7. Discussion Trees on
Social Media
A New Approach to Detecting Antisemitism Online
©2024 Chloé Vincent, CC BY 4.0 https://doi.org/10.11647/OBP.0406.07
Antisemitism often takes implicit forms on social media, therefore making it difficult to detect. In many cases, context is essential to recognise and understand the antisemitic meaning of an utterance (Becker et al. 2021, Becker and Troschke 2023, Jikeli et al. 2022a). Previous quantitative work on antisemitism online has focused on independent comments obtained through keyword search (e.g. Jikeli et al. 2019, Jikeli et al. 2022b), ignoring the discussions in which they occurred. Moreover, on social media, discussions are rarely linear. Web users have the possibility to comment on the original post and start a conversation or to reply to earlier web user comments. This chapter proposes to consider the structure of the comment trees constructed in the online discussion, instead of single comments individually, in an attempt to include context in the study of antisemitism online.
This analysis is based on a corpus of 25,412 trees, consisting of 76,075 Facebook comments. The corpus is built from web comments reacting to posts published by mainstream news outlets in three countries: France, Germany, and the UK. The posts are organised into 16 discourse events, which have a high potential for triggering antisemitic comments. The analysis of the data help verify whether (1) antisemitic comments come together (are grouped under the same trees), (2) the structure of trees (lengths, number of branches) is significant in the emergence of antisemitism, (3) variations can be found as a function of the countries and the discourse events.
This study presents an original way to look at social media data, which has potential for helping identify and moderate antisemitism online. It specifically can advance research in machine learning by allowing to look at larger segments of text, which is essential for reliable results in artificial intelligence methodology. Finally, it enriches our understanding of social interactions online in general, and hate speech online in particular.
1. Introduction
While research on automatic detection of hate speech is a growing field, the focus on antisemitism is rarer in comparison to other hate ideologies. Unlike other forms of hate speech, antisemitism has always changed and adapted to conditions throughout its history (Wistrich 1992). In the awareness of the Holocaust, it is often expressed implicitly in mainstream public discourse and is therefore making it difficult to detect automatically. This is also the case in social media contexts of the political mainstream, where antisemitism is generally not accepted.
Previous quantitative work on antisemitism online has focused on independent comments obtained through keyword search (e.g. Jikeli et al. 2019, Jikeli et al. 2022b). These studies often ignored the discussions in which they participated; the comments were analysed independently of this context. This poses a problem because discussions on social media are rarely linear: web users have the possibility to comment on the original post and start a conversation or to reply to earlier web user comments.
In many cases, context is essential to recognise and understand the antisemitic meaning of an utterance (Jikeli et al. 2022). In our corpus, more than half of the comments that were annotated as antisemitic could be considered as such by taking the context into account, that is, by considering either the article to which the comment refers or the comments to which the web user is replying. For instance, a simple comment with only the word “who?” would not be considered antisemitic in most contexts. However, in the French context of the 2021 protests against the health pass during the Covid-19 pandemic, “who?” [“qui?”] can be understood as a dog whistle―an antisemitic coded phrase which implies that Jews are controlling the world and are responsible for the pandemic. Being able to refer to the article in order to evaluate the comments might help categorise the comment accordingly. Another example is the antecedents of pronouns. In a discussion, if one user mentions Jewish people then the next makes a reference to the previous comments using pronouns (for example, “They are evil”), the pronoun’s meaning can only be understood using the contextual information provided by the previous comment. Therefore, within our corpus, more than half of antisemitic comments (56%) could not have been categorised as such if context had not been taken into account.
My contribution aims at exploring new ways of handling social media data with the goal of adding context to the short texts that constitute comments. Taking a data-based approach, it examines how antisemitic comments are distributed, how the online discussions are structured and how the patterns observed vary depending on discourse event and country.
In this chapter, I first present the dataset I studied by describing the collection and its processing. I then move on to answer the following three research questions: (1) are antisemitic comments more likely to be grouped under the same trees, (2) is the structure of trees (lengths, number of branches) significant in the emergence of antisemitism and (3) are there any variations depending on the countries and the discourse events? I consider the structure of the comment trees constructed in the online discussion, instead of single comments individually, in an attempt to include context in the study of antisemitism online. The results of the statistical models are presented, followed by a discussion.
