussed above, formalises models into rules, from algorithms to software systems and applications; however, as discussed in Section 3.3.2, the process of abstraction (Gooding 2003, p. 280) of target objects or complex phenomena into rule-based procedures cannot be reduced to strict formalisation only. Indeed, even if the functioning of the source code of a software system through its compilation into machine code is rule-based, the design methods and data modelling processes, the writing of the source code itself, and the interactions with the interfaces experienced by the user are not. Even more evidently, the re-integration or expansion (Gooding 2003, p. 278) of modelling efforts into interpretative frameworks relies on verbal and visual language to document code and to explain the results of an experiment.100 The variations between formal representations in modelling processes can be ascribed to a more or less conscious, context-dependent adoption of defined iconic systems of representation “with determinate rules of interpretations” (Giardino and Greenberg 2015, p. 16) and “replete with rules of construction, interpretation, and even proof” (Giardino and Greenberg 2015, p. 12) which can vary in their rigour, explicitness, expressiveness, applicability and readability. Chapter 5 showcases an arbitrary selection of this variation expressed with different iconic systems which range, for example, from “Terras: Levels of Reading” and “Pierazzo: Dimensions of Text” to “Text as Expression and Content”, “Stokes: Text as Script”, “FRBR Group One: Hierarchy of Textual Entities”, “Witmore: Text as a Vector”, and “Argamon: Burrows’ Delta Formula”. While formalisation into computable models is prominent in DH research and teaching, the variation across levels of formality is less specific to DH but endemic to individual and socialised iterative modelling processes. This variation arguably contributes to the epistemic and creative potential of modelling across its contexts of use.
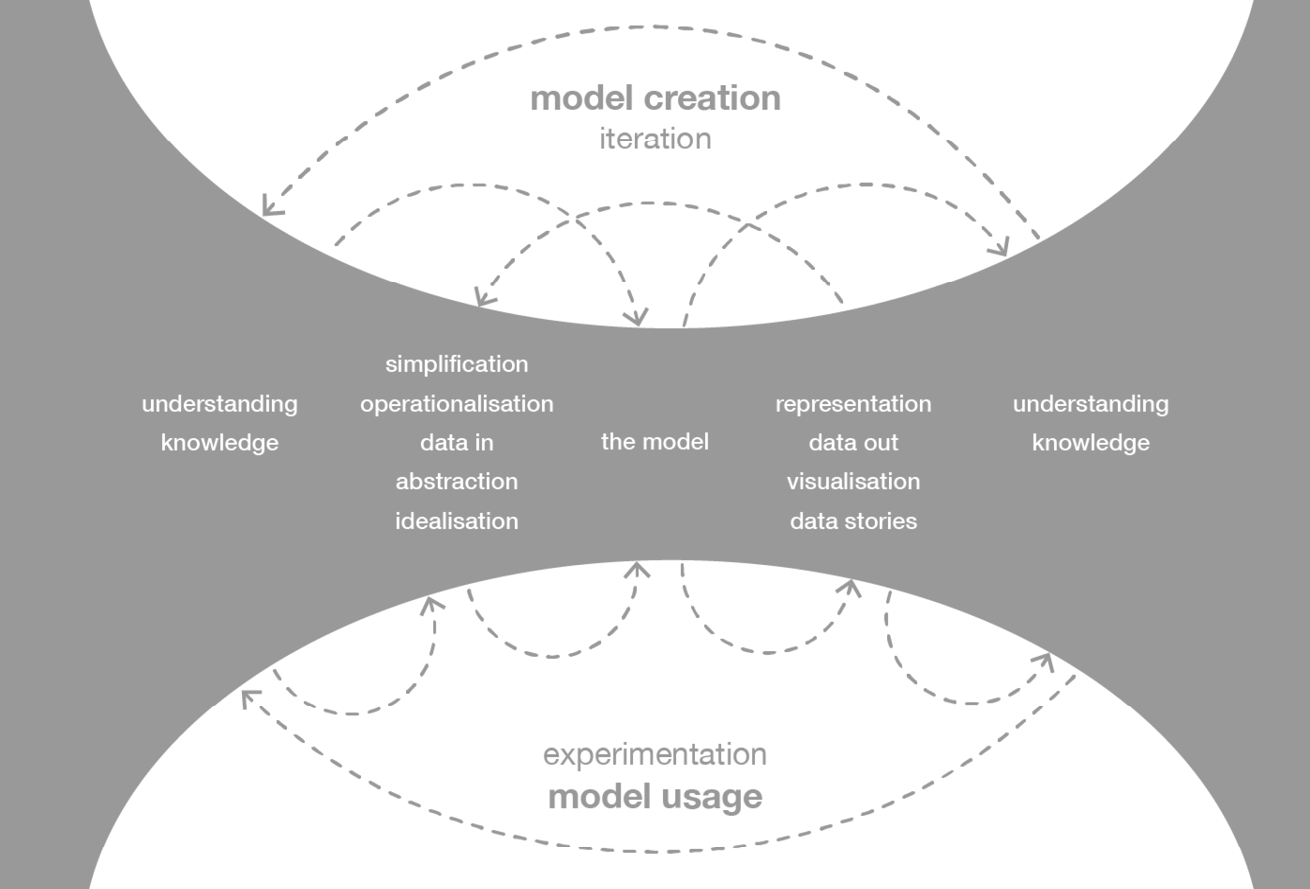
Fig. 3.6 Inspired by Gooding 2003 (Fig. 13.4) and McCarty 2018 (Fig. 1),
this graphical model serves to generically illustrate modelling as an iterative process of reduction of complex phenomena to enable quantification and manipulation on the one hand (via simplification, abstraction, etc.) and as a process of expansion (via visualisation of findings, data stories, etc.) to make the models interpretable on the other hand. It shows how computational approaches in DH can be considered analogous to other processes of abstraction, measurement and contextual interpretation in experimental settings, whereby reduction of complexity is followed by expansion in the guise of a double funnel-shaped process. Iterations occur both at the level of creation as well as usage of the model.