2. Data collection
This study uses the data collected in the context of the ongoing project Decoding Antisemitism in the period between June 2021 and December 2022. The data was obtained by collecting comments reacting to news articles published in the context of specific discourse events. The discourse events are delimited by the research teams in preparation of the data collection. The discourse events are chosen according to whether the articles from the mainstream media reporting on them are potentially triggering antisemitism and whether they generate a large enough online discussion (at least one or two posts per news outlet, for which at least fifty comments were posted). The delimitation of which articles will be included in the analysis varies from one discourse event to the other. The articles must fit the topic, the period in time and in case the discourse event triggered large discussions we focus on articles that had more comments.
Some discourse events studied in this chapter are international, such as the Russian invasion of Ukraine, the Arab-Israeli conflict, the Covid-19 vaccination campaign in Israel, the terrorist attacks perpetrated in Israel in the spring of 2022 and the company Ben & Jerry’s decision not to sell their products in Israeli settlements. Others are country specific, for instance the reactions to the emergence of antisemitic slogans in the demonstrations against the health pass, the use of the Pegasus spyware (developed by the Israeli cyber-arms company NSO Group) to spy on various French politicians, the ban of both the comedian Dieudonné M’bala M’bala’s and the political essayist Alain Soral’s Facebook and YouTube accounts in France, the trials of the concentration camps guards, the Gil Ofarim and Maaßen controversy in Germany, the case of the Irish novelist Sally Rooney who refused permission for an Israeli publishing company to translate her best-selling novel Beautiful World, Where Are You into Hebrew as part of a cultural boycott of Israel and the claims made by Professor David Miller, who alleged that the students from the University of Bristol’s Jewish Society were “political pawns by a violent, racist foreign regime engaged in ethnic cleansing” in the United Kingdom (Liphshiz 2021). Once the discourse event is clearly delimited, all articles and social media posts that meet the selected criteria are crawled (that is, collected and downloaded from their source website) to gather all comments reacting to them, in the order they appear online.
For each discourse event, the research team from Decoding Antisemitism annotated the comments using the software MAXQDA, following a guidebook. The comments are annotated not only for ideation―that is, whether the comment is (contextually) antisemitic, countering antisemitic speech, or not antisemitic―but also for linguistic characteristics, antisemitic tropes and mentions of Jewish people, Jewishness or Israel.
2.1 Contextual antisemitism and mentions
Previous studies examining antisemitism online have used keyword search, ignoring most of the contextual antisemitism. Figure 7.1 shows that, in our corpus, while antisemitic comments are found most often when Jewish people, Jewishness or Israel is mentioned, context is still essential to understand the antisemitic meaning of the comment in 26% of cases. More importantly, even though our corpus is built by focusing on discourse events likely to trigger antisemitism, the vast majority of comments do not explicitly mention Jewish people, Jewishness, Israel or related words and phrases (40,547 comments, compared to 6,384 that include such mentions), and therefore would not be found by keyword search. Context is essential to understand the antisemitic meaning in 67% of these cases.
Figure 7.1: Distribution of comments in the Decoding Antisemitism dataset, depending on their ideation and on the presence of specific mentions. Note that the scale varies between mentions and no mentions
3. Data processing
The annotated data is exported from the MAXQDA content analysis software to the CSV (comma-separated values) file format and then processed in the statistical analysis programme R. The complete dataset consists of 76,075 comments. This chapter focuses on Facebook data, which represents 54,215 comments taken from 371 posts. In order to avoid outlier skews, I eliminated the 5% shortest and 5% longest threads; a thread is defined, for the purpose of this study, as the totality of comments annotated under a specific post, regardless of the structure of the conversation. This elimination resulted in a dataset of 333 threads containing from 71 to 256 comments (with the mean of 141 and a median of 117). In total, the threads comprised 46,931 web comments, out of which 6,484 were considered antisemitic (either explicitly or contextually).
In general, the threads below the posts are constituted of trees of comments. Some trees are very short; these might contain only one comment to which no other web user replied. Others are composed of multiple comments, organised in branches. On Facebook, web users wishing to comment under a post have two options. They can either post their comment directly in reply to the initial post, or they can reply to another comment. The direct-response comments form the trunk of the trees, while the replies are their branches. Replies are restricted to a depth of two levels―the first level being a user’s reply to the initial comment (the trunk) and the second level a user’s reply to another reply.
In order to analyse the data, the comments were grouped together by trees. Within each tree, the proportion of antisemitic comments is computed as well as the length of the tree―single comments that received no replies are of length 1―and the number of branches or replies to the initial ‘trunk’ comment. For the purpose of this chapter, I use the term ‘discussion’ to refer to a succession of comments responding directly to one another, as opposed to responding directly to the post published by the news outlet on their social account; a discussion is a tree of length greater than 1.
For our three research questions, I formulate the following hypotheses:
- Antisemitic comments are grouped together:
- The proportion of antisemitic comments is higher in discussions than in single comments (H1)
- Replies to comments are more likely to be antisemitic than replies to media posts (H2)
- Discussion starting with antisemitic comments are more likely to trigger antisemitism (H3).
- The structure of trees is related to the emergence of antisemitism:
- The longer the trees, the higher the proportion of antisemitism (H4)
- The larger the trees, that is to say, the more branches there are, the higher the proportion of antisemitism (H5).
- There is variation depending on the countries and the discourse events (H6).
4. Statistical analysis
In order to answer the three research questions laid out in the introduction, I build statistical models using the ideation of the comment, simplified to antisemitic versus not antisemitic, as the dependent variable. The independent variables differ depending on the research question I try to answer. Given that the dependent variable can only take two values (either antisemitic or not), I run a generalised linear model in R with a binomial family.
4.1 RQ1: Are antisemitic comments grouped together?
The first research question deals with whether more antisemitic comments can be found in a conversation about the topic that has been identified as a potential trigger for antisemitic reactions, as opposed to stand-alone comments. Three hypotheses were made in this regard: (H1) there are more antisemitic comments in discussions than in single comments, (H2) initial comments are less likely to be antisemitic than replies to comments and (H3) in case an antisemitic comment triggers the discussion, antisemitic comments are more likely to be found in the corresponding discussion.
H1: In order to verify whether the proportion of antisemitic comments is higher in the discussions than in the single comments, I built a linear model using the simplified ideation of the comment (‘antisemitic’ versus ‘not antisemitic’) as the dependent variable, and the type of tree the comment is in as independent variable. The type of tree determines whether the tree comprises a single comment or a discussion (that is, at least one reply to the initial comment).
Table 7.1 shows the results of the statistical analysis. The p-value (<2e−16) stands for the probability of observing this result due to chance. A p-value lower than 0.05 is widely taken as threshold for significance. The type of tree is thus a significant variable in determining if a comment is more likely to be antisemitic. The estimate corresponds to the log odds of a comment being antisemitic. The intercept is the basis (here a single comment), and the value for the discussion is the estimated difference for the log odds in case the tree is a discussion. The odds of a comment being antisemitic decrease when in a discussion in comparison to single comments: Figure 7.2 shows that, contrary to my first hypothesis (H1), comments in a discussion are less likely to be antisemitic than single comments (note that the scales on the y axis differ: there are three times as many comments in the discussion than there are single comments). In discussions, 13% of the comments are antisemitic, whereas 17% out of the individual comments are antisemitic.
Estimate |
Standard error |
P-value |
|
(Intercept) |
−1.59 |
0.02 |
<2e−16 *** |
Type of tree (discussion) |
−0.32 |
0.03 |
<2e−16 *** |
Table 7.1: Results of the statistical analysis modelling the ideation as a function of the type of comment. The three stars indicate the high significance of this relation.
Figure 7.2: Ideation of comments from the Decoding Antisemitism dataset depending on whether the comment is an individual comment, or taken from a discussion. Note that the scale varies between Discussion and Single comment.
H2: Another possible approach to this question is to regard comments as sequential, in opposition to the end results that were crawled. We can understand all heads of trees to be single comments, and assume that trees only start with the replies to the initial comments, instead of considering the trees a posteriori.