Building on previous work (Ciula and Eide 2017; Ciula and Marras 2019) we will exemplify how different grades of iconicity corresponding to the three model types mentioned above, namely image, diagram, and metaphor, can be identified in DH modelling activities. Three specific examples are used below to map Kralemann and Lattmann’s trichotomy of models as icons to examples of digital modelling in DH research dealing with historical artefacts. These examples are drawn, respectively, from: (1) digital palaeography research for the image-like model; (2) research on generating digital maps from historical texts for the relational or structural (i.e., diagrammatic) model; (3) a fictitious example of modelling networks of characters (person entities) featuring in art historical objects for the metaphor-like model. These prototypical cases were chosen in order to investigate how model types relate to the cultural objects they represent and how modellers reason with those models.
If we accept Kralemann and Lattmann’s argument, it follows that in modelling, we link models to qualities and relationships that may already exist in and between the objects being modelled. Such linking is based on choices which are made for a certain end, informing and motivating the act of modelling. As discussed in Chapter 1 (see also Key term Model/s 5), models are contingent, created in actual scholarly situations of production and use. A model is partially arbitrary in that the same inferences drawn by manipulating one model could have been reached in other ways, for instance using a different model.
In this framework, models operate as sign-functions, initiating a sign-relation (model-relation). To understand their epistemic role, we need to look at both how they came to be and how the similarity relation with the object is realised. By analysing the association of syntactic attributes of the source object with the attributes of the model, we focus here mainly on the latter. The focus on this representational correspondence is part of what we defined above as factuality of the models and is useful in explaining how the similarity relation is realised via the creation of objects to be experienced. To explain the semantics of the model, however, the analysis of the similarity relation needs to be complemented with an analysis of the overall sign relation (looking also at the fictionality of the model) in which production and use of models are enacted. Three examples are discussed below to analyse the three types of sign-functions and relations in a DH context.
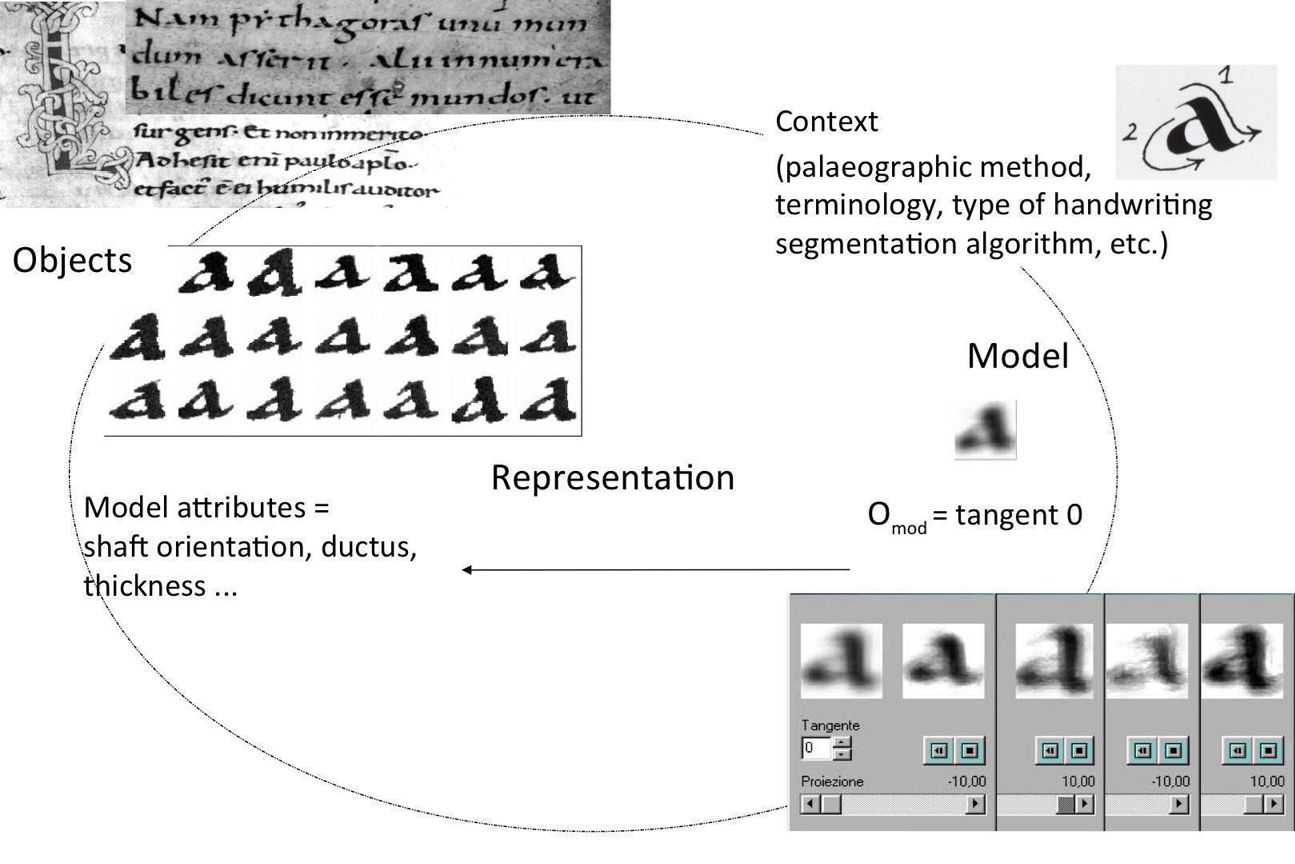
Fig. 3.7 Image-like model. Morphological features of segmented letter forms are modelled into an average morphing letter. Inferences on the manuscript handwriting are based on the analysis of the morphing letter-models by virtue of an ‘immediate resemblance’ between the original letters and the model.
The first example is taken from digital palaeography research (Ciula 2005; 2009), where the abstract model letter acts as an image-like model of the samples from which it was algorithmically generated. What we can learn about the objects of analysis (the mediaeval handwritten letterforms) depends on the features selected in the modelling process. The inferential power of this specific palaeographical model is mainly based on a strong immediate similarity (or resemblance) between model and object.101 The ‘a’ of the model looks very much like the ‘a’ of the handwriting in the manuscript, they have the same spatiality. The sign-relation hermeneutical power relies, however, on a difference in temporality between object and model. Anchoring the reasoning on spatial similarity enables us to go beyond it and learn new things about the object. Indeed, new inferences are fostered by the availability of an ‘actual’ temporal element in the morphing of the model.102 While we have to look at all single instances in the manuscript, we get a model which incorporates all variants, and by sliding from left to right, we can ‘see’ those variants in real time. The object itself, however, is not temporal in this sense. So while the model is an abstraction—a fuzzy image which loses the precision of the instances from which it was generated (the representation is indeed asymmetrical) while maintaining a basic (symmetrical) similarity—it still gains an actual temporal mode that the single-instance objects do not hold. The ability of the modeller and user of the letter models to make inferences is also based on their awareness of scribal variants and of which morphological traits are more revealing of different dating and locations than others. So context and prior knowledge are important, not only for the creation of models but also—not surprisingly—for their use and interpretation.
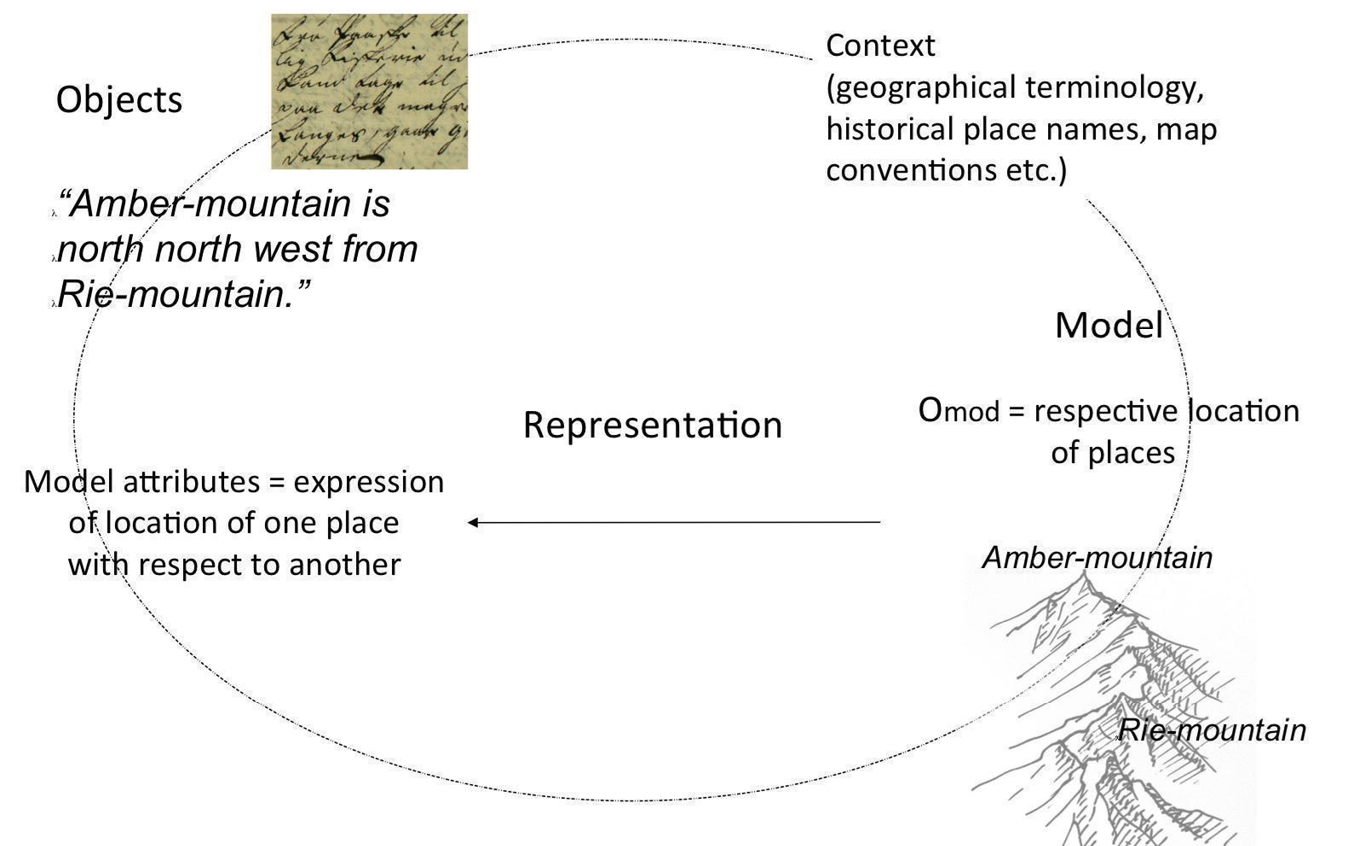
Fig. 3.8 Relational model. Relational textual expressions are modelled into geometrical relations. Inferences on space as expressed in the text are drawn by virtue of the corresponding spatial structure on the map.