Figure 7.3 shows a coherent result with reply to comments being significantly less likely to be antisemitic than initial comments: thus, the second hypothesis (H2) is also invalidated. Of the initial comments, 18% are antisemitic (Level 0) compared to 12% and 11% for the direct and indirect replies (Level 1 and 2 respectively), which are not significantly distinct. Table 7.2 shows the estimate of the statistical analysis, together with the p-value. The diagonal corresponds to the log odds for a comment being antisemitic for each level. The rest of the table indicates the estimated difference for the log odds between the different level of comments. The odds of a comment being antisemitic decrease when the level increase, but the difference between level 1 and 2 is not significant.
Figure 7.3: Distribution of comments depending on the levels. Note that the scale varies between the different levels.
Estimate (p-value) |
Level 0 |
Level 1 |
Level 2 |
Level 0 |
−1.50 (<2e−16 ***) |
||
Level 1 |
−0.54 (<2e−16 ***) |
−2.04 (<2e−16 ***) |
|
Level 2 |
−0.59 (<2e−16 ***) |
−0.05 (0.198) |
−2.09 (<2e−16 ***) |
Table 7.2: Results of the statistical analysis modelling the ideation as a function of the level of the comment.
H3: To evaluate the hypothesis that discussions beginning with an antisemitic comment are more likely to trigger antisemitism, I ignored the single comments since their ideation automatically matches the initial comment of the tree, which, in this situation, is of length 1.
As shown in Figure 7.4, antisemitic comments are more likely to be found in a discussion that started with an antisemitic comment. In such discussions, 28% of comments in the dataset are antisemitic, compared to 9% for discussions starting with a non-antisemitic comment. Table 7.3 presents the results of the statistical model, showing that the ideation of the initial comment of a discussion is significant in explaining the variation of the comments’ ideation and that the odds of a comment being antisemitic increase when the initial comment of the tree is antisemitic.
Figure 7.4: Distribution of ideation of comments in discussions, depending on the ideation of the first comment of the tree
Estimate |
Standard error |
P−value |
|
(Intercept) |
−2.36 |
0.02 |
<2e−16 *** |
Ideation of the initial comment (antisemitic) |
1.39 |
0.03 |
<2e−16 *** |
Table 7.3: Results of the statistical analysis modelling the ideation of a comment as a function of the ideation of the initial comment of the tree.
To conclude, I invalidated the first two hypotheses, both of which claim that antisemitic comments are more likely to appear in discussions. However, the analysis revealed a new correlation: when a discussion starts off with an antisemitic comment, it is more likely that more antisemitic comments will follow. I review this result further in the discussion section.
4.2 RQ2: Does the structure of trees reflect the proportion of antisemitism?
For the second research question, I focused on the discussions in the corpus and ignored single comments; that is to say, I concentrated on trees that contain at least two comments. Two hypotheses were formulated regarding the structure of the trees: that the proportion of antisemitic comments increases as the conversation grows in length (H1), and in width (H2). The width refers to the number of branches started by replies (Level 1) to the initial comment (Level 0), while the length is the overall number of replies (Level 1 and 2) to the initial comment (Level 0).
H4: Aligned with the results from the previous research question, I found that the proportion of antisemitism decreases with the length of the discussion. These results show that the discussion does not trigger more antisemitism as it develops.
The fourth hypothesis (H4) was, therefore, invalidated. On the contrary, the median of tree lengths for antisemitic comments is 7, while it is 9 for non-antisemitic comments. Given the variation in the length of comment trees, as shown in Figure 7.5, it is not the best indicator of the variation between antisemitic and non-antisemitic comments. Table 7.4 shows that, while the length of the tree is not significant (p value 0.856) in explaining the variation of the odds, the estimated variation is very small in any case (0.0001 per additional comment in the tree).
Figure 7.5: Distribution of the length of trees for antisemitic and non-antisemitic comments.
Estimate |
Standard error |
P−value |
|
(Intercept) |
−1.96 |
0.02 |
<2e−16 *** |
Length of tree |
0.0001 |
0.0005 |
0.856 |
Table 7.4: Results of the statistical analysis modelling the ideation of a comment as a function of the length of the tree.
H5: Regarding another element of the structure of trees, I studied the potential effect of the number of branches of a discussion tree on the probability of finding antisemitic comments. When focusing only on comments that are part of a discussion, that is to say where there is at least one branch of replies, I found that (1) the relationship is significant in explaining the variation in the data and (2) the more branches there are, the more likely it is to find antisemitic comments (cf. Table 7.5).