The second example is taken from models of landscapes described in historical sources, where information read in texts is modelled in the form of maps (Eide 2015). The inferential power of the model relies on the analogous relational structure between the object (here: text) and the maps as model. When the text says ‘A is north of B’ it makes a claim about a geometrical relationship between places denoted in the text. A map showing A north of B makes a claim expressing a similar geometrical structure. What new knowledge we can gain about the object of analysis depends very much on the correspondence between the structuring of the textual expressions in the modelling process and the structure of the map model.
The model–object relationship here is not between an expression and a landscape but between two expressions in different media as shown in Fig. 3.8 (more on this in Chapter 4). These media express structural relationships in fundamentally different ways. To see the structural similarity, one needs to understand the written language being used in the text, the schemas used in topographical maps to convey meaning, and to have experience of real landscapes. These elements define the context of the model.
In this example, ‘similarity’ is not an immediate resemblance. The digital model—the map—looks completely different from the source object—the text. There is, however, a structural similarity with a strong hermeneutical potential between the two. It can be used to reveal gaps; there are things expressed in the text that cannot be put on the map. Examples of things that cannot be expressed include open, borderless expressions such as ‘the area north of the river’ and ambiguous expressions such as ‘Either A or B is on the border’. The analogy breaks at some point; the examples show how the signification of rich expressions in the text cannot be communicated via the structure of the map. This realisation can lead to new knowledge and a need to renegotiate what a text can mean. Based on the structural correspondence and non-correspondence between the virtual geographical space of the text and the geometrical geographical space of the map, the map makes the ‘virtual space’ ‘visible’ and in so doing reveals a dissimilarity. As explained in Chapter 4, a virtual space is the space a competent reader can establish in her mind when encountering a text. The dissimilarity between these virtual and visible spaces pinpoints the degree to which the text is spatially underspecified, that is, how open the virtual space of the text is. This forces our understanding of the text to change.
Example 3: Metaphor-like model.