Estimate |
Standard error |
P−value |
|
(Intercept) |
−2.01 |
0.02 |
<2e−16 *** |
Length of tree |
0.007 |
0.001 |
1.2e−05 *** |
Table 7.5: Results of the statistical analysis modelling the ideation of a comment as a function of the number of branches in the tree
The variation is so small, however, in comparison to the variation in the data (cf. Figure 7.6), that I could disregard its use in distinguishing between these parameters. The median of branch numbers in both cases is 4.
Figure 7.6: Distribution of the number of branches in a discussion tree for antisemitic and non-antisemitic comments
To conclude, I found that the elements of the structure of the trees that I examined are not significant in representing the proportion of antisemitism in the discussion. The length of the discussion in not significant, while the number of branches is significant in showing there is no evolution depending on the width of the conversation.
4.3 RQ3: Is there variation depending on the countries and the discourse events?
Finally, the question remains whether the structure of the conversation varies between the three language communities under analysis. I found that there are slightly more discussions in the UK (26%) and France (24%) compared to Germany (22%), as shown in Figure 7.7 and Table 7.6.
Figure 7.7: Discussions and single comments depending on the country. Germany differs significantly from France and the UK. The variation between France and the UK is less significant. Note that the scale varies between the countries.
Estimate (p−value) |
Germany |
UK |
France |
Germany |
1.26 (<2e−16 ***) |
||
UK |
−0.19 (3.4e−11 ***) |
1.07 (<2e−16 ***) |
|
France |
−0.13 (1.5e−05 ***) |
0.06 (0.02 *) |
1.13 (<2e−16 ***) |
Table 7.6: Results of the statistical analysis modelling the odds of a comment being in a discussion as a function of the speech community