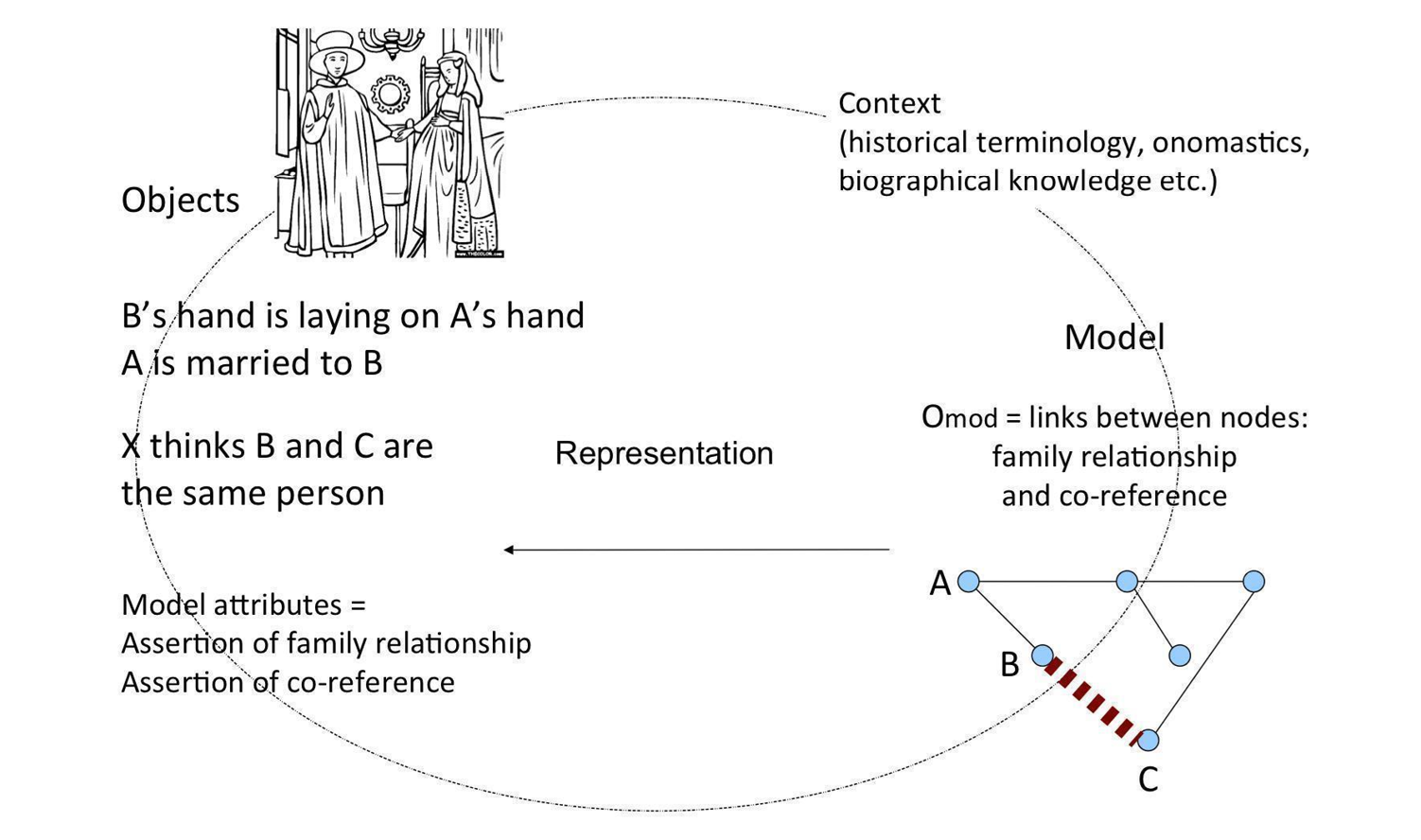
Fig. 3.9 Metaphor-like model. Person names and their relationships as referred to by a document (artwork) are modelled respectively as entities (nodes) and properties connecting them (links). Assertions of co-reference are also modelled as properties connecting entities. Thus the net is used to model social relations as well as assertions about people.
The third example is a network model designed to capture information about references to persons in historical sources. Even if this specific case is fictional (i.e., not used as it is in a research context), similar models are often used in DH research to tie specific objects (e.g., textual passages) to real-world historical entities. It is also used to form parts of co-reference networks (Eide 2009). The use of things shaped as woven networks (e.g., leaf venation, a spider, or a fishing net) or of technical networks (e.g., in telecommunication) to describe relationships between people is metaphorical. The inferential power of the model relies on a deep conceptual similarity between the model (the topography of a network) and the object (e.g., kinship of historical figures). It can generate unexpected connections between the objects it represents, which exist ‘only’ metaphorically in the context of a network-like model.
In the example in Fig. 3.9, we see the sketch of a historical picture by Jan van Eyck of a woman laying her hand on a man’s hand. The literature on readings of this fifteenth-century painting is vast. For example, one interpretation of this image sees it as a claim that the two individuals depicted are married; another suggests more subtly that the joining of arms is rather an act of presentation by the man of the picture of the child to be borne in the woman’s womb to the destinatary in the mirror, hence exhibiting the fatherhood of the painter (Lancioni 2012). Whatever the symbolic link between the figures, the physical link (the joint hands) establishes a bond between them. This bond can be associated with, and hence expressed as, a link between two nodes in a network.
There are also other types of links deduced from historical documents that can be expressed using a network model. One is co-reference, which occurs, for instance, when two person references expressed in two different statements, such as names in texts or pictures of identifiable persons, refer to the same person. A source can for instance claim that B, the person in the image, and C, a name in a text, refer to the same person. Such claims of co-reference can also be expressed as links between nodes in a network.