The lengths of the trees across the three country datasets are similar (median are 8, 9 and 10 for Germany, the UK and France respectively) and do not vary significantly.
Regarding the effect of the initial comment on the rest of tree, the statistical analysis shows that both variables (the country and the initial comment), and their interactions are significant, as shown in Figure 7.8 and Table 7.7. The effect of the initial comment is most important in the German corpora and least important in the British corpora.
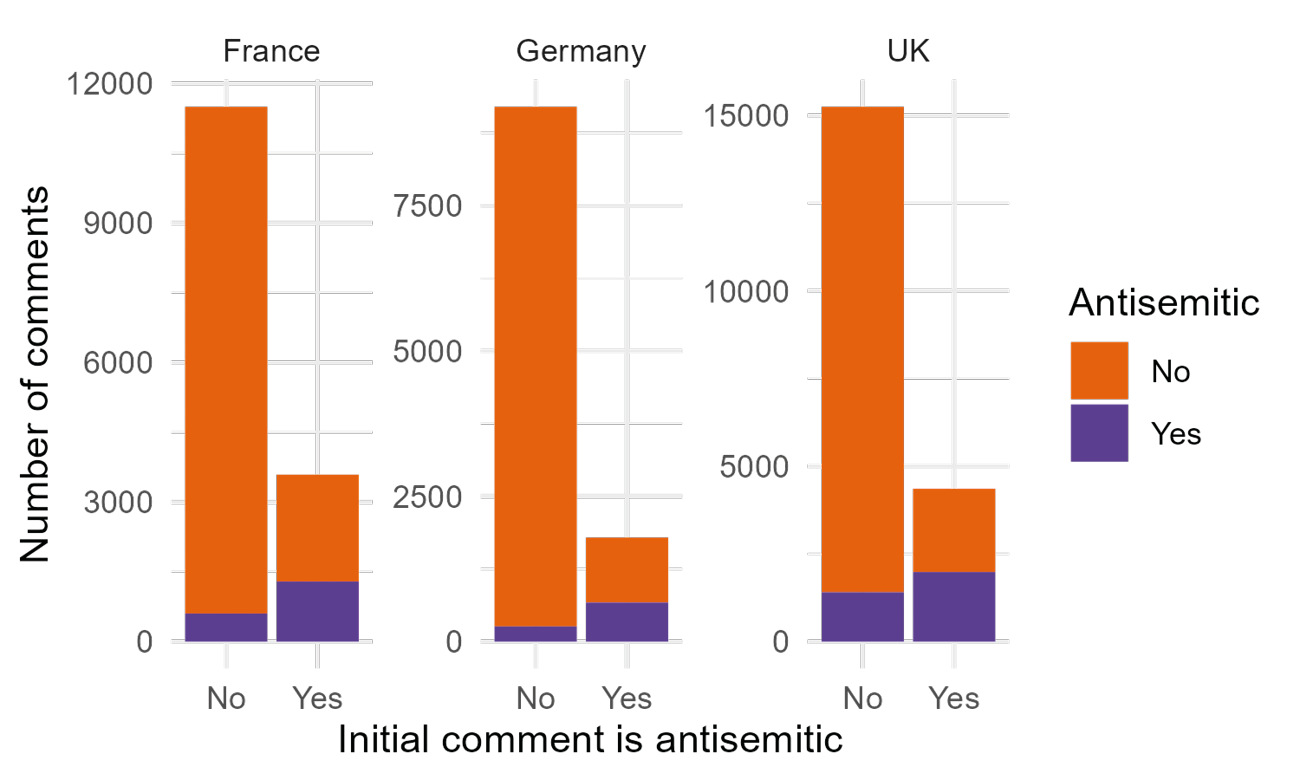
Figure 7.8: Interaction between the country and the initial comment ideation in determining the ideation of a comment. Note that the scale varies between the countries.
Estimate (p−value) |
Germany |
UK |
France |
Germany |
−3.48 (<2e−16 ***) |
||
UK |
1.21 (<2e−16 ***) |
−2.27 (<2e−16 ***) |
|
France |
0.61 (<2e−16 ***) |
−0.60 (<2e−16 ***) |
−2.87 (<2e−16 ***) |
Ideation of the initial comment (antisemitic) |
3.05 (<2e−16 ***) |
2.13 (<2e−16 ***) |
2.25 (<2e−16 ***) |
Interaction between initial comment and Germany |
0.92 (<2e−16 ***) |
0.70 (8.1e−14 ***) |
|
Interaction between initial comment and UK |
−0.22 (0.001 **) |
Table 7.7: Results of the statistical analysis modelling the odds of a comment being antisemitic as a function of the interaction between the speech community and whether the initial comment of the tree is antisemitic
Overall, the proportion of antisemitic comments varies significantly from one country to the other: 18 % in the UK, 13 % in France and 9 % in Germany as shown in Figure 7.9 and Table 7.8. I also observed that there are many more non-contextual antisemitic comments in the UK corpus than were found in the French and German corpora.
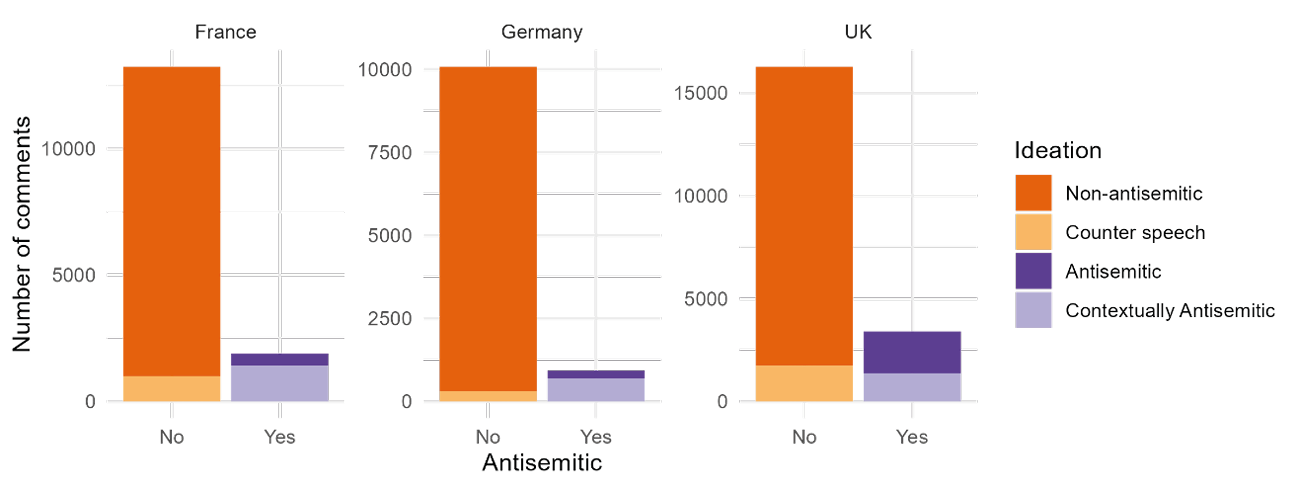
Figure 7.9: Overall distribution of comments in the three countries. The three countries differ significantly from one another (p value is <2e−16 for all relations). Note that the scale varies between the countries.
Estimate (p−value) |
Germany |
UK |
France |
Germany |
−2.31 (<2e−16 ***) |
||
UK |
0.77 (<2e−16 ***) |
−1.54 (<2e−16 ***) |
|
France |
0.41 (<2e−16 ***) |
−0.36 (<2e−16 ***) |
−1.90 (<2e−16 ***) |
Table 7.8: Results of the statistical analysis modelling the odds of a comment being antisemitic as a function of the speech community
5. Discussion
The statistical analysis shows that antisemitic comments are not distributed in a specific pattern in the examined corpus. Whether or not antisemitism is present in a discussion is not reflected in the structure of the trees of the online conversation. Contrary to our hypotheses, the longer a discussion continues, finding antisemitic comments does not become more likely and replies to the initial comments are less likely to be antisemitic. Antisemitic comments are more numerous in single comments than in the discussions.
This result shows that conversations around potentially triggering topics do not necessarily lead to antisemitism in higher proportions as discussion develops. However, in the process of analysis, I found that replies in discussions with an antisemitic starting point―ones in which the initial post in a comment tree is antisemitic―are more likely to contain antisemitism than those in discussions that start with a non-antisemitic statement. This could be due to the fact the web user who opens the discussion is more likely to comment further in the discussion, continuing to express either the same or new antisemitic tropes, which will then lead to increased probability of finding an antisemitic comment in that discussion. Moreover, comments on public posts―such as the ones under scrutiny in the Decoding Antisemitism project―are visible not only to any Facebook user exploring the thread but also on timelines of Facebook friends of that user (depending on their privacy settings). This visibility may trigger response from a specific social group influenced, in the same way as the initial user, by antisemitism.
Thus, while it seems that conversation on social media in itself does not trigger greater antisemitism, these platforms are conceived and built in a way that will lead to the comments attracting web users who hold similar world views and, thus, amplifies antisemitism online.
Another finding of this study is the variation between the speech communities both in terms of the structure of the discussion and in terms of antisemitic content. I found that the overall proportion of antisemitism is much higher in the data collected from the comment sections of news outlets in France and the United Kingdom compared to those in Germany.
I can only speculate on the reasons for the variation between the three countries. One potential explanation is that there is less antisemitism in German society than in France and the UK. One can suppose that, contrary to France and the UK, the memory work done by the German society in the past decades has led its web users to understand better what constitutes antisemitic statements, to recognise antisemitic stereotypes and concepts and to grasp why they are harmful. There is, of course, no denying that there is still antisemitic hate speech in Germany, but the findings here might be an invitation to educate society at large.
Another reason, related to the above, may be a difference in the countries’ moderation policies: these might be stricter in Germany and more permissive in the UK and in France.
The third potential reason is that French and English, unlike German, are global languages. Both are spoken by hundreds of millions of people around the world. The proportion of antisemitism in the comments does not represent only the British and French society respectively but, rather, the language communities linked to, or under the sphere of influence of, the two countries.
In the UK data, I found that many more discussions starting with non-antisemitic statements still triggered a considerable amount of antisemitic content, and discussions from that corpora that do start with antisemitic statements (which are proportionally less contextual than in France or Germany) do not trigger as much antisemitism as those in the German or French corpora. The reasons for this finding are still to be uncovered and could form the topic of future study.
6. Conclusion
In this chapter, I explored several hypotheses regarding the structure of online conversations on the social media platform Facebook in comment threads posted by web users on the official pages of mainstream news outlets. The analysis found that the structure of the conversation does not determine and, therefore, does not offer insight into the patterns of antisemitism online. Nevertheless, some structural parameters can be very useful in predicting antisemitic hate speech content in online debates.