Both these types of links (marriage or child presentation vs. co-reference) are metaphorical. There are no strings attaching occurrences of names referring to the same historical characters to each other, and there are no familial or sentimental connections between historical persons that bear any structural similarity to the topography of a net. The social network in the model is a projection of a conceptual framework. Concepts from our understanding of social relations are combined with a sequential object, such as the textual document attesting to a wedding, and a two-dimensional painting, to form a spatial network model. Aspects of this process can be understood as a complex media transformation from the media products of the basic media types of textual document and painting to a media product of the basic media type of network, as discussed further in Chapter 4 (Section 4.5).
But the development and use of such models change our view of history; we start to see relationships as networks. The network gains hermeneutical power and makes visible as well as quantifiable aspects of a past family network or societal relations. However, different types of relationships (family vs. co-reference) easily lose their particularity and become merely links. The chain of signs becomes greedy and takes over another cognitive space or plane, which in fact deals with relations of a different semantics, in our example moving from the plane of assertion of social relations to the plane of assertion of co-reference.
One meaning can trigger others; for example, the links between entities not only connote a relationship (e.g., kinship), but their length or thickness might also be interpreted as more or less the distance between those entities (i.e., more or less related); in this sense the sign (model) takes on a life of its own. In Chapter 5, this cognitive and creative resonance in the use of metaphorical visual and verbal languages is exemplified by, amongst others, “The Keyhole Model, Frozen Text”, “The Staircase of Text”, “Text is like the Coast of England”, “Text as Kintsugi”. A link in the net is just a link, and a documented co-reference relationship becomes a supposed marriage. Context and prior knowledge influence the construction and interpretation of the model, but in turn are also influenced by it.
Reasoning Approaches
Common to all three types of model is the inferential power operating at the interplay between their “intrinsic structure” and their “extrinsic mapping” (Kralemann and Lattmann 2013, p. 3409). Indeed, the features being selected in the modelling process are influenced by contextual elements of different kinds, including hypothesis, scholarly methods and conventions, sample selection, and the technologies being used. However, the inferential and epistemic power of the model relies both on extrinsic and intrinsic aspects of the model relation. In the former case (extrinsic), the examples show us how—sometimes with vivid immediacy—a similarity of existing verifiable qualities between object and model enables DH modellers to manipulate models to make new sense of those objects. In the latter case (intrinsic), the examples show us again how models are conducive to new meaning and further modelling through our exercising of a certain imaginative freedom in selecting salient qualities to model and associating concepts.
These three examples can be matched to the following reasoning approaches:
- Morphing reasoning. In the case of the image-like model, the model is an average morphing letter generated from a semi-automatic process of selection of specific morphological features of segmented letter forms of mediaeval manuscript image samples. Inferences on the manuscript handwriting with respect to dating and localisation were based on the analysis of the morphing letter-models by virtue of an ‘immediate resemblance’ between the original letters in the manuscripts and the model.
- Corresponding reasoning. In the case of the relational model, relational textual expressions (e.g. location A is north of location B) were modelled into geometrical relations, which in turn were rendered into geographical maps. Inferences on space as expressed in the text were drawn by virtue of the corresponding spatial structure on the map.
- Metaphorical reasoning. In the case of the metaphor-like model, person names and their relationships as referred to by an art historical object (a painting in the example) are the hypothetical salient objects modelled respectively into entities (nodes) and into properties connecting them (links) in a network graph. As it happens in real DH models of social networks, the metaphor-like model of the net was used both to model the morphology of the painting (e.g. B’s hand is laid on A’s hand), the inferences on social relations (e.g. A is the wife of B) as well as the assertions of co-references about historical people (e.g. A in painting X is the same person as C in the historical document Y). This example illustrates the power of metaphorical models to extend creatively across domains, but also the potential risk of applying them inaccurately to make assumptions spanning instinctively different contexts and semantics.
3.4 What Comes Next
3.4.1 From Icons to Mediated Expressions
While many more angles that intersect DH with semiotics exist in the literature or could be explored, our argument in this chapter has addressed the following basic questions: Do models work as icons? If so, what kind of study of modelling could allow us to overcome the apparently implicit dichotomy between observer and observatum, sign and object, subject and object?