More importantly, these findings point towards a new way of organising data to provide machine-learning models with more context for the evaluation of comments, with the goal of categorising them as antisemitic or not antisemitic with greater accuracy.
6.1 Potential applications: Providing context to the evaluation of single comments
The study presented in this chapter did not provide evidence that some types of structures in an online conversation are more likely to contain antisemitic comments. It cannot be used to inform or streamline moderation guidelines and processes, as we have seen that the activity on one particular tree does not mean that the proportion of antisemitic comments is likely to increase. All comments, therefore, must be evaluated however different their structure (whether they appear singly or in trees).
The results regarding the initial comment on the tree are particularly interesting from the point of view of online content moderation, as one could imagine focusing initially on comments replying directly to the post (first level), then moving on to the discussions triggered by the comments categorised as antisemitic. However, while discussions starting with antisemitic comments should be given priority, discussion starting with a non-antisemitic statements should not be ignored as they still contain many antisemitic comments. This way of processing could be helpful for assisting in the identification and prioritisation of discussions in need of moderation.
This way of processing is also very beneficial because the context needed to understand the meaning of the comments might differ depending on the level of the comment. The introduction presented the two types of context needed to understand the meaning of a comment. The discourse event context (the abovementioned “who/qui” example) is found in the article or initial media post. For the initial categorisation focusing on the first level comments, context can only be the media article posts or current events, since at this level web users do not refer (yet) to each other. To understand the replies to the initial (trunk) comment, however, further context is required from surrounding (branch) comments. The context for the deeper levels can then be understood as the entirety of the tree in which the comment is placed. In other words, a second categorisation of comments can then take place at the tree level.
Machine-learning models require context for a better categorisation of small pieces of text (see Chapter 8). Distinguishing between the initial comments that reply directly to a post or article and replies to this initial comment provides context to aid in categorising comments for antisemitic ideation.
References
Becker, Matthias J., Daniel Allington, Laura Ascone, Matthew Bolton, Alexis Chapelan, Jan Krasni, Karolina Placzynta, Marcus Scheiber, Hagen Troschke and Chloé Vincent, 2021. Decoding Antisemitism: An AI-driven Study on Hate Speech and Imagery Online. Discourse Report 2. Technische Universität Berlin. Centre for Research on Antisemitism, https://doi.org/10.14279/depositonce-15310
Becker, Matthias J., and Hagen Troschke, 2023. “Decoding implicit hate speech: The example of antisemitism”. In: Christian Strippel, Sünje Paasch-Colberg, Martin Emmer and Joachim Trebbe (eds). Challenges and Perspectives of Hate Speech Research. Digital Communication Research, 335-352, https://doi.org/10.48541/dcr.v12.0
Jikeli, Günther, Damir Cavar and Daniel Miehling, 2019. Annotating Antisemitic Online Content. Towards an applicable definition of antisemitism, https://arxiv.org/pdf/1910.01214, https://doi.org/10.5967/3r3m-na89
Jikeli, Günther, Damir Cavar, Weejeong Jeong, Daniel Miehling, Pauravi Wagh, Denizhan Pak, 2022a. “Toward an AI Definition of Antisemitism?” In: Monika Hübscher and Sabine von Mering (eds). Antisemitism on Social Media. Abingdon: Routledge, 193–212, https://doi.org/10.4324/9781003200499
Jikeli, Günther, David Axelrod, Rhonda K. Fischer, Elham Forouzesh, Weejeong Jeong, Daniel Miehling and Katharina Soemer, 2022b. “Differences between antisemitic and non-antisemitic English language tweets”. Computational and Mathematical Organization Theory, 1-35, https://doi.org/10.1007/s10588-022-09363-2
Liphshiz, Cnaan, 27 February 2021. “Nearly 200 scholars back UK lecturer who called Jewish students Israel ‘pawns’” The Times of Israel, https://www.timesofisrael.com/nearly-200-scholars-back-uk-lecturer-who-called-jewish-students-israel-pawns/
Steffen, Elisabeth, Milena Pustet and Helena Mihaljević. “Algorithms against antisemitism? Towards the automated detection of antisemitic content online”. In this volume.
Wistrich, Robert, 1992. Antisemitism: The Longest Hatred. New York: Pantheon