To address these questions, we followed two complementary paths: we presented modelling as a form of reasoning in practice, in alignment with Nersessian’s (2008) continuum hypothesis that modelling in science and scholarship is a special case of modelling strategies that humans adopt in everyday life; the other argument we have followed is based on semiotics, whereby models behave as icons and hence support reasoning based on a faceted notion of similarity. We have shown how both paths can be applied to DH practices and contexts. To this aim, we have placed DH and semiotics in dialogue in order to consider the central concepts of iconicity and reasoning approaches as they relate to modelling in DH. We have contextualised DH practices within a semiotic conceptualisation, which presents modelling primarily as a strategy for making sense (signification) via practical thinking (creating and manipulating models). In particular, we have focused on some aspects highlighted in Kralemann and Lattmann’s semiotic theory of models with respect to the role of ‘context’ in modelling and the nature of the ‘representational relation’ between objects and models through practical examples. We believe that these two foci point to where modelling practices in DH productively meet with a specific semiotic framework. In particular, they are useful in explaining the form or factuality of models (intended both as morphology and topology of models in addition to rule-based formality of models) and the contexts where formal (in the sense of rule-based) modelling practices are integrated with interpretative visual and verbal languages (to take account of the fictionality dimension of models). We contextualised this framework with specific examples of image-like, relational, and metaphor-like modelling in DH research. Prior knowledge is a sine qua non to create models in the first place and to use them as interpretative tools with respect to the objects they signify (Ciula and Eide 2014). The relationships between modelling processes and interpretative outcomes are neither mechanical nor directly causal (Ciula and Marras 2016); however, the type of similarity on which modelling relies shapes the interpretative affordances of those ‘anchor’ models. Modelling processes bring about investments and burdens with respect to our knowledge of the objects we model. In particular, models as signs relate to the interpretation of those objects in different ways, from the immediate similarity on the image end of the iconic continuum (Elleström 2013) to the imaginative ramifications of conceptual similarity on the metaphorical end. To understand the inferential, epistemic, and heuristic role of models as sign-relations, we need to look at both the factual and fictional aspects of models, at how they come to be and how the similarity relation with the object is used to create meaning. In summary, studying the “single respects” (Kralemann and Lattmann 2013, p. 3401; in Peircian terms “the ground of the representantem”) by which a model becomes a sign for an object is useful for explaining both the logic and syntax of DH models within specific contexts. The next chapter integrates this overarching semiotic perspective on modelling with concepts from intermedia studies. It focuses further on how models are built as well as how the relation with the object is realised by discussing models as media expressions made up of modalities and exploring modelling as a media transformation process. Before we explore these elements further, a few reflections on what we are leaving out are needed.
3.4.2 The Role of Technical Objects
First of all, the selection of salient qualities or features to exhibit in the models plays a crucial role both in the creation and interpretation of these models. However, such selection is not necessarily exclusively human-driven. In DH, we increasingly use computing algorithms to facilitate and even propose such selection, especially in complex environments where variables are manifold and interconnected (e.g. pattern recognition in image processing or textual similarity in stylometry). In deep learning systems, the complexity of, for instance, classification networks goes beyond what can be understood, explained, and made explicit with human methods of analysis because of the reliance on algorithmic modelling and computer systems as de facto black boxes even for those who develop them (see Fazi 2021). Our examples have shown how the relationship of iconicity between the model and the object being modelled is partly extrinsically determined (it relies on the similarity between the model and the object) and partly guided by intrinsic choices (it depends on theory, conventions, imaginative associations, and prior knowledge). Indeed, we have shown how the inferential power operates at the interplay between the models’ “intrinsic structure” and their “extrinsic mapping” (Kralemann and Lattmann 2013, p. 3409)103 favouring, however, a human-centred perspective.
From this exploration of the semiotics of models we have gained a different way to look at and analyse models: models as a type of sign mediating between the impressions of experience and freedom of association. While we have left out a more granular view of the socio-technical contexts of these mediations in the examples we used, a holistic, semiotic and pragmatic understanding of modelling imply that humans, technical objects (Smithies, ffrench and Ciula 2023) and computational agents co-exist in, have agency in (Fazi 2021), and therefore co-create this environment.
By reflecting on some DH examples of modelling, we have shown that a semiotic understanding of modelling could ultimately allow us to surpass the duality of object vs. model (as well as sign vs. context). Semiotic concepts applied and used in a DH context acquire a specific ‘meaning’ and a semiotic approach gives high prominence to a dynamic view of models and modelling which reinstates the understanding of modelling as an open, indeterminate process in renewed terms, as a creative process of thinking and reasoning where meaning is made and negotiated through the creation and manipulation of external (mainly iconic) representations, combined with an imaginative use of formal and informal languages. Different styles of reasoning and modes of thought, including algorithmic modelling, can contribute to this process but their incommensurability in terms of human explanation (Fazi 2021) remains unresolved and at the moment more or less critically integrated via processes of human signification and interpretability (corresponding to Gooding’s expansion efforts). Ultimately, a semiotic approach facilitates the integration of a “techno-scientific” perspective on modelling with a “humanistic” one. Indeed, if acts of modelling—inclusive of the technical apparatus via which they are operationalised and interpreted—revisited from a semiotic perspective are used to make sense of our cultural objects, they are meaning-making practices and hence in themselves objects of study for the humanities.
3.4.3 Variety and Creativity
Compared to other contexts,104 in DH, ’models and stories’ coexist and are not in opposition; formal and experimental modelling techniques are combined with a constructive use of programming, verbal, and visual languages. We touched on how metaphorical modelling can lead to the creation of project narratives in Chapter 2. In Chapter 5 we collected examples of visual models which in some cases inspired, influenced or directly resulted in computational models. In Section 3.2.2 and elsewhere (Ciula et al. 2023), we have reflected on how modelling iterations typically adopt different levels of abstraction and different languages of expression within a collaborative setting such as a research software engineering team. However, a focus on the variety and creativity potential of models is a line of enquiry that would require further research.
82 This chapter is built extensively on: Ciula and Eide (2017) and Ciula and Marras (2018).
83 From 2009 to 2013 the working group “Reference Curriculum for the Digital Humanities” worked towards defining a common methodological core for the field. In essence, it identified exactly these two aspects as core areas that are central to all work in DH. First, “modelling” is defined as making explicit and computable research questions, their domains and pertinent data, and second “formalisation” as finding algorithms and software solutions to process these models and their data. For more details see Sahle (2013, p. 20).
84 ‘Hypoicon’ is a technical term introduced by Peirce in order to define ‘iconic signs/representamen’ which rely on shared rules to be decoded and understood as distinct from ‘pure icons’ (which, if that were possible, could be understood without referring to any cultural or conventional rules): “But a sign may be iconic, that is, may represent its object mainly by its similarity, no matter what its mode of being. If a substantive be wanted, an iconic representamen may be termed a hypoicon. Any material image, as a painting, is largely conventional in its mode of representation; but in itself, without legend or label it may be called a hypoicon” (2.276).
85 Models for and models of are Janus concepts discussed extensively in the literature (e.g. Geertz 1973 and, in DH literature, McCarty 2005). According to Mahr (2009) they embed relational aspects present in all models, even if the respective emphasis varies: “[F]rom the perspective of creation, the model object may be viewed solely as a model of something, whereas from a perspective of application, it may be viewed solely as a model for something. However, this does not affect the circumstance that the judgement on model-being is justified only if the model object is linked to both constructive relationships, at least in a mental sense” (p. 372).
86 A multi-disciplinary approach to the early modern world of material culture can be found in: Early Modern Things: Objects and Their Histories, 1500-1800, ed. by: Paula Findlen (2012); The point of view of the science in the definition of the objects and the role of the (disappearance) of the observer in Gal and Raz (2012).
87 An example of how such processes can be understood and analysed in the light of media transformations can be found in Chapter 4.
88 Jerome McGann has articulated this framework extensively over the years and made it relevant also to a DH research context (e.g. McGann 2014).
89 Note that we are implying a DH informed mainly by textual scholarship and de facto excluding other traditions, for example in computational archaeology, a community that mostly does not recognise itself under the DH label. Some examples from archaeology are included in Chapter 4.
90 This is the case if we accept a wide definition of what the objects of study for the humanities at large are: “The humanities study the meaning-making practices of human culture, past and present, focusing on interpretation and critical evaluation, primarily in terms of the individual response and with an ineliminable element of subjectivity.” (Small 2013, p. 57).
91 Modelling as a research methodology is deeply intertwined with DH research. Statistical analysis of texts, the development of experimental tools to study visual objects, critical mapping, and many other important parts of DH research all include modelling as an important methodology together with other practices and approaches.
92 As in Chapter 2, we refer to Morgan’s definition of ‘formal’ which she applies to modelling studied within the social science context of economics. Models are formal in at least two senses as proposed by Morgan (2012, pp. 19–20): (1) models give form and shape to ideas; (2) models make ideas rule-bound.
93 For example, in an interactive design context: Cooper, Reinman and Cronin (2007); see the comprehensive overview of process models in design and development by Wynn and Clarkson (2018).
94 For an in-depth discussion on the role of formal models and stories in economics, see Morgan and Knuuttila (2012). In computer science and user-centred design, the methodology connected to user’s stories is an example of a similar phenomenon. For reflections on modelling iterations combining different levels of formality in a DH research software engineering context, see Ciula et al. (2023).
95 On Software Intensive Research in DH, see Smithies (2017), pp. 113–151.
96 See for example Guarino, Guizzardi, Mylopoulos (2019) and Mayr and Thalheim (2021) as recent excellent references on conceptual modelling in these respective areas.
97 Note that there has been a significant development over time in Peirce’s thinking, with further extensions of the system presented in very simplified form in this chapter.
98 The distinction between the three types of hypoicons is not ontologically clear-cut. We follow Elleström (2013) amongst others in seeing these types as grades in a ‘continuum’ or even as a development rather than separate categories.
99 For a recent detailed and comprehensive overview of Peirce’s categories and taxonomy of signs, see Olteanu (2015, pp. 61–79).
100 These are also media transformation processes. What can be expressed varies in the different media, as discussed further in Chapter 4.
101 In Chapter 4, we will see how this could be unpacked further by stating that the similarity is first and foremost of a spatial nature: the handwritten letter is a two-dimensional spatial object as its spatial model is. However, their temporalities are different. We encounter single instances of letters in the manuscript pages, while the morphing models as shown in Fig. 3.7 incorporate variants that can be visualised in sequence.
102 This morphing model expresses a ‘less fixed temporal mode’ than the object it represents (cf. Chapter 4 Sections 4.2 and 4.4).
103 A challenge for a research agenda in this area would be to explore how the interplay between intrinsic structures of models (selection of salient qualities) and extrinsic mapping (their iconic ground) develops in the creation of scholarly arguments in the humanities.
104 This aligns with the discussion about metaphorical models in the sciences (e.g. Wolynes 2001) and models as fiction in natural science modelling. See Suarez (2009) for an overview with different positions represented.